A Comprehensive Guide to Clinical Research Data Management
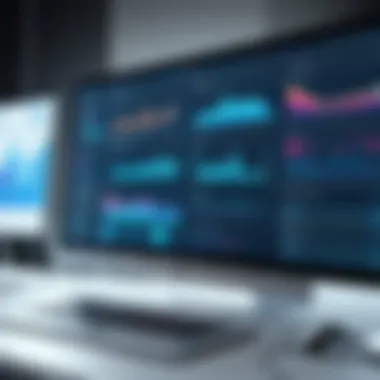
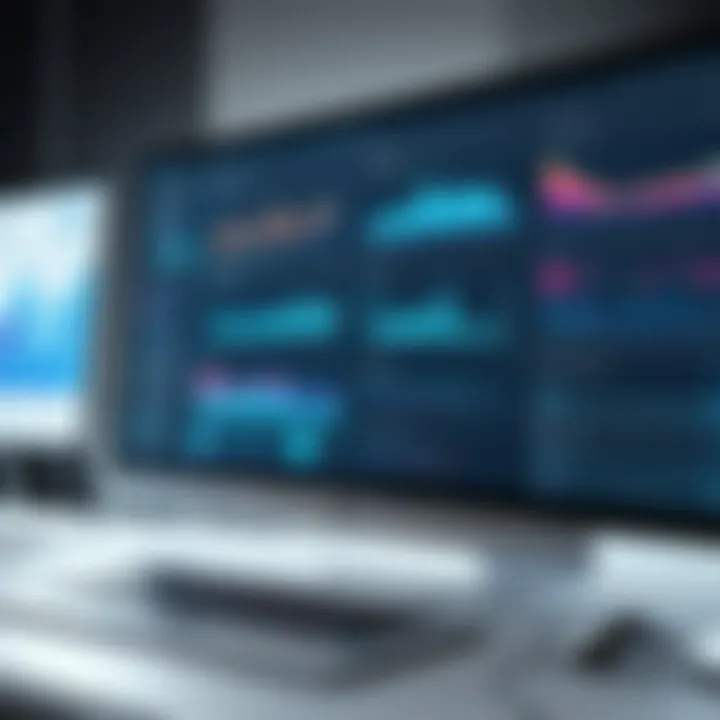
Intro
Clinical research is a vital aspect of advancing medical knowledge. Efficient management of data generated during clinical trials is paramount. This is where clinical research data management software comes into play. It plays a crucial role in ensuring that data collection, storage, and analysis are carried out with integrity and accuracy. Such software is specifically designed for the challenges posed by clinical research.
In the following sections, we will explore the purpose and function of clinical research data management software. We will also identify its key features and benefits, discuss installation and setup requirements, and highlight the significance of these tools in the clinical research landscape. Understanding these make it easier for professionals to select the best software for their needs and improve research outcomes.
Prologue to Clinical Research Data Management Software
Clinical research data management software plays a crucial role in streamlining the collection, storage, and analysis of data derived from clinical trials. The complexity and volume of data generated in the healthcare sector make it imperative for researchers to utilize robust data management solutions. These platforms not only enhance the efficiency of clinical research but also improve the accuracy and integrity of the data.
One of the key benefits of clinical research data management software is its ability to centralize data. In clinical trials, data comes from multiple sources such as clinical sites, laboratories, and patient records. A suitable data management solution aggregates and organizes this information in a unified interface, reducing the chances of errors and discrepancies.
Moreover, such software is equipped with functionality for data validation. It ensures that the data collected meets predefined standards and is consistent. This is essential for maintaining compliance with regulatory bodies like the FDA and for upholding the overall quality of the research output.
Clinical research data management software often incorporates advanced analytical tools. These tools allow researchers to generate insights from the data, aiding in decision-making processes. The reporting capabilities within these systems facilitate communication of findings among stakeholders, which can include sponsors, regulatory agencies, and medical professionals.
Furthermore, utilizing this type of software aids in maintaining the confidentiality of sensitive patient information. Many of these platforms are designed to comply with regulations such as HIPAA, ensuring that data security is a top priority.
In summary, the introduction of clinical research data management software transforms the landscape of clinical trials. By facilitating efficient data handling and ensuring compliance, it allows researchers to focus more on the scientific aspects of their work rather than data management challenges. This sets the foundation for meaningful advancements in healthcare research.
Significance of Data Management in Clinical Research
Data management in clinical research is not just a technical necessity; it is integral to the overall success and integrity of research projects. As clinical trials generate massive amounts of data, effective management processes ensure that this data is accurate, secure, and accessible. Without a robust data management framework, the quality of research findings can be compromised.
Enhancing Data Accuracy and Integrity
One crucial aspect of data management is enhancing data accuracy. Clinical research often requires precise data collection methods. Misinformation or errors in data can lead to incorrect conclusions, posing significant risks to patient safety and trial validity. Well-structured data management practices include validation protocols that help in identifying discrepancies early in the process. This continuous scrutiny promotes confidence in the results presented.
Streamlined Access and Collaboration
In addition to accuracy, efficient data management streamlines access to information. Various teams, including researchers, validators, and monitors, must collaborate. An organized data management system ensures that all stakeholders can access real-time data when needed. This accessibility is critical in making informed clinical decisions quickly. Improperly managed data could lead to delays, inhibiting collaboration and ultimately slowing down the research process.
Regulatory Compliance and Ethical Standards
Another significant aspect relates to regulatory demands. The clinical research landscape is governed by strict standards and guidelines, such as those from the FDA in the United States. Non-compliance with these regulations can lead to legal penalties and damage to a research organization's credibility. A solid data management framework helps address these compliance requirements systematically. It ensures that data handling practices meet ethical standards, protecting both the data and the rights of participants involved in the research.
Long-term Data Repository and Future Research
Effective data management also has implications for future research. Proper documentation and data storage allow researchers to refer back to previous studies. This continuity in accessing research data can be essential for longitudinal studies or for those looking to build upon past findings. An established data management system ensures that valuable information is preserved for future generations of researchers.
"Effective data management is foundational to the integrity and success of clinical research. It supports collaboration, regulatory compliance, and the accuracy of results."
Ending
Fundamental Features of Data Management Software
In the realm of clinical research, data management software plays a vital role in ensuring the efficiency, accuracy, and integrity of research data. The fundamental features of these software solutions are crucial for researchers and organizations, as they directly impact data handling processes.
With the right data management features, clinical trials can yield significant insights while maintaining compliance with regulatory standards. The following sections explore three essential aspects of data management software: data collection capabilities, data validation processes, and reporting and analytics tools.
Data Collection Capabilities
Data collection forms the backbone of clinical research. It encompasses the methods and systems employed to gather information crucial to study aims. Effective data collection capabilities allow for seamless input of data from various sources. For instance, electronic case report forms (eCRFs) provide standardized templates that simplify data entry for researchers. This standardization minimizes variability and helps maintain consistency across studies.
Moreover, the integration with other systems, such as electronic health records (EHRs) or laboratory information systems (LIS), enhances data collection. Such interoperability reduces manual data entry, thus minimizing errors. In addition, flexible data collection methods, such as mobile data capture options, can further increase the amount of data collected and the quality of that data.
Data Validation Processes
Data validation processes are crucial in ensuring that the collected data is accurate and reliable. This is especially pertinent in clinical research where incorrect data can lead to flawed conclusions. Validation procedures often involve checks for consistency, completeness, and range. Automated validation routines flag discrepancies, enabling prompt resolution of issues.
In practice, this means that researchers can be confident in the data they are using. Furthermore, having a clear validation process can streamline data review for regulatory submissions, making the entire workflow efficient and compliant. Having such mechanisms in place enhances the credibility of the research findings.
Reporting and Analytics Tools
The ability to generate reports and perform analytics is a vital feature of data management software. Reporting tools allow stakeholders to visualize trends, track progress, and summarize findings effectively. This can enhance communication among teams and assist decision-making processes.
Analytics tools provide deeper insights into collected data. For instance, they can identify patterns that may not be immediately visible through standard reporting. These tools can also support predictive analytics, allowing researchers to anticipate outcomes based on existing data trends, ultimately aiding in refining clinical strategies.
"The integration of robust reporting and analytics tools not only simplifies data interpretation but also enriches the overall research process."
Challenges in Clinical Data Management
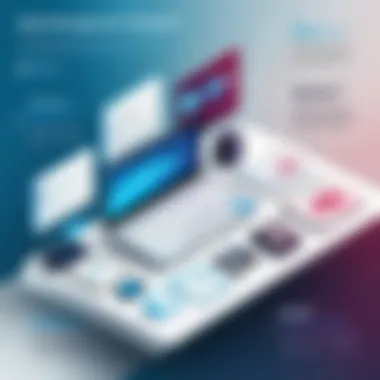
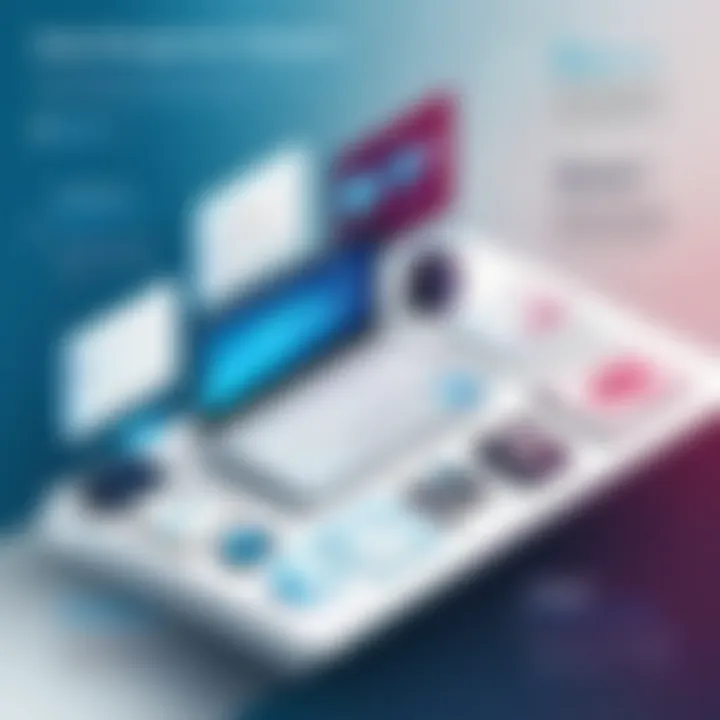
Clinical data management is a vital process in clinical research, yet it presents numerous challenges. Understanding these challenges can help organizations improve their data management strategies. The significance of addressing these hurdles is gleaned from their impact on research outcomes, compliance, and overall efficiency. Each challenge necessitates careful consideration to maintain the integrity and utility of clinical data.
Ensuring Data Integrity
Data integrity is paramount in clinical research. It refers to the accuracy and consistency of data throughout its lifecycle. With many stakeholders involved in data collection and processing, ensuring integrity becomes complex. Factors contributing to data integrity issues include human error, software bugs, and system malfunctions. Organizations must implement strict protocols to minimize these risks.
Adopting rigorous data validation methods is one approach. Automated checks can help detect anomalies and inconsistencies early in the data management process. Additionally, establishing clear procedures for data entry and review can further enhance data integrity. Regular audits can also serve as checkpoints to ensure compliance with prescribed standards. By prioritizing data integrity, organizations cultivate trust in their research findings.
Handling Large Datasets
The increasing volume of data in clinical research poses significant challenges. Large datasets can overwhelm traditional data management systems, leading to performance degradation and potential data loss. Handling these vast amounts of information requires specialized tools capable of efficient data processing and storage.
Implementing scalable cloud-based solutions can alleviate some of the issues associated with large datasets. These systems offer flexibility and resources that can adjust to the data volume. Moreover, data management software should include features that allow efficient querying, retrieval, and analysis of large datasets.
Training staff to use these tools effectively is equally important. An understanding of the system's capabilities can greatly enhance the efficiency of data handling. Continuous learning opportunities, such as workshops or online courses, can empower teams to manage large datasets more proficiently.
Integrating Diverse Data Sources
As clinical research evolves, so does the variety of data sources. Integrating data from electronic health records, laboratory systems, and patient-reported outcomes introduces new challenges. Each source may have its own format and requirements, creating friction in the data management process.
Developing a standardized framework for data integration can streamline this process. Organizations should explore strategies like using application programming interfaces (APIs) to connect disparate systems. APIs can facilitate seamless data flow between systems, reducing manual entry and the risks associated with it.
Moreover, adopting common data models can aid in harmonizing data from various sources. This approach ensures that data is structured uniformly, simplifying analysis and reporting. By effectively integrating diverse data sources, organizations can create a more comprehensive view of clinical research data, enhancing its value and applicability.
Regulatory Considerations in Data Management
The landscape of clinical research is heavily influenced by regulatory requirements. Regulatory considerations in data management are essential for ensuring that clinical trials maintain integrity, protect patient safety, and yield valid results. Variability in regulations across regions adds complexity to how data is managed. Understanding these regulations is critical for software developers and professionals working in IT in clinical settings. Compliance ensures not only the legitimacy of research outcomes but also that organizations avoid hefty penalties. Regulatory standards shape software design, influence data handling processes, and guide user training programs.
Compliance with FDA Regulations
The U.S. Food and Drug Administration (FDA) sets stringent guidelines for clinical research data management. Compliance with these regulations is of utmost importance for any research aiming to submit data to the FDA. The FDA's 21 CFR Part 11 outlines the requirements for electronic records and electronic signatures. This includes ensuring that data is protected from unauthorized access and that electronic signatures are unique and verifiable.
Additionally, maintaining good documentation practices is crucial. This includes audit trails, where modifications to data are logged and traceable. Organizations must establish protocols for data entry, verification, and validation that adhere to FDA standards. Failure to comply can lead to data rejection, delayed studies, and potential legal action. Thus, understanding and implementing FDA regulations is not just a necessity but an integral part of the software development process in clinical research.
HIPAA Compliance Issues
Health Insurance Portability and Accountability Act (HIPAA) compliance plays a vital role in clinical data management, especially when handling patient information. HIPAA sets the standard for protecting sensitive patient data. Research involving electronic health information must ensure that data is adequately safeguarded against breaches.
Organizations should implement administrative, physical, and technical safeguards to maintain HIPAA compliance. These safeguards include limiting access to sensitive information, encryption of data during transmission, and regular training for staff on privacy policies. Violations of HIPAA can result in severe penalties and undermine the public's trust in healthcare research, which is why prioritizing these compliance issues is crucial for any organization dealing in clinical data.
International Regulation Standards
As clinical research transcends borders, international regulation standards become increasingly relevant. Organizations must navigate a multitude of regulations like the European Union's General Data Protection Regulation (GDPR). GDPR emphasizes data protection and privacy. It requires explicit consent from participants before their data can be processed, along with the right for individuals to access and request deletion of their personal information.
Understanding and integrating these international regulations into data management software can be challenging. It is essential for developers to design adaptable systems that can comply with varying standards worldwide. This flexibility not only ensures regulatory adherence but also fosters trust among international collaborators and participants. Research data management must take these considerations into account to operate effectively on a global scale.
Market Overview of Clinical Research Data Management Software
Understanding the market landscape of clinical research data management software is essential for any stakeholder in the healthcare sector. This overview serves not only to highlight the current state of the software but also to emphasize the driving forces behind its growth and evolution.
As healthcare organizations increasingly recognize the value of efficient data management, the demand for software solutions has risen sharply. Clinical research data management software offers structured frameworks that streamline data collection, storage, and analysis. This enhances the quality of data derived from clinical trials and research, ensuring that findings are both reliable and reproducible.
One of the primary objectives of these software systems is to improve operational efficiency. By utilizing such tools, researchers can:
- Reduce data entry errors: Automated data collection methods minimize human errors associated with manual entries.
- Enhance collaboration: Integrated systems allow teams to access and share relevant data seamlessly across multiple departments or research sites.
- Comply with regulatory standards: Many software solutions are designed to assist organizations in adhering to essential regulations like FDA and HIPAA, ensuring that all data handling practices are in line with legal requirements.
Market trends indicate a clear shift towards cloud-based solutions. The adoption of cloud technology offers several advantages, including:
- Accessibility: Researchers can access data from anywhere, facilitating remote collaboration.
- Cost-effectiveness: Lower upfront costs and reduced need for on-premises hardware make cloud solutions financially appealing.
- Scalability: Organizations can easily scale operations up or down based on project demands.
Despite these advantages, several considerations must be acknowledged when evaluating the market.
"As organizations continue to adopt innovative solutions, it is vital to stay informed of emerging technologies and methodologies in data management to remain competitive."
As more players enter the field, understanding the competitive landscape becomes critical. Key factors to consider include:
- User Interface and Experience: A user-friendly design can significantly impact the effectiveness of the software. It is essential for developers to prioritize usability.
- Integration Capabilities: The ability to integrate with existing systems is crucial to streamline workflows and enhance productivity.
- Support and Training Services: Ongoing support and training are vital for ensuring that users can maximize the software's potential.
In summary, the market for clinical research data management software is dynamic and growing. Companies must be vigilant in choosing solutions that not only address current needs but also adapt to future advancements in technology and regulatory expectations. By recognizing the multiple elements influencing this market, stakeholders can make informed decisions that bolster the effectiveness and integrity of clinical research projects.
Comparative Analysis of Leading Software Solutions
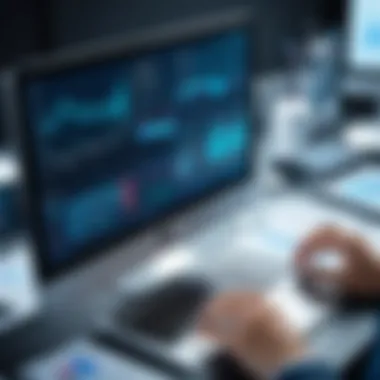
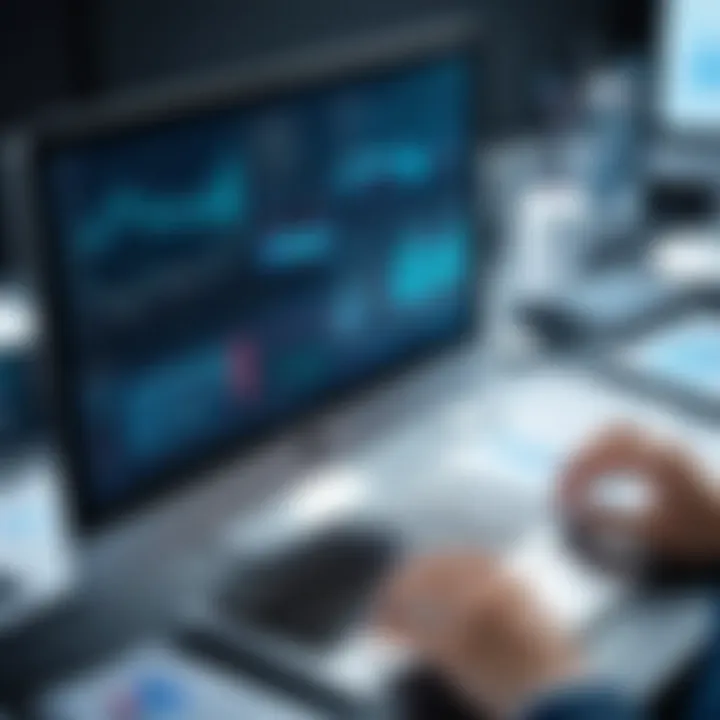
In the complex landscape of clinical research data management, selecting the right software is not a trivial task. A comparative analysis of leading software solutions becomes crucial for organizations aiming to optimize their research processes. By evaluating the strengths and weaknesses of various options, stakeholders can make informed decisions that align with their specific needs. This analysis focuses on essential elements such as user interface, data security, integration capabilities, and customer support.
Software A: Key Features and Usage
Software A, known for its robust data collection features, has carved a niche in the clinical research sphere. It allows users to customize data forms and ensures that data input is user-friendly. Additionally, it offers seamless integration with electronic health records, which is a significant advantage for clinical trials. Users appreciate its automated reporting capabilities, which save time and reduce errors during data analysis.
The usage of Software A in clinical settings indicates a steady rise in adoption due to its positive reputation among researchers. Many organizations have reported increased efficiency in managing large datasets without compromising data integrity. It's ideal for clinical trials where data accuracy is paramount.
Software B: Advantages and Disadvantages
Software B presents a different set of characteristics. Among its advantages, the scalability of the software stands out. It can grow alongside the needs of a clinical research organization, accommodating various trial sizes. Furthermore, it has strong security features that comply with regulations.
However, users have noted some disadvantages. The learning curve for new users can be steep, leading to frustrations during the initial setup phase. Additionally, customer support has been described as needing improvement, where response times can lag during peak periods. Considering these factors is essential when evaluating this software for specific projects.
Software C: Industry Positioning and Feedback
In the realm of clinical data management, Software C has positioned itself as a leader. Its extensive market penetration can be attributed to its commitment to ongoing innovation and user satisfaction. Feedback from users highlights its intuitive interface, which facilitates a smooth navigation experience.
Moreover, the software has garnered praise for its continuous updates that respond to industry needs. Users have shared positive insights on how Software C integrates advanced analytics tools, allowing researchers to derive meaningful insights from their data. However, it faces criticism for its cost, which may deter smaller institutions from adopting its solutions.
"In a field as nuanced as clinical research, the right data management software can significantly influence the success of a project."
Assessing these software solutions will equip organizations with the necessary insight to choose tools that drive efficiency and enhance data management practices. Each software has unique offerings and potential drawbacks, which should be carefully weighed against the organizational goals.
Metrics for Evaluating Software Effectiveness
In the landscape of clinical research data management software, evaluating effectiveness is crucial. Metrics provide a quantitative basis for assessing how well a software solution meets its intended goals. Understanding these metrics allows organizations to make informed decisions.
Importance of Metrics in Clinical Research
Metrics serve as a guiding star for organizations. They enable teams to assess whether the software enhances productivity, maintains data integrity, and complies with regulatory standards. The right metrics can highlight performance issues and provide actionable insights. This is particularly relevant given the critical nature of data in clinical research.
Key Metrics to Consider
There are several metrics to evaluate software effectiveness. The following metrics are essential:
- User Satisfaction: A measure of how well users feel the software meets their needs. This can be gathered through surveys and feedback.
- Data Accuracy: This refers to the precision of the data being managed. High accuracy is vital in clinical research.
- System Reliability: This assesses the uptime of the software. Consistent downtime could lead to significant disruptions.
- Speed of Data Retrieval: Quick access to data enhances decision making. Measuring the time it takes to retrieve information is key.
- Compliance Rates: This metric evaluates adherence to regulatory requirements. Ensuring compliance is non-negotiable in clinical settings.
Benefits of Effective Metrics
Using metrics effectively can lead to numerous benefits:
- Enhanced Decision Making: Metrics provide a clear view of performance, encouraging data-driven choices.
- Resource Allocation: Understanding which aspects of the software require improvement helps in allocating resources efficiently.
- Risk Mitigation: Metrics can help identify potential issues before they escalate into larger problems.
- Increased Accountability: Clear metrics allow for better accountability across teams and departments.
Considerations for Implementation
While selecting metrics, it is important to consider several factors:
- Relevance: Choose metrics that align closely with organizational goals.
- Measurability: Ensure chosen metrics can be easily measured and tracked over time.
- Simplicity: Overly complex metrics can obscure insights rather than reveal them.
- Continuous Review: Metrics should not be static. Regular review ensures they remain relevant and effective.
"Understanding and implementing the right metrics is crucial for clinical data management software to succeed in today's data-driven landscape."
Through robust metrics, organizations can not only understand the effectiveness of their software but also continually improve performance. This ensures that clinical research projects proceed smoothly and effectively, ultimately benefiting patient care and outcomes.
Adoption Trends in Clinical Data Management Software
In the realm of clinical research, data management software is evolving rapidly. Various trends are reshaping how organizations approach data management. Understanding these trends helps professionals stay abreast of what tools and practices will enhance their research efficiency. Identifying the importance of shifts towards innovative solutions is crucial for making informed decisions.
The ongoing transition towards cloud-based and mobile data management is significant. Researchers are increasingly recognizing the advantages these technologies bring. Cloud solutions facilitate scalability, allowing institutions to adjust resources according to their needs. Meanwhile, mobile access is not just a convenience; it becomes a necessity in our fast-paced world.
"The adoption of modern technologies in clinical research is not merely an option but a strategic imperative."
Shift towards Cloud-Based Solutions
Cloud-based solutions have been at the forefront of transformation in clinical data management. Organizations find these solutions attractive because they reduce the infrastructure costs associated with on-premises systems. With cloud solutions, data is hosted remotely, allowing researchers to access information from virtually anywhere. This flexibility fosters collaboration among teams spread across various locations.
Moreover, cloud solutions offer robust data security measures. Sensitive information can be protected through advanced encryption technologies. These features help organizations comply with rigorous regulatory standards, such as HIPAA and GDPR, ensuring data privacy.
Some specific benefits include:
- Cost-effectiveness: Lowering IT overhead and maintenance.
- Scalability: Ability to expand services without disrupting ongoing projects.
- Collaboration: Enhanced sharing capabilities among researchers and stakeholders.
- Backup and Recovery: Reliable data backup solutions reduce risks of data loss.
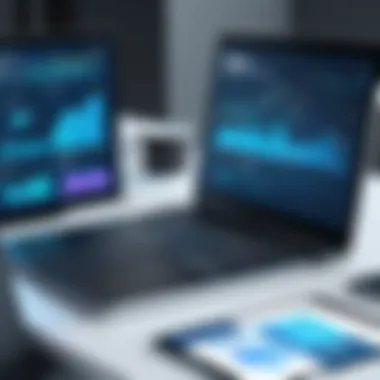
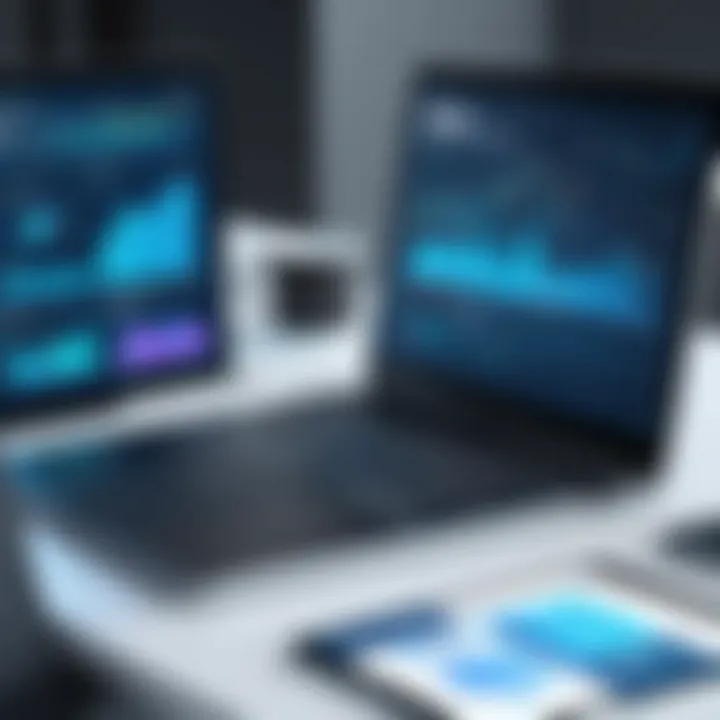
Rise of Mobile Access to Data
Mobile access to clinical data is another trend that cannot be overlooked. With the increasing reliance on smartphones and tablets, researchers can now perform tasks on-the-go. This advancement leads to improved efficiency and real-time decision-making.
Not only does mobile access facilitate data entry and management, but it also allows researchers to collect data from subjects directly. This capability is vital in clinical trials, where timely data collection can significantly impact study outcomes.
Among the notable considerations are:
- User Experience: Intuitive interfaces make it easier for researchers to adapt.
- Real-Time Updates: Immediate access to up-to-date information supports quick decision-making.
- Increased Engagement: Participants may be more likely to interact when using familiar devices.
The ongoing changes in data management software underscore a critical trajectory in the clinical research landscape. By embracing cloud-based solutions and mobile access, organizations optimize operations and meet the demands of modern research methodologies.
Future Innovations in Data Management Software
The realm of clinical research data management is poised at the brink of transformative change. Future innovations in this field promise to redefine how data management software operates. The integration of advanced technologies is essential, creating opportunities for enhanced efficiency and accuracy in data handling. Software developers and IT professionals must stay informed about emerging trends. This discussion addresses pivotal elements, advantages, and considerations concerning future innovations in data management software.
Integration of Artificial Intelligence
Artificial intelligence (AI) stands out as a core innovation for clinical research data management software. AI can automate various processes, significantly reducing the manual workload. Its capabilities include predictive analytics, which can anticipate data trends based on historical patterns. This not only enhances decision-making but also increases the speed at which research outcomes can be produced.
AI can also improve data accuracy and integrity. Machine learning algorithms can be trained to identify anomalies within datasets, flagging potential errors for human review. This is pivotal in maintaining the compliance needed in clinical research, allowing for immediate response to discrepancies.
According to a report, the application of AI in clinical settings can decrease the data entry and verification time by up to 50%.
Implementing AI can initially require significant investment and adjustments to existing systems. Organizations need to consider the training of personnel to ensure they can effectively collaborate with AI tools and interpret the data generated. Overall, AI is not merely a trend, but a substantial enhancement that offers long-term benefits for data management practices in clinical research.
Enhancements in Data Visualization Tools
Data visualization is another crucial facet of future innovations in data management software. The complexity of clinical data necessitates tools that can distill vast amounts of information into comprehensible formats. Advanced data visualization tools enable researchers to identify patterns and gain insights quickly and efficiently.
With real-time data visualization, stakeholders can monitor clinical trials as they unfold. This immediacy allows for prompt adjustments to study protocols and improves communication among team members. Moreover, these tools can enhance presentations to regulatory bodies, showcasing robust data through rich visual narratives.
- Improved data visualization tools may include:
- Interactive dashboards that display real-time analytics.
- Heat maps that reveal spatial patterns in data.
- Graphs and charts for easier comparison of study results.
The evolution of data visualization technologies also means more accessibility. Cloud-based platforms facilitate collaboration among teams based in different geographic locations. Such enhancements build a framework where insights flow freely, fostering innovative approaches to clinical research.
In summation, the future of clinical research data management software appears promising with the integration of artificial intelligence and upgrades in data visualization. Professionals in the field must look ahead, preparing for the implementation of these innovations. By doing so, they will not only stay relevant but also play a crucial role in advancing clinical research methodologies.
Strategies for Successful Implementation
Successful implementation of clinical research data management software is crucial for enhancing research efficiency and ensuring data integrity. The effectiveness of such software largely depends on how well it fits into the existing workflows and structures of the organization. Implementing solutions that align with organizational goals not only aids in maximizing the software's utility but also ensures compliance with regulatory requirements.
Assessing Organizational Needs
To begin with, understanding organizational needs is paramount. Each institution has unique requirements, influenced by factors like size, type of research, and target demographic. A thorough assessment helps in identifying specific functionalities that the software must offer. Here are some steps involved in this assessment:
- Conduct Stakeholder Interviews: Engaging different departments can provide insights into various data needs and processes. Feedback from users who will rely on the system offers essential information.
- Evaluate Existing Tools: Analyze the current systems in place. Understanding what works and what doesn't will help fine-tune the selection criteria for new software.
- Identify Compliance Requirements: Different organizations may have various compliance responsibilities. A clear understanding of these needs will ensure that the chosen software aligns with regulatory standards.
The outcome of this assessment will provide a foundation for making informed decisions, leading to a more harmonious integration process.
Training and Support for Users
Once a suitable software is selected and implemented, investing in comprehensive training and support for users is vital. This training can enhance user confidence and proficiency, ultimately leading to better utilization of the software's features. Consider the following elements:
- Customized Training Sessions: Tailoring training programs based on user roles can aid in addressing specific functionalities relevant to different departments.
- Ongoing Support: Establish a system for continuous support, such as help desks or forums, to assist users as they navigate through the software.
- Feedback Mechanisms: Encourage users to share their experiences with the software. Gathering this feedback can help in refining training materials and support processes.
Investing in thorough training ensures that users are not merely using the software but are leveraging it fully for improved data management outcomes.
The best training programs include hands-on sessions, covering both basics and advanced features. This approach fosters a sense of ownership among users, making them more likely to engage fully with the software.
Strategies for successful implementation address both the immediate technical needs and the broader organizational context. Careful consideration of organizational needs combined with effective training and support forms a strong foundation for realizing the benefits of clinical research data management software.
Finale and Takeaways
In the realm of clinical research, effective data management software plays a crucial role in supporting high-quality research outcomes. This article has articulated the significance of this software by outlining key functionalities such as data collection, validation, and reporting. These elements are essential for maintaining data integrity and ensuring compliance with rigorous regulatory standards.
The examination of challenges faced in clinical data management highlights the complexity researchers encounter. Ensuring data integrity, handling large datasets, and integrating diverse data sources are not mere obstacles but pivotal aspects that affect the entire research process. Therefore, understanding these challenges allows professionals to proactively address potential setbacks.
Moreover, we discussed notable market trends, including the shift toward cloud-stored solutions and the increasing demand for mobile access. These trends indicate that organizations must embrace innovative practices to stay competitive. Future innovations, especially those involving artificial intelligence and enhanced data visualization tools, promise to further streamline operations.
When evaluating software options, taking into account adoption trends and success metrics will provide a clearer picture of what to pursue. Analyzing Software A, B, and C has shown how different features cater to varying organizational needs. Ultimately, strategies for successful implementation are just as vital, as they dictate how well a team can adapt to and leverage these technologies.
In summary, a thorough understanding of clinical research data management software is imperative for professionals in this field. It enables them to make informed decisions that enhance research efficiency, ensuring that valuable insights lead to impactful medical advancements. As the landscape continues to evolve, staying abreast of the latest developments will be key for anyone involved in clinical research.
"Adopting the right clinical data management software can significantly impact research success, providing tools for better decision-making and clearer insights into data trends."
By synthesizing this information, professionals can better navigate the complexities of clinical research data management. Awareness of trends, challenges, and software capabilities ensures readiness to meet future demands.