Integrate Snowflake and Power BI for Enhanced Analytics
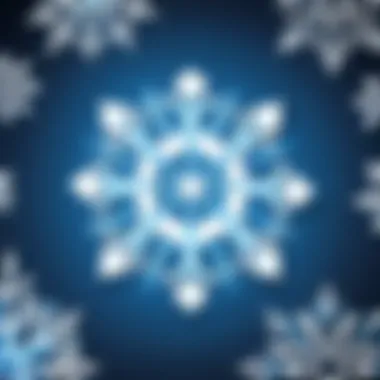
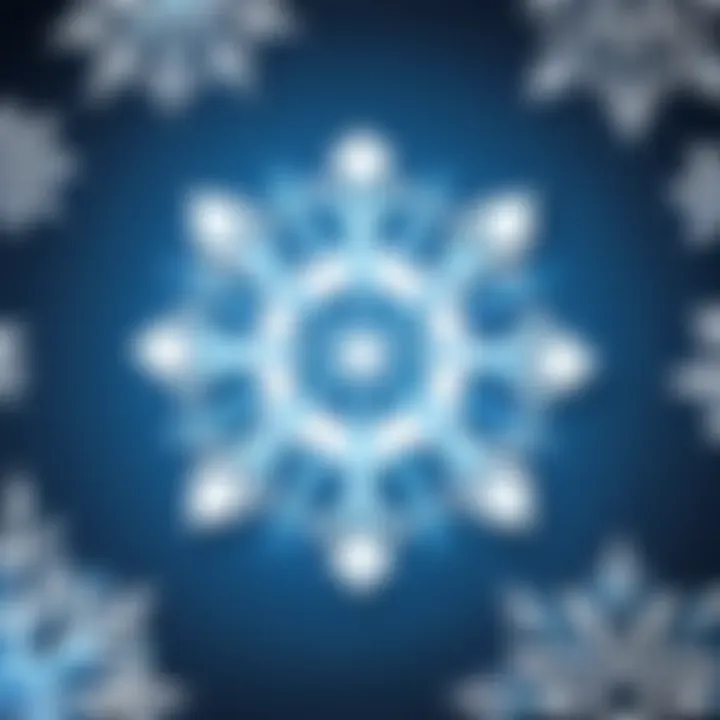
Intro
With the avalanche of data in this digital age, businesses need powerful tools to make sense of it. Two such tools, Snowflake and Power BI, have emerged as titans in their respective domains. Snowflake serves as a robust cloud-based data warehousing solution, designed to handle everything from raw storage to complex querying. On the other hand, Power BI, developed by Microsoft, stands tall as a versatile analytics service that transforms raw data into insightful reports and visualizations. Together, they represent a formidable strategy for organizations aiming to elevate their data management and analytical prowess.
This article unpacks the journey of integrating Snowflake with Power BI, detailing the technical necessities, installation steps, and tried-and-true practices to optimize this connection. As you dig through these sections, expect to gather rich insights that could empower team members from various backgrounds, whether they are software developers, IT professionals, or students embarking on their data analytics journey.
Software Overview
Purpose and function of the software
Snowflake is not just any run-of-the-mill data storage solution. Instead, it’s designed to deal with the immense variety and velocity of big data. Its architecture separates storage and computing, enhancing scalability and performance. Organizations use Snowflake to consolidate varied data sources, ensuring everything is accessible for querying and analysis. In essence, it's a one-stop-shop for data warehousing in the cloud.
On the flip side, Power BI allows users to create compelling reports and dashboards. Think of it as the canvas on which data turns into a work of art. With its drag-and-drop capabilities, analysts can easily slice and dice datasets, discovering patterns and insights that might otherwise fly under the radar.
Key features and benefits
- Customizable Dashboards: Power BI’s ability to create personalized dashboards enables stakeholders to visualize exactly what’s crucial for their decision-making.
- Real-time Data Monitoring: Staying current is essential; Power BI facilitates the fluid update of reports as the underlying Snowflake data changes.
- Robust Security: Data security is paramount. Both platforms comply with industry standards, helping businesses keep their information safeguarded while still accessible.
Here’s a nugget of wisdom: when Snowflake's capabilities combine with Power BI’s visualization strengths, the synergy can lead to streamlined operations and informed decisions.
"The combination of Snowflake and Power BI transforms heaps of data into actionable insights. It's like having a compass in a data forest."
Installation and Setup
System requirements
Before diving into the installation of either platform, first, ensure your systems meet the necessary requirements.
- Snowflake: As a cloud-based solution, it primarily requires a compatible web browser. However, for optimal operation, a stable internet connection and some level of data governing strategy are beneficial.
- Power BI: For desktop installations, Power BI operates on Windows 10 or later. It requires a minimum of 1 GB of RAM (2 GB or more is preferred), and about 1 GB of available disk space should be allocated for a seamless experience.
Installation process
The installation for Snowflake is straightforward, largely requiring you to sign up for an account on their website. Here’s a brief walkthrough:
- Create an account on the Snowflake website. Choose your preferred cloud provider and region.
- Set up a data warehouse. After the account is active, follow the prompts to define your data structure.
- Connect data sources. Use Snowflake's interface to connect to various data platforms, whether it’s a database or a simple CSV file.
Next comes Power BI:
- Download Power BI Desktop from the official Microsoft site.
- Run the installer: the steps are mostly click-and-go, just pay attention to the installation destinations if you have specific folders set aside.
- Launch the application and connect it to your Snowflake data warehouse using the connection wizard.
Prelude
In today’s world, data has become a critical asset for businesses seeking to navigate the complexities of decision-making. The interplay between Snowflake and Power BI showcases the synergy that can be achieved by effectively harnessing data. Snowflake provides a robust, cloud-based data warehousing solution, while Power BI serves as an effective tool for data visualization and analytics. Together, they empower organizations to unlock valuable insights and foster informed strategic decisions.
By establishing a seamless connection between these two powerful platforms, users can tap into the vast capabilities of Snowflake's data management and Power BI's analytical prowess. This integration is more than just a technical requirement; it represents a transformative approach to data handling and reporting. Organizations can create detailed reports, visualize complex datasets, and derive actionable intelligence in a way that enhances responsiveness and agility in the business environment.
Key benefits of linking Snowflake to Power BI include:
- Enhanced Data Visualization: With Power BI's user-friendly interface, stakeholders can easily interpret insights from data kept in Snowflake.
- Real-Time Analytics: Connecting these tools allows for real-time data processing and reporting, ensuring that users are working with the latest information.
- Scalable Data Solutions: Both platforms are designed to handle increasing data volumes, making it easier for businesses to grow without running into bottlenecks.
- Cost-Effectiveness: By utilizing cloud services, companies can minimize upfront costs and adapt their infrastructures according to actual needs.
However, it’s vital to consider several aspects before diving into the integration process. Understanding specific prerequisites, security settings, and best practices is essential for ensuring a smooth and efficient connection. Knowledge of these elements not only optimizes the connection process but also safeguards the integrity and security of the data being handled.
Understanding Snowflake
To appreciate the full potential of integrating Snowflake with Power BI, it is crucial to grasp what Snowflake is and how it operates. This understanding informs the connectivity process, data modeling, and ultimately, the insights derived from analytics. Snowflake, being a cloud-based data warehousing solution, is designed for scalability, performance, and a robust architecture that separates compute from storage. For organizations aiming to harness the power of data in decision-making, comprehending these elements is not just beneficial—it’s foundational.
Overview of Snowflake Architecture
Snowflake is built on a unique architecture that separates its storage and compute layers. This separation allows for independent scaling, meaning organizations can adjust resources based on their specific workload needs. In simpler terms, think of it like a large warehouse where goods can be stored and forklifts can be called in as needed.
At its core, Snowflake has three main components:
- Cloud Storage: This holds all the data, which can vary in type and size. Whether it’s structured data like sales information or semi-structured data like JSON files, everything is stored securely.
- Compute Layer: Here lies the processing power that runs queries and performs analytics. This layer can be scaled up or down depending on whether a large data set needs to be analyzed or just a quick report.
- Services Layer: This includes everything from the user interface to the monitoring tools that assist users in managing their data warehouse efficiently.
Understanding this architecture enables better planning concerning data processing and how to leverage Power BI for visualization effectively.
Key Features of Snowflake
Snowflake brings numerous features to the table, ensuring that it can meet varied organizational data needs. Here are significant highlights:
- Seamless Data Sharing: Snowflake facilitates easy and secure data sharing across different organizations without data duplication.
- Automatic Scaling: It intelligently scales during peak loads and reduces resources when demand decreases, saving costs in the process.
- Support for Multiple Data Formats: Unlike traditional data warehouses, Snowflake handles structured and semi-structured data, thus enabling a versatile approach to analytics.
- Concurrency Management: Multiple users can run queries simultaneously without performance degradation, reducing bottlenecks during busy periods.
- Data Cloning: It allows users to create copies of databases instantly without using extra storage, making it easy to experiment and conduct testing.
Each of these features significantly enhances how organizations can manage, analyze, and derive insights from their data, making Snowflake a vital part of modern data strategies.
Common Use Cases for Snowflake
Snowflake shines in various scenarios across industries, emphasizing its versatility. Common applications include:
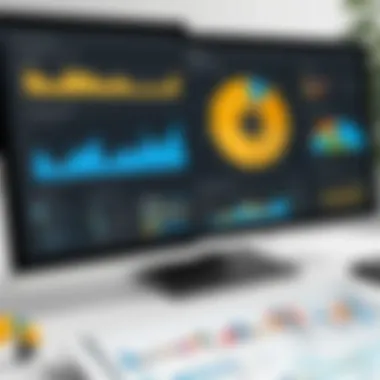
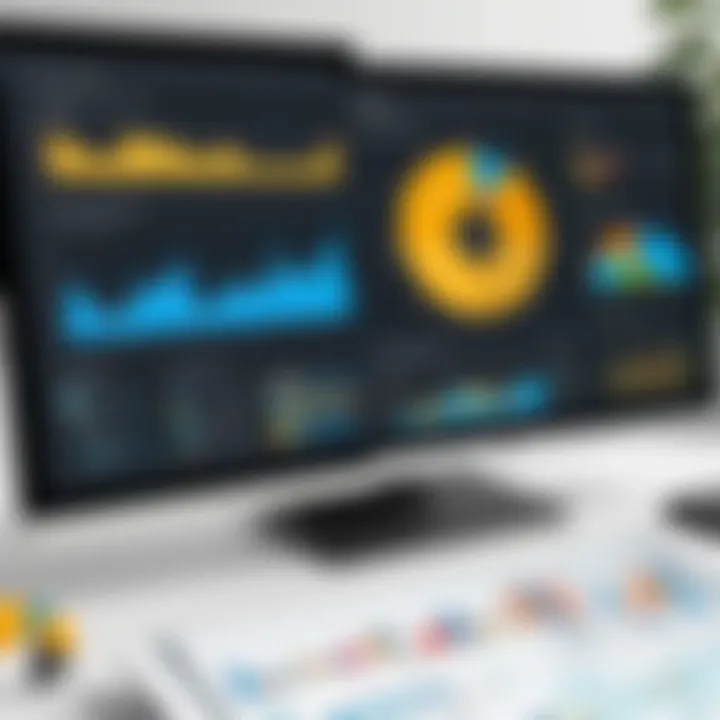
- Data Warehousing: Businesses leverage Snowflake to centralize their data for reporting and analysis, gaining clarity and insights that inform strategic choices.
- Data Lakes: The capacity to handle diverse data types reveals uses in establishing data lakes, where raw data can be stored and transformed as needed.
- Business Intelligence: By integrating with tools like Power BI, Snowflake acts as a backend support, powering intricate data visualizations and dashboards that allow for efficient monitoring of key performance indicators.
- Machine Learning: With data easily accessible, data scientists can fuel machine learning models directly from Snowflake, driving innovation and automation.
As organizations continue to navigate the complexities of data analytics, understanding these use cases helps illustrate why Snowflake is a favorable option for many businesses wanting to enhance their analytical capabilities.
"Snowflake’s potential lies not just in its architecture but in the diverse applications that organizations can harness to drive measurable outputs."
Understanding Snowflake is not merely about recognizing its features but realizing its impact on data-driven strategies. With a robust understanding of its architecture, key features, and common use cases, the groundwork is set for a seamless connection to Power BI, thus unlocking unparalleled analytical capabilities.
Exploring Power BI
In the realm of data analysis, Power BI emerges as a critical player, enabling organizations to turn data into compelling narratives. This section will take a closer look at Power BI, emphasizing its significance in conjunction with Snowflake. Understanding Power BI is not just about knowing its functionalities; it’s about recognizing its potential to elevate data analytics and visualization to new heights.
Preface to Power BI
Power BI, developed by Microsoft, stands out as a robust business analytics tool that collates data from various sources and transforms it into insightful reports and dashboards. With a user-friendly interface, it caters to both technical and non-technical users. People can drag and drop elements, manipulate datasets, and visualize trends without needing extensive programming knowledge.
The tool offers a myriad of capabilities. Users can connect to a multitude of data sources, including Excel spreadsheets, databases, and, notably, cloud services like Snowflake. The ability to seamlessly integrate with existing databases allows businesses to leverage historical data while adding value to their reporting processes.
Power BI Components and Functions
Power BI encompasses several key components that work in harmony to deliver analytics capabilities:
- Power BI Desktop: This is where users design their reports. It allows for data shaping and visualization.
- Power BI Service: A cloud service that enables easy sharing and collaboration of reports and dashboards.
- Power BI Mobile: An app that allows users to access reports on the go, ensuring data availability at any time.
- Power BI Gateway: A bridge that connects on-premises data sources to the Power BI service, facilitating seamless data refreshes.
Each of these components serves a unique purpose, contributing to a collaborative ecosystem in data analytics and reporting. For instance, Power BI Desktop lets you create intricate reports with interactive visuals, while the Power BI Service is essential for sharing insights across teams.
Why Use Power BI for Analytics?
The advantages of utilizing Power BI for data analytics are manifold:
- Data Integration: Power BI can pull data from a variety of sources including Snowflake, making it easy to harness big data for analysis.
- Interactive Visuals: Its strong visualization capabilities help make sense of complex datasets. Users can create graphs, charts, and maps that are not only aesthetically pleasing but also insightful.
- Real-Time Reporting: Businesses can take advantage of real-time data updates. Whenever data in connected systems refreshes, Power BI can automatically update reports.
- Collaboration Facilitation: With the Power BI Service, teams can share reports and insights, ensuring that everyone is on the same page regarding data interpretation and decision-making.
"Power BI empowers users to capture the story within their data, transforming numbers into actionable insights."
Establishing Connectivity
Prerequisites for Connection
Before diving into the connection process, a few essential prerequisites must be in place. Having these elements sorted out can save a world of headaches down the road.
- Snowflake Account: You need a Snowflake account set up with permissions to use the data warehouse. Ensure that the user role has the proper access rights to the data you intend to analyze.
- Power BI Desktop: Download and install the latest version of Power BI Desktop. It provides the tools you need to visualize data from Snowflake effectively.
- Network Accessibility: Your firewall or VPN settings must allow connections to Snowflake’s cloud services. This can often be a point of contention; make sure that those in charge of IT understand the requirements.
These prerequisites are more than just checkboxes; they lay a solid foundation that ensures smoother communication between Snowflake and Power BI.
Setting Up Snowflake Access
Once prerequisites are lined up, the next step is to create and configure the necessary access on Snowflake. It’s like laying down the rail tracks before the train can roll in.
- User Creation: Navigate to your Snowflake management interface to create a new user if none exist. This is where roles come into play; assign the correct role that has access to the data you want to analyze.
- Database and Warehouse Access: Next, grant access to specific databases and warehouses. Use the following command in your SQL command line:
- Testing Access: To confirm everything is set up correctly, try logging into the Snowflake UI with the created user credentials. Successful login and access to the desired tables indicate that setup is correct.
Failing to properly configure access can lead to nerve-racking errors later when you try to connect Power BI. Having access neatly organized helps facilitate a smoother integration down the line.
Configuring Power BI for Snowflake
With Snowflake access ticked off, it’s time to pivot to Power BI and configure it to connect to your Snowflake instance. This might seem daunting, but taking this step methodically makes it manageable.
- Open Power BI Desktop: Start the application you freshly installed. Familiarize yourself with its interface if you haven't already.
- Obtain Snowflake Credentials: Keep your Snowflake account name, username, and password handy. You’ll need these essential details to ensure a successful connection.
- Select the Data Source: In Power BI, go to the "Get Data" option, and select Snowflake as your data source. This tells Power BI where to look for your data.
- Enter Connection Details: In the connection window, enter the Snowflake account URL, your user name, and password. Make sure to input the right warehouse and database as well. Here’s where it pays off to double-check all entries to avoid connection glitches.
- Test Connection: Most importantly, once you’ve input all the information, use the test connection feature provided by Power BI. It’s a small but critical step to ensure connectivity is solid before moving forward.
Following these steps diligently can help set the stage for a fruitful relationship between Power BI and Snowflake. When connectivity is properly established, the real fun begins, enabling professionals to visualize and analyze data in new and exciting ways.
Connection Process
Implementing this connection properly can enhance your analytics workflow, streamline data retrieval, and improve your overall reporting efficiency. Understanding essential components—and common pitfalls—can save one from unnecessary headaches down the road.
Step-by-Step Guide to Connect
Accessing Power BI Desktop
To kick things off, Accessing Power BI Desktop acts as the gateway to all subsequent actions in this connection process. Think of it like the front door to your data-driven abode. Power BI Desktop is a favorite among professionals because it is rich in functionalities and intuitive in its use, setting the stage for data visualization.
A unique characteristic of Power BI Desktop is its user-friendly interface which allows users to drag and drop elements effortlessly. This makes it an accessible choice for professionals from various backgrounds, whether they come from software development, business analysis, or any other IT field.
Accessing it is simple—just download it from the official Microsoft Power BI site, and you're good to go. However, sometimes users encounter issues if they’re not using the latest version, which may lead to some features being incompatible.
Selecting Data Source
Following that, we have Selecting Data Source. This step influences how Power BI interfaces with your Snowflake data. Choosing the right source is crucial as it determines how efficiently your data is pulled into Power BI.
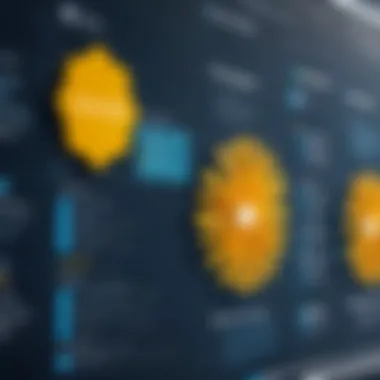
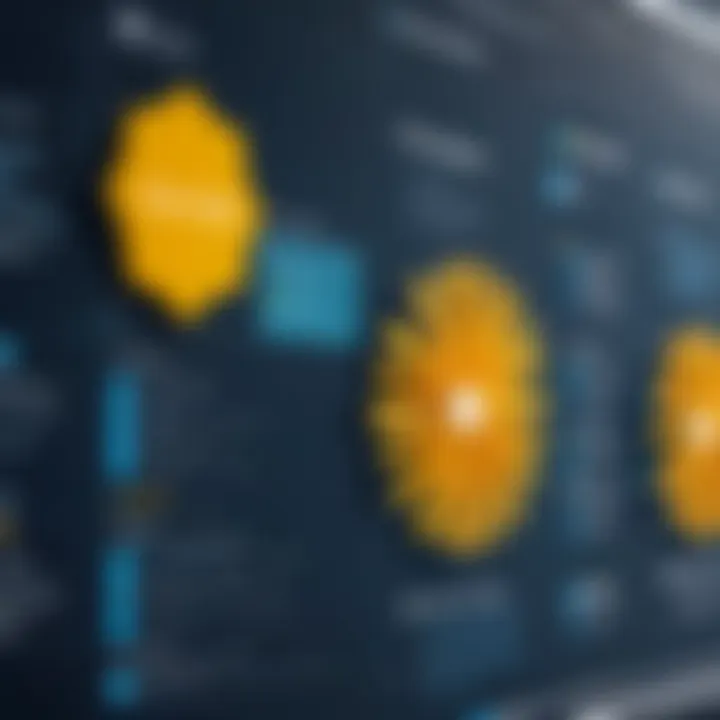
One of the standout features during this selection process is the inclusion of various data source options, including databases, files, and even online services. For integrating Snowflake, you'll specifically navigate to the database section where options for cloud sources are available.
This selection is beneficial because it ensures that you establish the connection with the correct parameters right from the start. However, mistakes here can lead to misconfigured settings, resulting in data that might not be complete or actionable.
Entering Snowflake Connection Details
Now we reach the nitty-gritty part: Entering Snowflake Connection Details. This is where you plug in the necessary information needed to access your Snowflake account effectively.
Here, you'll input your account name, warehouse, database, and schema details. It’s important to note that these details must be precise; otherwise, it can throw a wrench in the whole setup. Getting these details right is a key characteristic of ensuring a successful connection.
The beauty of this process is that Power BI will usually help you troubleshoot some basic errors, which adds an extra layer of ease. However, overlooking certain configurations, such as VPN settings or firewall rules that may hinder access, can cause frustration down the road.
Handling Data Import
Once the connection is established, the next logical flow is to consider how to import your data effectively. This section covers key decisions that will impact performance and usability.
Choosing Import vs. DirectQuery
The choice between Import vs. DirectQuery can significantly affect your analytics strategy. Import mode enables you to pull data into Power BI for faster performance, whereas DirectQuery keeps your data in Snowflake, querying it live.
The key characteristic of Import is speed—loaded data is faster to visualize once fetched. However, for those who need real-time data, DirectQuery is indispensable. The downsides? Import might lead to outdated data if not refreshed regularly, whereas DirectQuery can slow down if the underlying Snowflake queries aren't optimized.
Loading Data Models
Next up is Loading Data Models, which is central to shaping the visualizations you will create in Power BI. This step involves transforming data into models that can be easily analyzed.
Power BI allows for a range of transformations, from simple aggregation to registering complex relationships between tables. This flexibility makes it a favored choice for professionals needing to customize their data representations. However, overly complex models can lead to performance lags, which is a common downside for users who attempt to push the envelope too much.
Refreshing Data
Finally, Refreshing Data is crucial for keeping your reports up-to-date. Whether you choose to go with scheduled refreshes or manual updates, having an effective strategy is key.
The core benefit of having an automatic refresh schedule is that it mitigates data staleness, ensuring that users always have the latest insights at their fingertips. Yet, it's worth noting that too many refresh attempts can lead to overloading your Snowflake account, impacting performance.
Thus, balancing is essential between how often you refresh and how much data you are pulling.
Best Practices
Optimizing Performance
Data Caching Strategies
One of the specific strategies crucial for performance optimization is employing effective data caching techniques. This involves storing copies of frequently accessed data segments in a temporary location. By doing this, users save time since retrieving this data becomes much quicker, which is particularly vital in environments where real-time analytics are a priority. A key characteristic of caching is its ability to significantly reduce load times for reports, thus improving user experience.
In this article, focusing on data caching helps maintain high performance levels during various data operations.
However, one should be careful with cache freshness. Cached data becomes stale if the underlying data changes, possibly leading to inaccuracies. Regularly managing cache refresh rates can be a balancing act between performance and data accuracy. Still, it’s considered a beneficial practice to implement when done thoughtfully.
Efficient Query Writing
Next up is efficient query writing. This means crafting queries in a way that the Snowflake data warehouse can process them with greater speed and less resource consumption. This practice contributes significantly to overall system performance. A well-structured query can lead to faster result sets and less strain on databases.
These queries can make all the difference in reducing time when pulling data into Power BI dashboards.
The unique advantage of efficient query writing lies in its ability to minimize the use of resources. Such optimization ensures that database operations can run smoothly, even under heavy loads. However, it requires a good grasp of both SQL syntax and Snowflake’s functionality, which can be a barrier for some users. But the long-term gains in performance make it a popular choice.
Resource Management
Efficient resource management helps prevent over-provisioning, which occurs when more resources are allocated than necessary, resulting in wasted expenditure.
A distinctive feature of resource management is the ability to automatically scale compute resources up or down based on demand. This feature mitigates the risk of performance bottlenecks during peak usage times. However, it’s essential for users to have sound knowledge of their usage patterns to use this feature effectively. Thus, this practice is invaluable for anyone serious about leveraging Snowflake and Power BI effectively.
Security Considerations
Managing Role-Based Access
One big component is managing role-based access. This ensures that individuals have access only to the data and resources they are authorized to view. A key characteristic of this practice is its capability to minimize the risk of unauthorized data access, which is a critical requirement in today’s data-driven landscape. Crucially, role-based access simplifies administrative tasks by centralizing permission settings.
However, misconfigured roles can become a pitfall, leading to either overly broad data access or unwarranted restrictions. Clear guidelines and regular audits can help avoid these issues.
Implementing Data Encryption
When it comes to securing data in transit, implementing data encryption cannot be neglected. This practice safeguards sensitive information from potential breaches during transmission between Snowflake and Power BI. It ensures confidentiality and protects against identity theft. Encryption should be the norm—merely a good habit to keep in mind.
One unique feature of data encryption is that it offers various encryption algorithms to choose from, allowing companies to select a method that best fits their security needs. The downside? While encryption protects data, it might introduce some latency during transmission. Still, this balanced approach is invaluable for sensitive data handling.
Auditing and Monitoring Connections
Lastly, auditing and monitoring connections can play a critical role in security. Regular audits help ensure compliance with internal policies and external regulations, which is essential in environments that handle sensitive data. Auditing tracks data access patterns and detects any irregular or unauthorized access. An important aspect of auditing is that it can alert administrators to suspicious activity, allowing for quicker responses to potential threats. On the flip side, maintaining a comprehensive audit log can be resource-intensive and complex to manage. Nevertheless, incorporating auditing acts as a line of defense that cannot be overlooked.
Advanced Features
The integration of Snowflake with Power BI opens the door to advanced features that can significantly amplify data analysis capabilities. These advanced capabilities are not just add-ons; they are essential tools for professionals looking to harness the full potential of their data. Utilizing these features can lead to more insightful visualizations and faster decision-making processes. In this section, we will explore how Snowflake's functionalities can be effectively used within Power BI and focus on essential data modeling techniques that enhance analytics performance.
Utilizing Snowflake Functions in Power BI
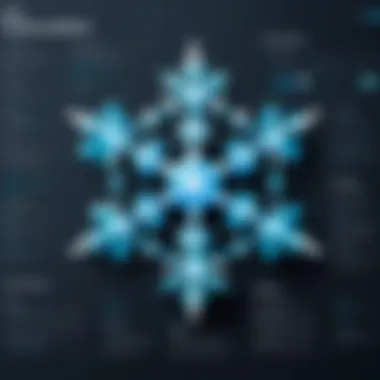
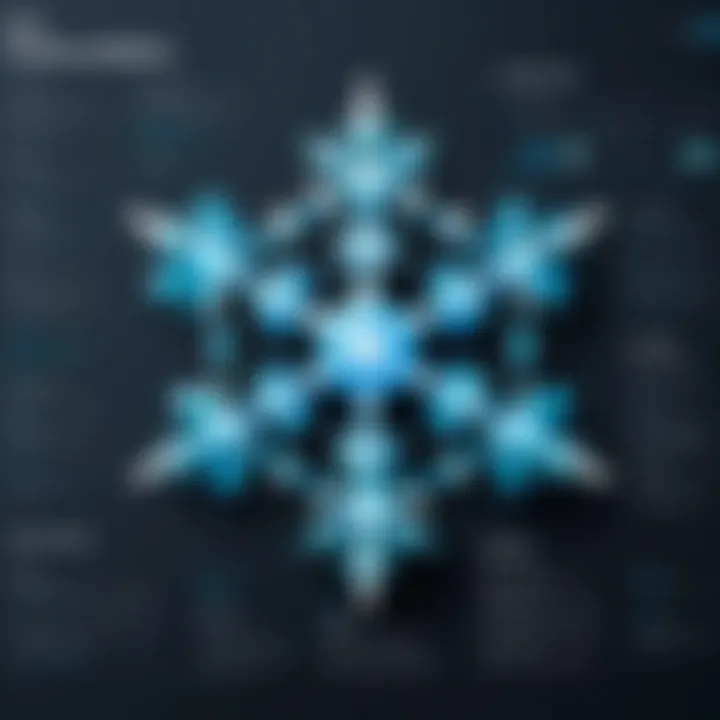
The first aspect to delve into is the Snowflake functions. These functions are quite powerful when incorporated into your Power BI reports. They provide a unique way to perform in-database calculations, streamlining the process. For instance, let's say you need to analyze sales data. Instead of pulling all that data into Power BI and crunching the numbers there, you can leverage Snowflake’s compute power.
Some commonly used functions include:
- Aggregate Functions: These let you perform calculations on your data, providing insights like totals or averages without moving data out of Snowflake.
- Window Functions: They are especially useful for analytics, letting you perform calculations across a set of table rows related to the current row.
- JSON Functions: If your data includes semi-structured formats, Snowflake's JSON functions allow for easy manipulation and querying.
Integrating these functions into your Power BI reports can greatly enhance performance, as calculations occur at the source.
"Using Snowflake functions within Power BI not only saves time but also reduces the load on your reporting tools, allowing them to work more efficiently."
Data Modeling Techniques
When it comes to data modeling, the aim is to create a structure that allows for efficient data retrieval and insightful analysis. In Power BI, effective data modeling can help simplify complex datasets, making the analysis more intuitive.
Some key data modeling techniques to consider include:
- Star Schema Modeling: This is a method where you create a central fact table connected to multiple dimension tables, optimizing query performance.
- Normalization/Denormalization: Depending on your analysis needs, you may choose to normalize your data to reduce redundancy or denormalize it to enhance query speed.
- Hierarchies: Creating hierarchies in your data model enables users to drill down into details without losing the context of the larger dataset.
By carefully designing your data model using these techniques, you not only ensure better performance but also improve usability for end-users who are accessing reports through Power BI. Proper data modeling has a direct impact on the speed and efficiency of query execution in Snowflake, which is crucial for timely decision-making.
Troubleshooting Connection Issues
Troubleshooting is not just about fixing problems; it’s about learning and growing from them. By recognizing frequent issues and implementing solutions, you can save a significant amount of time and resources. This can improve your overall workflow and ensure a seamless integration of your data avenues. Thus, let’s delve into the nitty-gritty.
Common Error Codes and Solutions
In the realm of connecting Snowflake with Power BI, several error codes may rear their heads. Here's a rundown of some frequent culprits and their fixes:
- Error Code 404: This indicates that the data source cannot be found. Verify your Snowflake account name, user info, and password. Sometimes, it's as simple as a typo or an incorrect endpoint.
- Error Code 10001: This typically points to network connectivity issues. Check if your internet connection is stable and that there are no firewalls blocking access to Snowflake.
- Error Code 300004: A common one highlighting authentication issues. Ensure that the account you are using has enough permissions to access Snowflake resources. If not, adapt the role settings accordingly.
- Error Code 401: This one signifies unauthorized access. Double-check your credentials, ensuring that your username and password are accurate.
"The best way to learn is by overcoming challenges. Each error encountered is a stepping stone to mastery."
When you come across these codes, always refer to Snowflake’s comprehensive documentation or user forums for additional insight. The community often shares solutions that can be very effective.
Connection Timeout Strategies
Connection timeout can be an irritating issue when you're trying to work with data in real time. Typically, this occurs when the Power BI application is unable to establish a connection to Snowflake within a designated timeframe. Here are some strategies to avoid or alleviate this problem:
- Adjust Timeout Settings: Consider increasing the timeout duration in Power BI. Power BI has default settings which might not be sufficient under heavy data loads or poor network conditions. Tweaking these settings can sometimes do the trick.
- Network Reliability: Ensure that you have a steady and reliable internet connection. Using a wired connection instead of Wi-Fi can also help enhance the stability.
- Monitor Performance: Regularly check the performance metrics of both Snowflake and Power BI. High query loads or heavy data pulls can lead to higher chances of time-outs.
- Optimize Queries: Review your queries. Long-running queries can cause time-outs. Optimizing your SQL queries can improve performance and lessen the load on your connection.
- Limit Data Size: If you're importing all the data at once, consider testing with smaller batches to see if the connection stabilizes.
By implementing these strategies, you can mitigate the likelihood of encountering connection timeouts, and focus on analysis rather than troubleshooting.
Closure
The blend of Snowflake’s robust cloud-based architecture with Power BI’s user-friendly interface allows for streamlined data visualization. By leveraging Snowflake, businesses gain the flexibility and scalability required to handle large data workloads. Meanwhile, Power BI serves as an accessible tool that turns complex datasets into insightful, actionable reports.
Consider the ease of monitoring performance trends or analyzing customer behavior, which can often be burdened by data silos. The combined power of these two platforms ensures that teams are not only working with live data but also empowering their decision-making processes in real time.
"The value of analytics lies not in the data itself, but in the insights derived from it."
Additionally, a few key takeaways from the previous sections include:
- Streamlined Data Process: Connecting these two technologies fosters a smoother data flow from warehouse to visualization.
- Enhanced Performance: Through optimization practices discussed, users see not just better speed, but also improved accuracy in their reports.
- Security Considerations: Emphasis on the security aspects of connecting these platforms ensures peace of mind, vital in our current environment where data breaches are becoming distressingly common.
Ultimately, as businesses continue to navigate through complex data landscapes, integrating Snowflake with Power BI becomes less of a luxury and more of a necessity. Those who successfully implement this connection will benefit greatly, leading to informed decisions based on data that speaks clearly and efficiently. It's not just about connecting two platforms; it's about pioneering a better way to visualize, understand, and act on your business intelligence.
Further Resources
When merging the capabilities of Snowflake and Power BI, having access to further resources plays a significant role. These tools provide crucial support for practitioners and developers navigating through this integration. Not only do they enhance understanding, but they also pave the way for smoother implementation and creative problem-solving.
Documentation and Guides
A solid foundation of knowledge starts with documentation. Snowflake and Power BI both come equipped with extensive official guides that serve as a roadmap for users. These resources often cover everything from initial setup to advanced features that can elevate user experience. Tracking the documentation updates is necessary since both platforms undergo iterations and enhancements frequently.
Key Benefits of Documentation:
- Clarity: Well-organized documentation can demystify complex topics, breaking them into manageable sections.
- Up-to-date Information: Both companies release frequent updates, and their documentation changes to reflect the latest functionalities.
- Step-by-Step Instructions: Ideal for those who prefer a guided approach. Documentation usually includes detailed examples and screenshots that help users grasp the configuration quickly.
For comprehensive guides, consider the official websites:
Online Forums and Communities
When facing challenges or seeking to enhance one's skills, the collective knowledge found in online forums and communities can be invaluable. These platforms allow users to connect, share experiences, and discuss real-world problems and solutions.
Benefits of Engaging in Communities:
- Peer Support: Other users often have faced the same hurdles. Their insights can save you time and prevent common pitfalls.
- Diverse Solutions: Different perspectives on a challenge can unlock innovative solutions you might not have considered.
- Real-World Examples: Community discussions tend to revolve around actual use cases, providing practical understandings that formal documentation might overlook.
Check out platforms like:
Utilizing these resources not only helps in troubleshooting but also enables users to grow their expertise, keeping them at the forefront of data analytics innovations.