Top Data Analytics Software to Enhance Your Insights
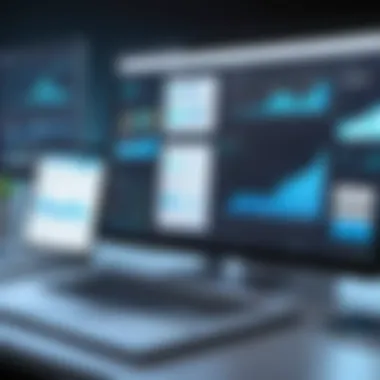
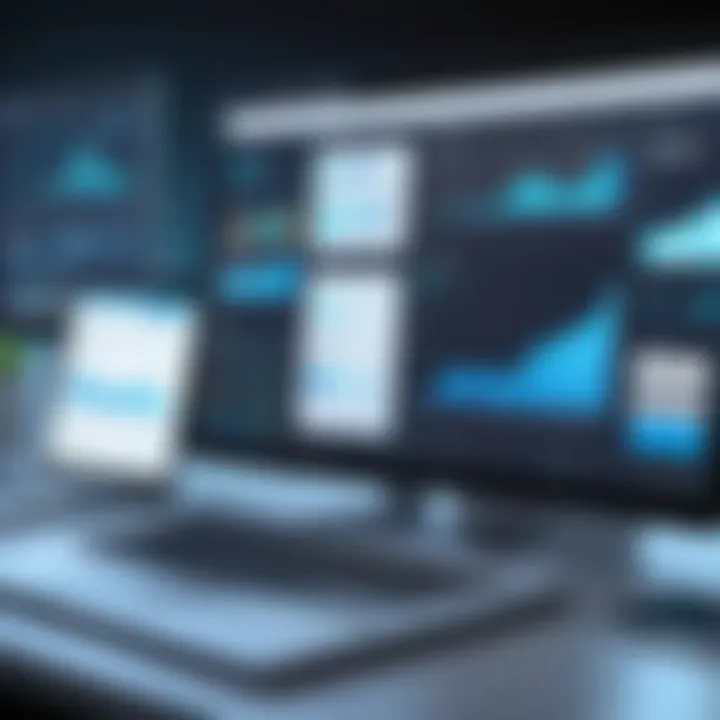
Intro
In the age of big data, everyone is looking to make sense of all the numbers swirling around. Whether it’s businesses aiming to increase their profits or students eager to hone their analytical skills, data analytics software step into the fray as vital tools. But with numerous options flooding the market, how does one sift through the myriad of choices to find the perfect fit? This guide aims to illuminate the diverse landscape of data analytics tools, exploring their functionalities and unique strengths.
As we dive deeper, the focus will not just be on the heavyweights like Tableau or SAS, but also emerging tools that are slowly carving out a niche. Understanding this software’s purpose, capabilities, and ideal scenarios of usage allows anyone, from seasoned analysts to budding data enthusiasts, to navigate the selection process with confidence. Equipped with this knowledge, users can elevate their data-driven decision-making processes, tailor solutions to specific needs, and ultimately drive better outcomes.
Software Overview
Purpose and Function of the Software
Data analytics software serve to transform raw data into meaningful insights. They help organizations make sense of complex datasets by facilitating data visualization, statistical analysis, and predictive modeling. The ultimate goal is to extract actionable intelligence that can guide decision-making.
Here’s a breakdown of the core purposes these tools fulfill:
- Data cleaning and preparation to ensure accuracy
- Implementing analyses to uncover patterns or trends
- Visualizing data for clear, intuitive presentations
- Automating reports for consistent, real-time insights
Most importantly, they cater to a wide array of industries and use cases—from finance analytics to healthcare informatics, enabling stakeholders to drive performance effectively.
Key Features and Benefits
When it comes to features, varied data analytics software might resemble each other on the surface, but digging deeper reveals distinct attributes and advantages. Here are some key features common across many platforms:
- Interactive Dashboards: Presenting data dynamically for easy comprehension.
- Collaboration Tools: Allowing teams to work together seamlessly on projects.
- Machine Learning Capabilities: Facilitating predictive analytics to forecast future trends based on historical data.
- Seamless Integration: Supporting connections with various data sources, which is essential in today’s data-hungry environment.
The benefits range from improving productivity to enhancing accuracy in analyses. Ultimately, businesses that harness the right software witness enhanced operational efficiency and informed strategic decisions.
"Data is not just a collection of facts; it’s a key to unlocking new opportunities."
Installation and Setup
System Requirements
Before jumping into the installation phase, it's crucial to be aware of the prerequisites for optimal performance. Most data analytics tools specify certain hardware and software requirements, which might include:
- Operating System: Windows, macOS, or Linux support depending on the tool
- RAM: Generally, 8GB minimum is advisable, though large datasets may require more.
- Processor: A multi-core processor can enhance processing speed
- Storage: Adequate disk space to accommodate datasets and applications
Installation Process
Setting up data analytics software typically involves these steps:
- Download the Installer: Obtain it from the official website to ensure it’s safe and up-to-date.
- Run the Installer: Follow prompts carefully, ensuring all components are selected.
- Configure Settings: Depending on your workflow, you might want to customize initial settings, such as data source connections or preferences.
- Activate License: If it’s a paid software, you’ll need to input your license key to unlock full features.
- Test Run: Finally, run a sample analysis to confirm everything works smoothly.
With this guidance, you’re now equipped to make insightful decisions about data analytics software. Don’t forget to keep exploring and experimenting with new tools that might just revolutionize your approach to data.
Prologue to Data Analytics Software
In today’s fast-paced world, data isn't just fluff. It's the engine driving decisions across industries. Organizations, big and small, are navigating the vast ocean of data to harness valuable insights. This tide of information amplifies the need for robust data analytics software—tools designed to sift through data and unveil patterns.
The significance of understanding data analytics software is multifaceted. First, it arms professionals with the ability to make informed decisions, ensuring that their strategies are data-driven rather than based on gut feelings. Without these tools, insights from potentially valuable data could slip through the cracks, leaving organizations at a disadvantage. This article serves as a roadmap to understanding the landscape of data analytics software, providing clarity amidst the technical jargon and complexity.
Defining Data Analytics Software
Data analytics software refers to tools and platforms used to analyze raw data with the aim of drawing meaningful insights. These tools enable users to prepare, process, and visualize data, turning it from mere numbers on a screen into actionable intelligence. Examples include Tableau and Microsoft Power BI, which allow users to create visual representations of their data, and statistical analysis tools like SAS that help tackle deeper inquiries. It's through these software solutions that data transforms from a chaotic mess into structured, useful knowledge.
In very simple terms, if data is the fuel, analytics software is the engine that makes it go.
Importance of Data Analytics in Today’s Landscape
In the current market scenario, the question isn't if data is important—it's how organizations leverage it to stay ahead. Data analytics has become pivotal across sectors, ranging from healthcare to finance. Here are some reasons why data analytics holds a critical place in today's business practices:
- Informed Decision-Making: Rather than shooting in the dark, companies can base their decisions on solid evidence, minimizing risk.
- Identifying Trends: Data analytics helps businesses spot patterns, allowing for timely responses to market shifts. If a retail company notices a sudden spike in demand for a particular product, they can pivot their strategy accordingly.
- Customer Insights: Understanding customer behavior through data analytics illuminates preferences and improves service. This level of insight can effectively enhance customer experiences and retention rates.
- Operational Efficiency: By analyzing processes, companies can identify bottlenecks and streamline operations, ultimately cutting costs.
"In an age where data is generated faster than light, the ability to analyze it in real-time can be the difference between leading the pack and getting left behind."
As we delve deeper into the realm of data analytics software, it becomes evident that these tools are not just luxuries; they are necessities in surviving and thriving in the modern world. Organizations that recognize and adapt to the capabilities of data analytics are often the ones who emerge as leaders in their fields.
Key Features of Data Analytics Software
Data analytics software is a pivotal component for organizations intending to transform raw data into actionable insights. These tools not only empower users by simplifying complex data manipulations but also enhance decision-making processes. When individuals or companies explore various software options, understanding the features that underpin their functionality becomes essential. Key features not only differentiate one software from another but also dictate their performance and relevance to specific business needs.
Data Integration Capabilities
Having robust data integration capabilities is a hallmark of effective analytics software. The ability to connect with diverse sources of data—be it databases, spreadsheets, cloud storage, or even APIs—ensures that users can consolidate information seamlessly.
For instance, imagine a marketing team gathering data from social media channels, CRM systems, and website analytics. Without easy integration, compiling reports could become a cumbersome task, fraught with duplications or missing insights. Here are some points to consider when evaluating integration features:
- Compatibility: Ensure the software can connect to existing systems and platforms.
- Real-time Data Access: Check if it provides live access to data feeds, improving timely insights.
- ETL Processes: Evaluate Extract, Transform, Load capabilities that allow for cleansing and structuring of data before analysis.
Visualization Tools and Techniques
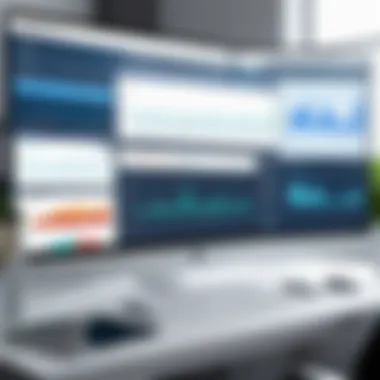
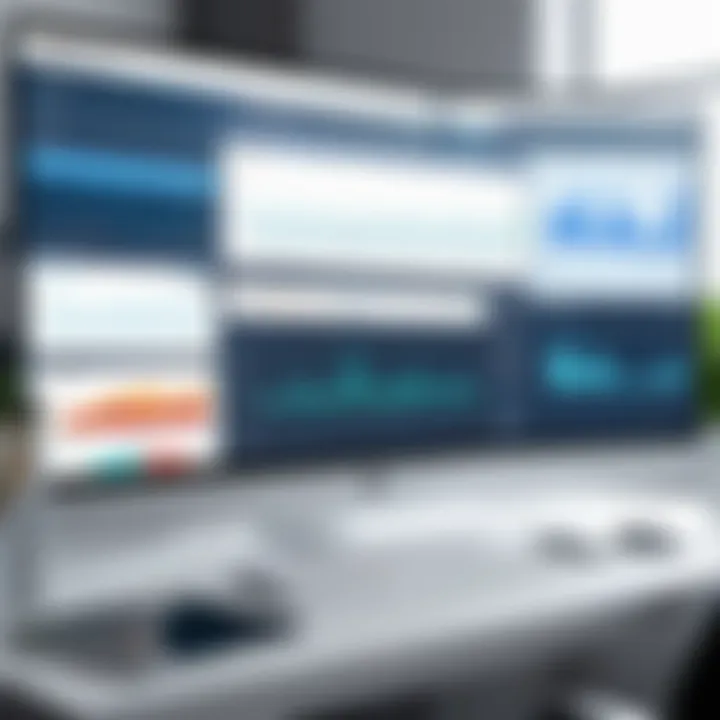
Visualization stands at the forefront of making data comprehensible. No one wants to wade through endless rows of numbers. A good software platform provides a variety of visualization tools and techniques. These allow users to depict data in engaging formats—charts, graphs, and maps—making complex data sets easier to digest.
Visualization is about more than mere aesthetics; it's about understanding trends and anomaly detection. A striking example is the use of heatmaps for demographic data analysis. They enable marketers to quickly spot areas of interest, helping to tailor campaigns effectively.
"A picture is worth a thousand words"—this rings especially true when it comes to data analysis.
- Customization: Look for tools that allow users to create custom dashboards tailored to specific needs.
- Interactivity: Dynamic visualizations encourage exploration, enabling users to ask questions and uncover hidden patterns.
Advanced Analytical Functions
Advanced analytical functions are the cherry on top for those seeking deeper insights beyond surface-level analysis. Sophisticated analytical capabilities, such as predictive analytics, data mining, and what-if scenarios, enable users to foresee potential outcomes based on historical data trends.
For example, consider retail companies forecasting their inventory needs to meet customer demand while avoiding overstock. Utilizing predictive functions can significantly reduce waste and optimize resource allocation. It’s like having a crystal ball—but with data as your oracle.
Some essential features to consider include:
- Machine Learning Algorithms: Allow for automatic learning and adaptation from new data.
- Statistical Functions: Essential for professionals conducting hypothesis testing or regression analysis.
User Interface and Experience
No matter how powerful the tool, if users find it difficult to navigate, the effectiveness diminishes. A strong user interface and experience (UI/UX) are crucial for maximizing user engagement and efficiency. The software should present a clean layout and intuitive workflows, allowing users—from data scientists to business analysts—to harness the tool’s full potential without unnecessary hurdles.
Good UI/UX doesn't just help in ongoing analysis but can also facilitate onboarding for new users.
Consider these aspects:
- Ease of Use: Ensure that tools support quick access to functions, dramatically reducing the time spent learning.
- Help and Documentation: Good software comes with ample support resources, from tutorials to community forums.
In summary, understanding these key features equips users to choose data analytics software that aligns with their needs while reaping the full benefits of data exploration and analysis.
Types of Data Analytics Software
When it comes to navigating the vast landscape of data analytics, understanding the various types of data analytics software is essential. Each type serves distinct purposes and caters to different analytical needs, from simple reporting to complex predictive modeling. Selecting the right kind for your specific context can drastically improve the effectiveness of data insights and decision-making processes. To help demystify this subject, we delve into four primary categories: Business Intelligence Tools, Statistical Analysis Software, Predictive Analytics Platforms, and Big Data Analytics Tools.
Business Intelligence Tools
Business Intelligence (BI) tools focus on providing actionable insights through data analysis and reporting. They act like a compass for organizations, guiding them through massive data flows to identify trends and patterns that can influence strategic decisions. Tools like Tableau and Microsoft Power BI exemplify this category, offering intuitive dashboards where complex data can be visualized with ease.
These tools aggreggate data from various sources, can help teams understand business performance, track key performance indicators (KPIs), and generate reports that resonate with decision-makers. The beauty of these tools is that they bridge the gap between technical data analysis and everyday business understanding, which makes them invaluable in today’s data-driven world.
Statistical Analysis Software
Statistical Analysis Software (SAS) plays a critical role for those who need to drill down into data’s underlying structure. These tools allow users to perform advanced statistical tests, regressions, and data mining processes. Software like R and SPSS falls under this category, arming professionals in fields like healthcare, finance, and academia to dissect complex datasets and extract meaningful narratives.
The strength of these applications lies in their capacity to handle extensive datasets with precision. Users often employ this software to identify correlations, make predictions based on historical data, or validate hypotheses. For those looking to conduct serious empirical research or analyze experimental data, statistical analysis software is often non-negotiable.
Predictive Analytics Platforms
Predictive Analytics Platforms are designed to forecast future outcomes based on historical data. Think of this category as the crystal ball of data analytics, providing a forward-looking perspective. Tools like SAS Predictive Analytics and IBM Watson utilize sophisticated algorithms and machine learning techniques to propel businesses into the future.
By employing predictive analytics, organizations can anticipate customer behaviors, manage risks, and optimize marketing strategies. This type of software is particularly valuable in industries such as retail or finance, where understanding potential market trends can significantly enhance stock management or customer engagement strategies.
Big Data Analytics Tools
In today’s world, where data grows at an exponential rate, Big Data Analytics Tools have emerged to address the unique challenges associated with massive datasets. Technologies like Apache Hadoop and Spark enable organizations to store, process, and analyze vast amounts of data quickly and efficiently. They function as the backbone for big data operations across numerous sectors, from telecoms to social media.
Big Data analytics tools often employ distributed computing models that allow for real-time data processing. The insights derived can be used for everything from improving product recommendations to optimizing supply chain logistics. Moreover, as companies collect more data than ever before, being harnessing insights from this data becomes increasingly pivotal for staying competitive.
"Choosing the right type of data analytics software can make the difference between mere data collection and transformative insights."
By recognizing these distinct types of software, readers can better assess their own analytics needs, ensuring they pick tools that align with their analytical goals and business requirements. As we progress through this guide, we will explore leading data analytics solutions and their specific applications in further detail.
Leading Data Analytics Software Solutions
Choosing the right data analytics software is crucial for any organization that aims to leverage the growing volumes of data they encounter daily. Not only do these tools streamline the process of data analysis, but they also enhance decision-making through clear insights and actionable intelligence. Knowing what solutions stand out in this crowded market is essential for professionals looking to optimize their analytics strategies.
In this section, we’ll take a closer look at some leading data analytics software solutions, exploring their key strengths, benefits, and practical applications that make them invaluable for businesses and organizations of all sizes. Each software solution has distinct characteristics designed to meet various analytical needs.
Tableau: Key Strengths and Use Cases
Tableau is often hailed as a powerhouse in the realm of data visualization. Its user-friendly interface allows users to create complex visualizations without needing to write a single line of code. This visual emphasis makes it easier to interpret data patterns and trends, enabling informed decision-making.
- Data Connectivity: Tableau supports numerous data sources like SQL databases, Excel files, and cloud data, ensuring ease of use.
- Interactivity: Users can manipulate dashboards in real-time, providing a dynamic experience that allows for immediate insights.
- Collaboration: Tableau’s sharing features let teams collaborate seamlessly on insights and reports, making it an ideal choice in a team-centric environment.
Tableau is particularly suitable for sectors like healthcare, finance, and marketing, where data comes in multiple forms and needs to be visualized for varied stakeholders.
Microsoft Power BI: Overview and Benefits
Microsoft Power BI has rapidly become a favorite among businesses seeking robust visual analytics. Its integration with the Microsoft suite means it fits snugly into existing workflows, making it a go-to option for organizations already using tools like Excel.
- Built-In AI Features: Power BI incorporates AI capabilities, making trend analysis even more straightforward.
- Cost-Effectiveness: The software offers a range of pricing tiers, making it accessible for small businesses as well as large enterprises.
- Customizable Reports: Users can create highly customized reports that can be tailored to specific business needs.
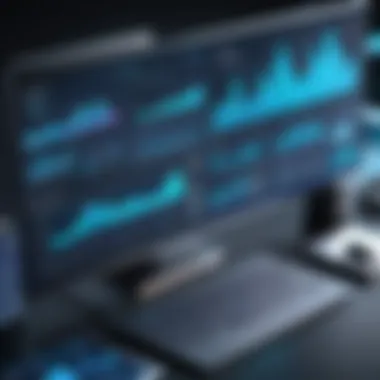
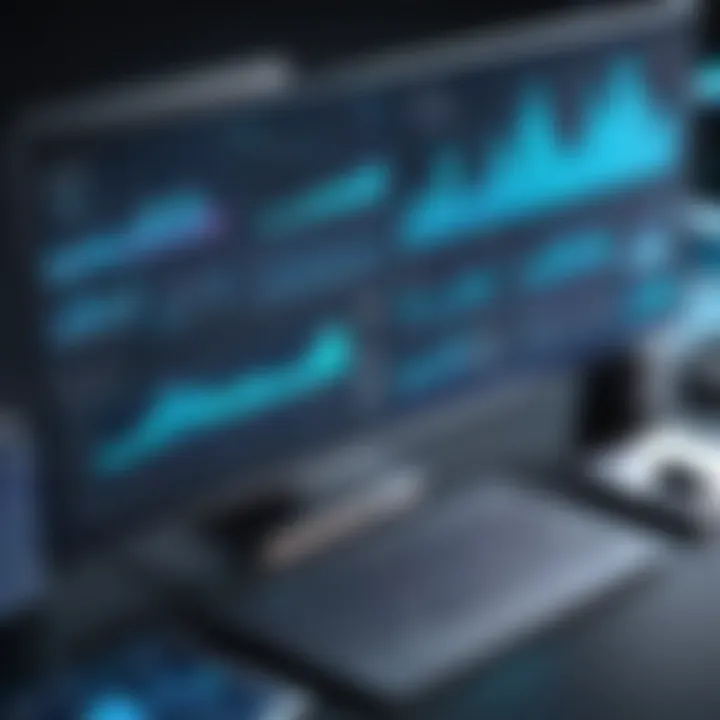
With its blend of powerful analytics and intuitive design, Power BI is well-suited for organizations of various industries, from retail to manufacturing.
SAS: Features and Applications
SAS is a long-standing name in data analytics, providing advanced statistical analysis and predictive analytics capabilities. The software is notably robust when it comes to analyzing complex datasets for future trends.
- Advanced Analytics: SAS excels in statistical modeling, making it a solid choice where predictive analytics are essential.
- Industry Applications: It is notably popular in sectors requiring intensive data analysis, like pharmaceuticals, where it supports clinical trials and regulatory compliance.
- Security and Governance: SAS provides strong security measures, which is vital for industries handling sensitive data.
Given its depth, SAS is appropriate for data-heavy environments demanding precision and customization.
Google Analytics: Usage Scenarios
Google Analytics is synonymous with web analytics. It provides comprehensive insights into website performance, user behavior, and conversion metrics.
- Ease of Access: The free version serves as an entry point for many small businesses, making it a great starting platform for budding analysts.
- Real-Time Data: Users can access real-time data, making it easier to adjust strategies on the fly.
- Integration with Google Ads: It seamlessly connects analytics and advertising data for a holistic view of marketing efforts.
Primarily, Google Analytics is beneficial for digital marketers and web developers tracking their online performance.
R and Python for Data Analysis
Both R and Python have emerged as go-to programming languages for data analysis. Their open-source nature allows users to wield significant power without hefty licensing fees.
- Flexibility: R is often chosen for its statistical capabilities, while Python’s versatility is unmatched, being useful for various tasks that extend beyond just analytics.
- Large Communities: Both come equipped with libraries and extensive community support, easing the development process.
- Visualization Tools: With packages like ggplot2 in R and Matplotlib in Python, users can generate compelling visual representations of data.
These tools cater to data scientists and analysts who require flexibility and power in handling complex datasets.
In the evolving landscape of data analytics, knowing which software to utilize can make the difference between strategic success and missed opportunities. Each tool, with its unique strengths, plays a pivotal role in driving data-centric decisions.
Emerging Trends in Data Analytics Software
Understanding the emerging trends in data analytics software is crucial for staying competitive in this fast-paced digital world. These trends not only influence the way data is analyzed but also shape strategic decisions across various industries. Adopting the latest tools and methodologies not only drives efficiency but also enhances decision-making capabilities. By embracing new technologies, organizations can harness the full potential of their data while addressing contemporary challenges like data privacy and integration hurdles.
Machine Learning Integration
One of the most significant trends in data analytics software is the integration of machine learning. This technology enables systems to learn from data and make predictions without being explicitly programmed. Businesses can analyze historical data faster and more accurately than traditional methods allow.
- Benefits of Machine Learning:
- Enhanced Prediction Accuracy: Through training on large datasets, machine learning models can provide insights that help predict future trends.
- Automation of Routine Tasks: By automating mundane data analysis tasks, professionals can focus on strategic planning.
- Personalized User Experiences: Machine learning algorithms can tailor recommendations based on user behavior, enhancing customer satisfaction.
As businesses continue to capitalize on this capability, pursuit of innovative solutions using machine learning is no longer optional but essential.
Real-Time Analytics Capabilities
In an age where instantaneity defines success, the demand for real-time analytics capabilities has skyrocketed. Companies are increasingly interested in tools that can process and analyze data as it arrives. This immediate insight allows organizations to react swiftly to emerging trends or operational hiccups.
- Why Real-Time Analytics Matters:
- Timely Decision-Making: Access to current data supports prompt actions in a dynamic market.
- Improved Customer Interaction: Companies can respond to customer queries or issues immediately, enhancing the overall experience.
- Operational Efficiency: Quick access to live data allows businesses to optimize operations, leading to cost savings.
Adopting real-time analytics is a game changer in today’s competitive landscape, ensuring organizations stay ahead of the curve.
Cloud-Based Solutions
Cloud computing continues to transform how data analytics solutions are developed and deployed. With its scalability and flexibility, cloud-based software solutions have emerged at the forefront of data analytics. This trend is increasingly appealing to organizations of all sizes struggling with limited resources.
- Advantages of Cloud-Based Solutions:
- Cost-Effectiveness: Companies can reduce their IT expenditure by leveraging cloud infrastructure rather than building on-premises solutions.
- Ease of Collaboration: With cloud platforms, teams can access data from anywhere in the world, promoting teamwork and productivity.
- Enhanced Security: Leading cloud providers implement stringent security measures to protect sensitive data, addressing major concerns around data privacy.
Cloud-based applications are not merely a trend; they are redefining how businesses approach data analytics, enabling them to adapt quickly in an ever-evolving digital space.
The integration of machine learning, real-time analytics, and cloud-based solutions represents a significant shift in data analytics software, making it imperative for organizations to stay informed and agile.
Selecting the Right Data Analytics Software
Choosing the right data analytics software is like picking the right tool from a toolbox; the effectiveness of the analysis hinges on the tool's capability to meet specific business needs. In today’s fast-paced environment, businesses lean heavily on data to drive decision-making, optimize processes, and uncover trends. Selecting suitable software can pave the way for these objectives, empowering professionals to transform raw data into actionable insights.
When considering potential data analytics solutions, it’s essential to focus on several key elements that go beyond just basic features. Every organization has unique requirements; hence, taking a tailored approach is vital. The landscape of data analytics is vast yet complex; navigating through this can be quite daunting if one doesn't have the right criteria in mind.
With careful consideration and strategy, choosing the right software can enhance the analytical process, streamline workflows, and ultimately contribute to achieving the company’s goals.
Assessing Business Needs
Before diving headfirst into vendor comparisons, it's crucial to assess the specific needs of the business. Asking pointed questions can clarify a lot. For instance:
- What are the primary objectives for using data analytics?
- Is the focus on reporting, advanced analytics, or both?
- Who will be using this software, and what is their level of expertise?
Identifying the scope helps narrow down options considerably. The software should align with not only the current analytical demands but also future aspirations. For example, a small e-commerce business might just need basic reporting capabilities when starting, while a multinational corporation might require advanced predictive analytics to inform strategic decisions across various departments. Don’t be afraid to dig deep; a thorough assessment can save time and resources down the line.
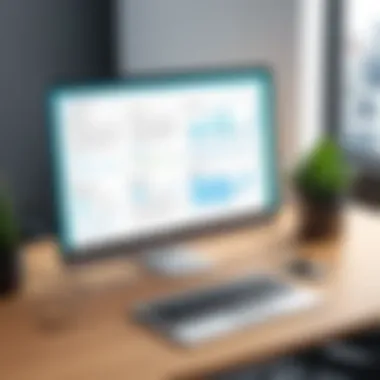
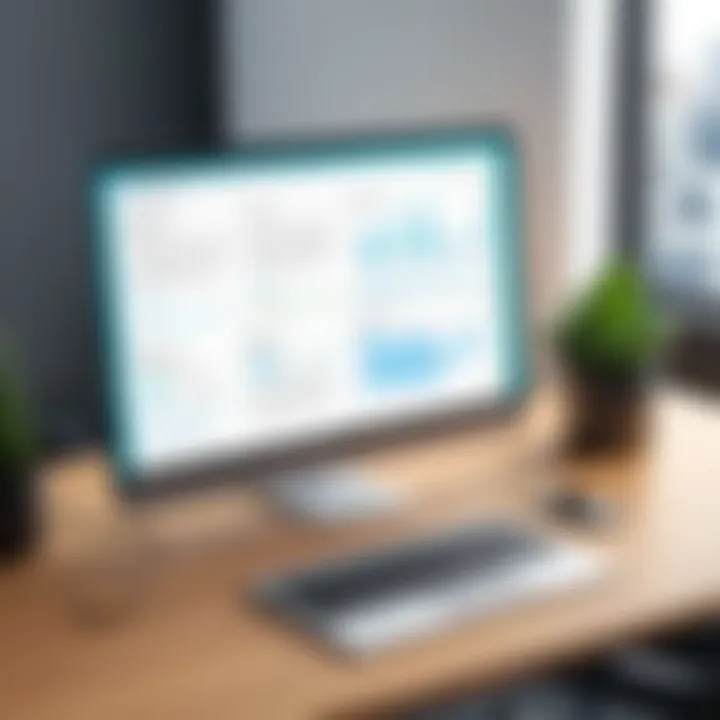
Budget Considerations
Budgeting is a hard but indispensable part of the decision-making process. Data analytics software can come at a pretty penny; costs can vary drastically depending on the complexity of the tool and the specific features offered. While it might be tempting to go for the most sophisticated option available, it is vital to analyze what fits within the budget constraints without compromising quality.
- Initial Costs: Licensing, implementation fees, and setup costs can stack up quickly.
- Ongoing Expenses: Training, support, and updates should be considered in total cost of ownership.
- Hidden Costs: Don’t overlook potential hidden costs associated with maintenance and integration.
Evaluating ROI is essential. An inexpensive tool might save money upfront but could lead to data quality issues or lack of necessary functionalities that complicate reporting and analysis in the long run. A mid-range solution with the right features might turn out to be a better investment overall.
Skill Level and Support Resources
Another layer that adds to the complexity of choosing the right software is the skill level of potential users. The software might have fantastic features, but they are moot if the staff can’t harness them effectively.
- Understanding User Expertise: Conducting a skills assessment can be insightful. A highly technical team might thrive with complicated software like R or Python, whereas less technical users might benefit more from user-friendly tools like Tableau or Power BI.
- Training and Support: Some vendors provide extensive training and support materials, while others may not. Analyze whether your team will need hands-on training, tutorials, or even a dedicated support team.
- Community and Resources: Availability of forums and online resources can be tremendously helpful, as troubleshooting can often lead to blind alleys without a helpful community.
To sum up, selecting the right data analytics software requires careful thought and analysis. Circling back to evaluate business needs, budgeting wisely, and ensuring that your team is equipped with the right skills and support resources can illuminate the path to a well-informed choice.
Ultimately, the 'best' software is the one that best aligns with your organizational goals, fits your budget, and empowers your team to leverage data effectively.
Challenges in Data Analytics Software Implementation
Implementing data analytics software comes with a fair share of challenges that can make or break the overall experience for businesses. Understanding these obstacles is crucial for organizations looking to harness the power of data. Not only do these challenges affect the choices made on software but they also impact the efficiency and utility of the entire data analytics process. Addressing these issues head-on can lead to more effective use of analytics tools and, consequently, more informed decision-making.
Data Quality Issues
Data quality is a cornerstone of any successful analytics initiative. It’s often said that “garbage in, garbage out,” and this saying couldn’t be more relevant. Poor data quality can stem from various sources such as human error, outdated information, or inconsistent data entry practices. When the foundation of your analysis is rocky, the results will often miss the mark.
To mitigate these issues, businesses should prioritize data cleansing and establish robust processes for data governance. Implementing validation rules during data entry, periodic audits, and employing data quality tools can significantly enhance the accuracy and reliability of datasets. For instance, consider an organization relying on customer feedback to guide product development. If the feedback collected is flawed or biased, the insights derived could lead to misguided strategies that may affect the overall business trajectory.
User Adoption Resistance
Even the best software can fall flat without user buy-in. Often, team members resist new tools out of fear of the unknown, disrupted routines, or lack of confidence in their ability to adapt to new systems. This resistance can lead to underutilization of the analytics software, defeating the purpose of its implementation.
A proactive approach can help ease the transition. This may include providing comprehensive training sessions that address user concerns and technological apprehensions. Additionally, involving employees in the selection process can create a sense of ownership and accountability, ultimately smoothing the path to adoption. Organizations can also highlight the software's benefits through case studies or pilot programs that illustrate tangible improvements.
Integration with Existing Systems
Integrating new data analytics software with legacy systems can often feel like trying to fit a square peg in a round hole. The challenges of interoperability can lead to costly delays and frustration. Issues might arise from data migration complexities, compatibility with existing software, or differences in data formats.
Before making any decisions, it is wise to conduct a thorough assessment of the current technology stack. This evaluation should ideally precede the software selection process, ensuring that the new solution can effectively integrate with foundational systems. Building a phased implementation plan can also be advantageous, allowing for gradual integration and troubleshooting that minimizes operational disruptions.
"Understanding the challenges in data analytics software implementation is key to ensuring that businesses leverage data effectively for strategic advantages."
Future Outlook for Data Analytics Software
The future of data analytics software is an exciting and crucial aspect in the realm of technology and business intelligence. As we progress, the landscape will witness significant transformations that can redefine how organizations operate, analyze, and leverage data. Keeping an eye on this outlook is essential for businesses aiming to maintain a competitive edge.
One dynamic feature is the sustained growth in AI integration within analytics platforms. These algorithms can sift through massive data pools faster and more accurately than traditional methods. The enhanced capabilities will allow businesses to uncover patterns and insights that previously might have gone unnoticed. For software developers and IT professionals, this means new opportunities to build smarter systems that can handle complex queries without breaking a sweat.
Moreover, the push toward cloud-based solutions presents companies with flexible options for scalability and data accessibility. No longer tethered to hefty hardware, organizations can pivot their analytical capabilities based on demand. This means less expenditure on infrastructure and more agility in decision-making.
Lastly, with every advantage comes the weight of data privacy concerns, particularly as regulations grow stricter across the globe. Addressing these challenges will be paramount when developing future analytics software. Unlocking the potential of data analytics demands a comprehensive strategy that puts user protection at the forefront. In this segment, we will delve deeper into critical elements—specifically, the impact of artificial intelligence and the rising importance of data privacy.
Impact of Artificial Intelligence
Artificial Intelligence (AI) is set to become the backbone of data analytics software. With the ability to handle vast amounts of data efficiently, AI tools can analyze patterns, automate repetitive tasks, and even assist in predictive modeling. The incorporation of machine learning algorithms creates a feedback loop that continuously improves the accuracy of data predictions over time.
This leads to informed decision-making, whether in marketing strategies, resource allocation, or customer relationship management. For instance, AI-driven analytics tools can segment customers based on behavior, helping businesses tailor their strategies to meet specific needs. This level of precision can dramatically enhance customer satisfaction and drive revenue.
The importance of AI is not just in analytics but in usability as well. Advanced natural language processing allows users to interact with software simply by asking questions, making analytics accessible to non-technical staff. Thus, the adoption of AI not only enriches the analytical capability but also democratizes access to insights among various levels of an organization.
"AI will not replace humans but augment their capabilities, redefining what is possible in data analytics."
Rising Importance of Data Privacy
As data analytics software advances, the issue of data privacy cannot be overlooked. With regulations stiffening globally, companies must find ways to integrate robust privacy protocols without stifling analytical capabilities. The need for transparency is becoming non-negotiable, with customers increasingly concerned about how their data is being used.
Organizations now face the challenge of ensuring compliance with regulations like GDPR in Europe and CCPA in California. This necessitates the implementation of comprehensive privacy frameworks and technology that safeguard consumer data while still allowing for valuable insights.
Adopting privacy-centric analytics not only builds trust but can also enhance a brand’s reputation. By actively promoting data protection, businesses can distinguish themselves in an overcrowded market. Therefore, a careful balance between powerful data insights and rigorous data protection practices is essential for any organization looking to succeed in the analytics domain.
Finale
In the ever-evolving landscape of data analytics, understanding the nuances of choosing the right software is crucial for professionals, students, and industry aficionados alike. This conclusion serves as a reflective end that ties together the various elements discussed throughout the article. The role of data analytics software in making sense of vast amounts of data can't be overstated. With the right tools in hand, businesses can transform mountains of raw data into actionable insights, driving strategic decisions and fostering innovation.
Summarizing Key Takeaways
As we summarize the key takeaways from this guide, there are several pivotal points to consider:
- Diversity of Tools: There is no one-size-fits-all solution. From Tableau’s powerful visualization capabilities to the robust statistical functions of R, the software landscape offers a multitude of choices depending on specific needs.
- Integration Importance: The ability to integrate different data sources is often a deciding factor when selecting analytics software. A seamless integration can enhance the flow of data, thereby improving analytical outcomes.
- User Experience: The learning curve of any software should be taken into account. User-friendly interfaces often lead to faster adoption and better results, especially in teams with varied levels of technical expertise.
- Continuous Improvement: The analytics field is not static—emerging trends like machine learning and real-time analytics are shaping the future. Staying informed on these innovations is vital for sustained competitive advantage.
"Data is the new oil, but like oil, it needs refining." This statement encapsulates the necessity for proficient tools that can process and analyze data effectively.
Encouraging Continuous Exploration in Data Analytics
With the pace of innovation in data analytics, it's imperative to foster a culture of continuous exploration. Software developers, IT professionals, and students should remain curious and proactive in seeking new knowledge and tools. Here are some recommendations:
- Stay Updated: Regularly engage with current articles, workshops, and training sessions on the latest data analytics software. Websites like Wikipedia and Reddit provide a wealth of community knowledge and updates.
- Experiment: Hands-on experimentation with various tools can yield insights that complement theoretical learning. Free trials offer a risk-free way to explore different capabilities before committing to a purchase.
- Networking: Join online forums or communities where discussions about data analytics software take place. Networking with peers can lead to new ideas and best practices that enrich your understanding.
- Long-term Vision: As the technologies evolve, so should your skill set. Regularly revisit your knowledge and adapt to new methodologies and tools as they emerge.