Data Analysis for Small Businesses: A Comprehensive Guide

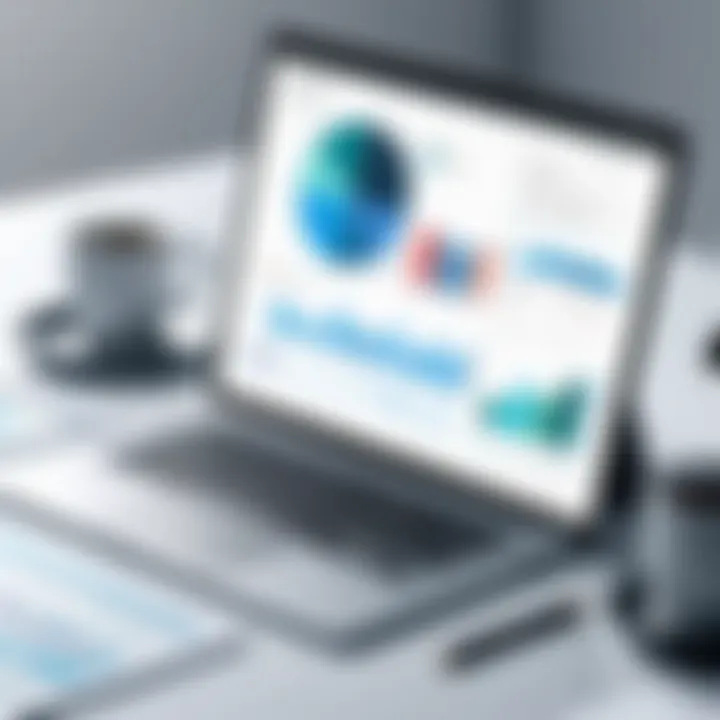
Intro
Data analysis plays a crucial role in the success of small businesses. In today's fast-paced and data-driven world, the ability to leverage data can distinguish a thriving enterprise from one that merely survives. This guide will elaborate on the methodologies, tools, and best practices small businesses can adopt to enhance their decision-making processes.
Understanding different types of data—such as customer feedback, market trends, and sales performance—allows small businesses to make informed decisions. However, analyzing data is not a mere technical task. It involves understanding the implications of data-driven decisions.
This guide will explore essential facets of data analysis, including software options, installation, and setup, alongside practical considerations. Special attention will be paid to the challenges small enterprises face in implementing effective data analysis strategies. Solutions will be offered to ensure that valuable insights from data foster business growth.
Without further ado, let us delve into the Software Overview.
Understanding Data Analysis
Data analysis is a crucial aspect of modern business practice, especially for small enterprises that often face unique challenges. Understanding data analysis involves grasping its definition, significance, and underlying principles that can enhance decision-making processes.
Definition and Importance
Data analysis refers to the process of collecting, organizing, interpreting, and presenting data to extract meaningful insights. For small businesses, this practice is essential. It helps in identifying trends, understanding customer behavior, and making informed decisions that can drive growth. The importance of data analysis cannot be overstated; it allows small businesses to utilize their resources efficiently and effectively.
By adopting data analysis, small businesses can identify key performance indicators (KPIs) that provide a snapshot of business health. They can also uncover hidden patterns within their operations that would otherwise remain unnoticed. This process empowers owners and managers to make strategic decisions based on empirical evidence rather than intuition alone.
Key Principles
Grasping the foundational principles of data analysis is vital for its successful implementation. These principles form the bedrock of effective data-driven decision-making:
- Accuracy: The data must be precise. Inaccurate data leads to flawed conclusions, which can result in poor business decisions.
- Relevance: Only relevant data should be analyzed. Focusing on information that directly impacts business objectives enhances the quality of insights.
- Timeliness: Data analysis should be conducted in a timely manner. Stale data can mislead business decisions which may have significant consequences.
- Scalability: As businesses grow, their data analysis processes must adapt. Scalable solutions ensure that analysis remains effective regardless of the volume of data.
Understanding these principles equips small businesses with the necessary tools to navigate the complexities of data analysis. It enables them to act on insights derived from their data, ultimately leading to enhanced operational efficiency and competitive advantage.
"The goal of data analysis is not just to report numbers, but to derive actionable insights from them."
In sum, understanding data analysis is a multifaceted endeavor that benefits small businesses in numerous ways. By recognizing its definition, importance, and key principles, business owners and decision-makers can leverage data as a powerful strategic asset.
Data Types Relevant to Small Businesses
Data analysis is an intricate process that greatly benefits from understanding various data types. Small businesses can leverage data cutting across different types to optimize their operations, improve decision making, and stay competitive in their market. Each type of data offers unique insights and advantages suitable for different analytical needs. This section outlines three main types: quantitative, qualitative, and transactional data, detailing their relevance and application for small businesses.
Quantitative Data
Quantitative data is numerical and can be measured and analyzed statistically. This type of data provides a clear, objective insight into business performance, consumer behavior, and market trends.
Common sources of quantitative data include sales figures, customer counts, and inventory levels. For example, a small retail shop could track monthly sales figures to identify seasonal trends or spikes in demand for certain products.
Benefits of Quantitative Data:
- Measurable: Quantitative data provides hard numbers that can be tracked over time.
- Statistically analyzable: Advanced statistical methods can be applied to derive meaningful trends and predictions.
- Objective: Reduces bias in decision-making through concrete evidence.
To summarize, quantitative data acts as a foundation for performance metrics and enables small businesses to make data-driven decisions. It's essential for any business aiming to enhance operational efficiency and growth.
Qualitative Data
Qualitative data, in contrast, is non-numerical and deals with descriptions, opinions, and subjective evaluations. This data type is less structured and more challenging to analyze, yet it provides richness and depth to insights regarding customer experiences and expectations.
Sources of qualitative data can include customer feedback forms, interviews, focus groups, and social media comments. For instance, a service-based business could analyze customer reviews to identify common themes or sentiments about their service quality.
Benefits of Qualitative Data:
- Rich insights: Captures customer sentiment, motivations, and behaviors effectively.
- Flexibility: Can be gathered through various methods, adapting to the specific needs of the business.
- Contextual understanding: Offers a narrative that helps in interpreting quantitative data.
Incorporating qualitative data gives small businesses a more rounded view of their operations, allowing them to enhance customer experience and refine their offerings.
Transactional Data
Transactional data is a specific type of quantitative data that records the transactions made by a business. It includes details such as the date, time, amount, and items bought or services provided. This type of data is critical for small businesses as it encompasses every interaction with the customer.
For example, a coffee shop can track each sale through its point of sale system to identify popular products and peak sales times. Understanding these trends allows for better inventory management and staffing decisions.
Benefits of Transactional Data:
- Actionable insights: Directly tied to sales and customer behavior.
- Operational efficiency: Helps in streamlining inventory and optimizing pricing strategies.
- Predictive capabilities: Enables businesses to forecast sales and identify trends over time.
The Data Analysis Process
The data analysis process is central to deriving actionable insights from data. For small businesses, understanding this process can lead to informed decisions that enhance operations and drive growth. Each step in the analysis process can optimize how businesses utilize their data. Key elements of this process include data collection, data cleaning, data exploration, and the application of various analysis techniques. By effectively navigating these stages, small businesses can mitigate risks and capitalize on opportunities.
Data Collection
Data collection is the foundation of the analysis process. This step involves gathering relevant data from various sources, be it internal records, customer feedback, or market research. The types of data collected should align with the objectives set by the business. For instance, sales data can reveal customer buying patterns, while social media insights may indicate brand perception.
Methods of Data Collection:
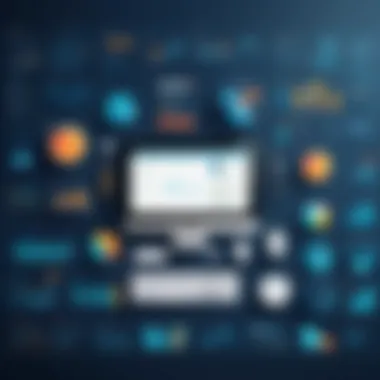
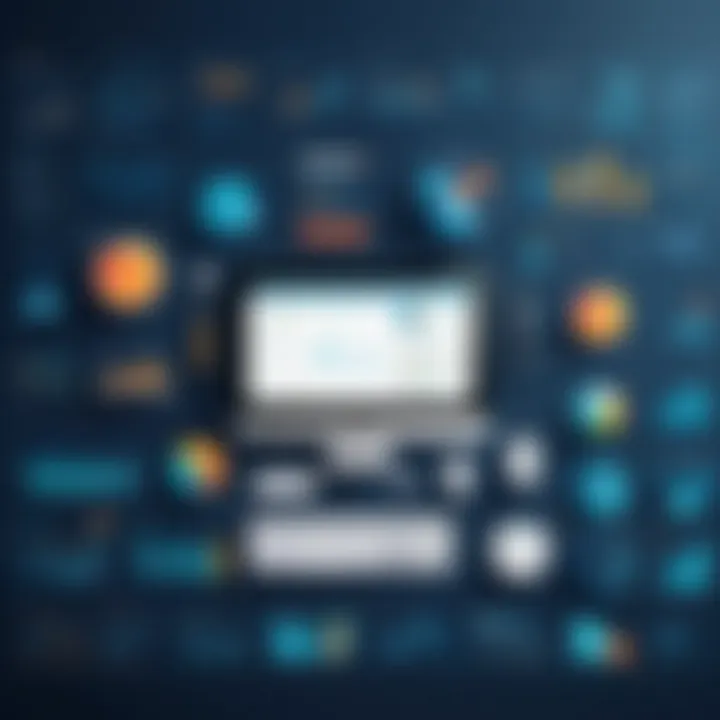
- Surveys and Questionnaires: Useful for gathering customer opinions directly.
- Transaction Records: Internal data that helps in understanding sales trends.
- Web Analytics: Tools like Google Analytics offer insights into user behavior.
Data collection must be systematic and comprehensive. A well-defined process reduces the chances of missing critical information.
Data Cleaning
Data cleaning, often considered an overlooked aspect, is vital for ensuring that analysis is based on accurate information. This stage involves identifying and correcting inaccuracies, duplicates, or inconsistencies within the gathered data. Poor quality data can lead to misleading results, making this step crucial for reliability.
Steps in Data Cleaning:
- Removing Duplicates: Ensures that each data point is unique.
- Correcting Errors: This can include fixing typos or updating outdated information.
- Handling Missing Values: Depending on the context, businesses can choose to fill gaps or remove incomplete records.
A clean dataset allows for more effective analysis and builds trust in the conclusions drawn.
Data Exploration
Data exploration is where analysts begin to examine the dataset to identify patterns, trends, and anomalies. This initial analysis allows businesses to develop questions that can guide deeper analysis. During this stage, visualizations can be particularly useful.
Techniques for Data Exploration:
- Descriptive Statistics: Measures such as mean, median, and standard deviation.
- Data Visualization: Charts and graphs provide quick insights every small business should consider.
- Correlation Analysis: Understanding relationships between different data points can inform further inquiry.
Exploration sets the stage for more complex analysis, enabling businesses to make sense of their data landscape.
Data Analysis Techniques
Data analysis employs various techniques tailored to specific business needs. Three main categories are commonly utilized: descriptive analysis, inferential analysis, and predictive analysis.
Descriptive Analysis
Descriptive analysis focuses on summarizing historical data. It provides a clear picture of what has happened in the business. This technique is a beneficial choice for small businesses as it helps in understanding past performance without needing complicated models.
Key Characteristics:
- Simplified Summaries: Users can easily grasp trends over time.
- Visual Representation: Graphs and charts make data digestible.
While descriptive analysis is advantageous, it lacks predictive capabilities, which means it cannot forecast future outcomes.
Inferential Analysis
Inferential analysis goes a step further by helping businesses make inferences about a larger population, based on sample data. This is important for understanding potential outcomes and making decisions under uncertainty.
Key Characteristic:
- Statistical Testing: Techniques like t-tests and chi-square tests help validate hypotheses.
This analysis is useful for small businesses looking to draw conclusions from limited data, yet it requires a certain level of statistical knowledge that may pose a challenge.
Predictive Analysis
Predictive analysis employs statistical models and machine learning techniques to forecast future events. This type of analysis gives businesses a competitive edge by anticipating customer behavior, sales trends, or inventory needs.
Unique Feature:
- Advanced Modeling: Techniques such as regression analysis or neural networks help in creating simulations.
Though highly beneficial for planning, it often requires sophisticated tools and skills, which can be a barrier for some small businesses.
Key Takeaway: The data analysis process empowers small businesses to leverage their data effectively, ultimately driving better decision-making and enabling sustainable growth.
Tools for Data Analysis
In the realm of small business, effective data analysis is crucial. Tools designed for data analysis increase efficiency and enable sound decision-making. These tools serve various needs, from handling extensive data sets to visualizing trends and generating actionable insights. The selection of appropriate tools can significantly impact the outcomes of data analysis efforts. A small business can leverage suitable technology to transform raw data into structured information.
Spreadsheet Software
Spreadsheet software is often the first tool that comes to mind for data handling. Programs like Microsoft Excel and Google Sheets are widely used because they are user-friendly and versatile. They allow small businesses to perform basic calculations, organize data, and visualize it through graphs and charts.
Benefits of Spreadsheet Software:
- Familiar interface, making it easier for team members to adapt.
- Comes with built-in functions to perform statistical analysis.
- Supports data visualizations, like pie charts and histograms, which simplify the interpretation of data.
However, it’s important to recognize the limitations of spreadsheet software. For larger datasets, performance may decrease. Also, spreadsheets might lack the sophisticated analytical capabilities needed for complex data queries. Therefore, it is essential to assess what data needs may require more than just spreadsheets.
Business Intelligence Tools
Business intelligence tools represent the next step in data analysis sophistication. Tools such as Tableau and Power BI provide small businesses with the ability to convert data into meaningful insights swiftly. They connect to various data sources, allowing for real-time data analysis and reporting.
Considerations for Business Intelligence Tools:
- Enable interactive dashboards that present data visually, aiding in quicker decision-making.
- Provide advanced analytics features like predictive modeling.
- Facilitates collaboration by allowing team members to share insights with ease.
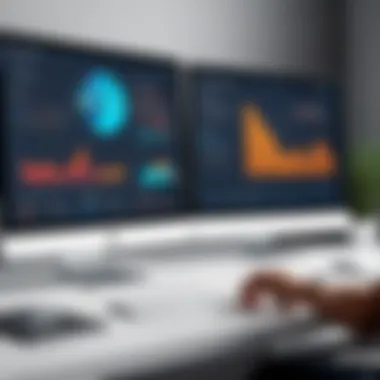
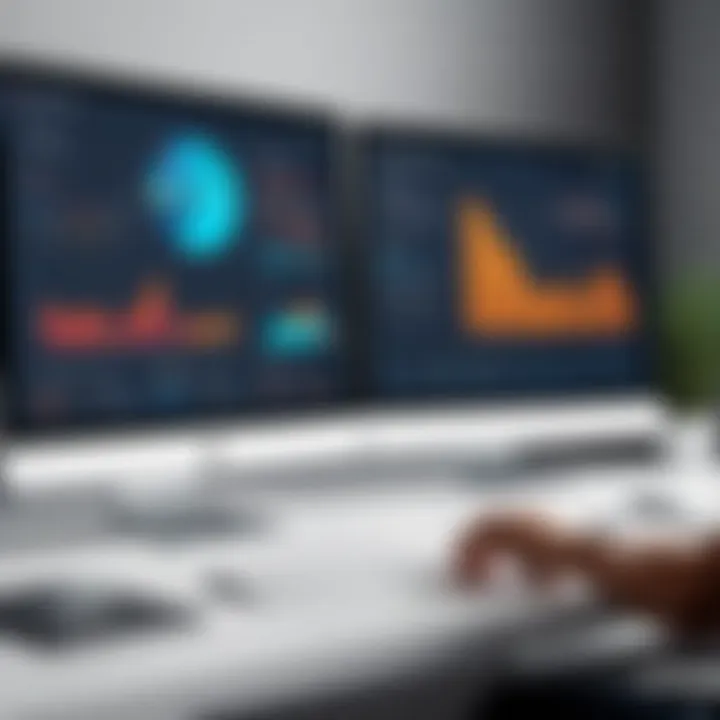
Despite these benefits, business intelligence tools often require some level of training. Small businesses need to evaluate their capacity to adopt these tools effectively.
Statistical Software
For more complex data analysis, statistical software becomes essential. Programs like R and SAS allow for in-depth statistical analysis, regression modeling, and advanced visualization techniques.
Importance of Statistical Software:
- Suitable for applying rigorous statistical methods that can unveil deeper insights.
- Enables predictive analytics, which can forecast trends based on existing data.
- Helps in data cleaning and preparation through advanced functionalities.
However, the use of statistical software can be daunting for those without a background in statistics. As such, small businesses may need support in terms of training or consulting to utilize these tools effectively.
In summary, the tools chosen for data analysis play a fundamental role in the ability of small businesses to leverage data effectively. By understanding the strengths and constraints of spreadsheet, business intelligence, and statistical software, businesses can better position themselves to harness data for growth.
Best Practices for Small Businesses
In the realm of data analysis, adopting best practices is crucial for small businesses aiming to harness the full potential of their data. These practices not only enhance accuracy but also guide decision-making processes and drive growth. Understanding and implementing these principles allows small businesses to extract valuable insights from data, thus enabling them to make informed choices. Additionally, best practices foster a consistent approach, making it easier for teams to collaborate effectively.
Establish Clear Objectives
Setting clear objectives is the cornerstone of effective data analysis. Small businesses must define what they hope to achieve through data-driven strategies. This may involve increasing revenue, improving customer satisfaction, or streamlining operations. Without well-defined goals, data analysis can become directionless.
For instance, a retail business may want to boost sales during a specific period. By establishing this objective, they can focus on analyzing sales data, customer preferences, and market trends related to that timeframe. With a clear target, data collection becomes purposeful, and analysis can provide actionable insights.
Foster a Data-Driven Culture
Cultivating a data-driven culture within a small business is essential. This entails encouraging all employees to leverage data in their daily operations. When staff members recognize the importance of data analysis, they are more likely to use insights to inform their decisions and strategies.
Training sessions can help employees understand how to interpret data effectively. Small businesses might consider utilizing software solutions like Tableau or Microsoft Power BI. These tools present data in an understandable manner and promote a culture of analysis across departments. With a proactive approach toward data, organizations can respond swiftly to changes in the market or customer behavior.
"A data-driven culture empowers teams to make informed decisions, unlocking potential for innovation and team dynamics."
Ensure Data Privacy and Compliance
Data privacy and compliance are critical aspects every small business must prioritize. As organizations collect and analyze data, they must ensure they are adhering to regulations such as the General Data Protection Regulation (GDPR) and the California Consumer Privacy Act (CCPA). Noncompliance can lead to legal issues and damage to reputation.
Implementing strong data security measures is a fundamental step in this process. Practices should include encrypting sensitive data, conducting regular audits, and training employees on data security protocols. By demonstrating commitment to protecting customer data, small businesses not only comply with legal standards but also build trust with their clients.
Common Challenges Faced
Understanding the common challenges small businesses encounter with data analysis is essential for developing effective strategies. Addressing these issues not only enhances data utilization but also promotes sustainable growth within the organization. Notably, challenges such as resource constraints, data overload, and lack of expertise can impede the data analysis process. Recognizing and overcoming these obstacles is a pragmatic approach that bolsters business decision-making and operational efficiency.
Resource Constraints
Small businesses often operate with limited budgets and personnel. This reality makes it difficult to allocate sufficient resources for data analysis initiatives. Many small businesses may lack the financial capability to invest in advanced analytics tools or hire dedicated data analysts. As a result, they might rely on basic software like Microsoft Excel or free online tools, which may not provide comprehensive insights.
Moreover, time constraints pose an additional challenge. Employees might juggle multiple responsibilities, leaving little time for thorough data analysis. This can lead to rushed decisions based on incomplete information, decreasing overall effectiveness. Therefore, prioritizing data analysis and possibly reallocating resources is crucial for navigating these constraints successfully.
Data Overload
The ability to collect vast amounts of data is an advantage. However, this wealth of information can become overwhelming, particularly for small businesses. Data overload makes it challenging to find meaningful patterns or insights. Employees may struggle to identify what data is relevant and instead become bogged down by excessive information.
To combat this, businesses must develop a focused approach to data collection and analysis. Establishing specific objectives prior to collecting data can provide direction. Furthermore, employing data visualization techniques can simplify complex data sets, allowing teams to grasp essential trends at a glance. Thus, avoiding data overload requires a strategic perspective on data management.
Lack of Expertise
A significant barrier for many small businesses is the lack of expertise in data analysis. Employees may not possess the necessary skills to interpret data meaningfully. This knowledge gap can lead to misinterpretation of results and poor decision-making. As data analysis becomes increasingly important for competitive advantage, upskilling staff or seeking external consultation becomes an urgent necessity.
To address this challenge, small businesses should consider investing in training programs or workshops focused on data literacy. Online courses from platforms like Coursera or Udemy can also offer accessible training options. Collaborating with professionals in the field can enhance a team’s analytical skill set and ensure that the insights drawn from data analysis are accurate and actionable.
"Data analysis is not just about numbers; it's about making informed decisions based on evidence."
Ultimately, recognizing and addressing these common challenges is key to harnessing the full potential of data analysis. Small businesses can enhance their operational strategies and ensure growth by allocating sufficient resources, avoiding information overload, and closing the expertise gap.
The Future of Data Analysis in Small Business
Data analysis is evolving rapidly, bringing new opportunities and challenges for small businesses. As the landscape continues to shift, understanding these developments is essential for harnessing data's potential. The future promises a greater integration of advanced technologies and methodologies that can redefine how organizations make decisions.
Emerging Technologies
Emerging technologies are transforming how small businesses approach data analysis. Big data, machine learning, and cloud computing are at the forefront. Each offers distinct advantages.
Big data allows businesses to analyze massive datasets, revealing insights previously obscured in smaller snapshots. This can help identify trends and patterns in customer behavior, improving product offerings and marketing strategies.
Machine learning enables predictive analytics. This technique leverages historical data to forecast future trends. Small businesses can use these insights to enhance inventory management, adjust marketing efforts, and personalize customer experiences.
Cloud computing plays a crucial role by providing access to powerful analytical tools without the need for extensive hardware. This means that even small enterprises with limited budgets can utilize sophisticated software for data analysis, leveling the playing field with larger competitors.
"Embracing emerging technologies in data analysis is not just an option; it's a necessity for survival in a competitive market."
Integration with AI
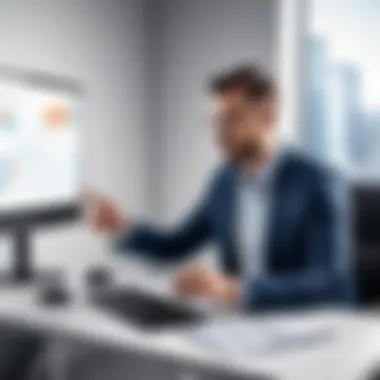
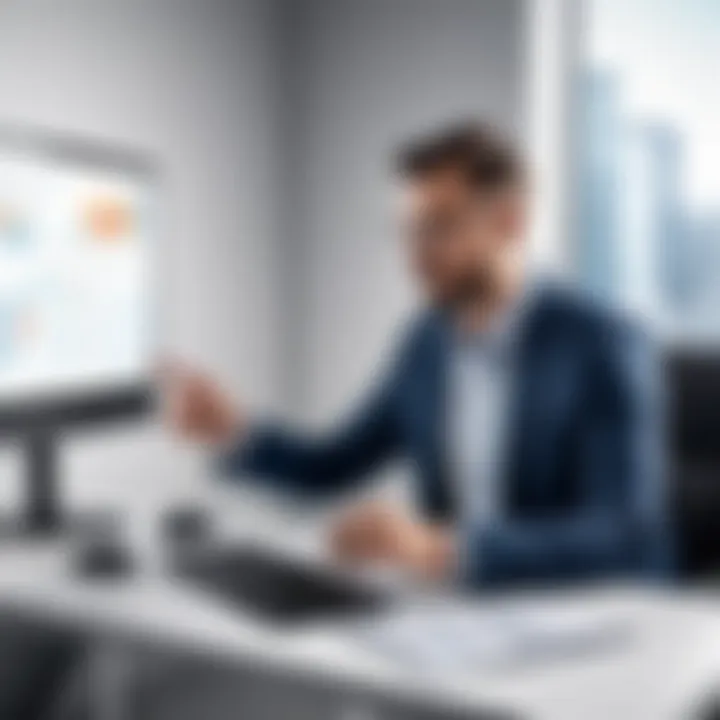
Integrating artificial intelligence into data analysis processes is a game changer for small businesses. AI has the ability to process data at unprecedented speeds, delivering insights that would be inconceivable through manual analysis.
Consider the application of AI in customer relationship management. By analyzing customer data, AI can uncover behavior patterns and preferences. This enables targeted marketing efforts, increasing the likelihood of conversion. AI chatbots can also analyze customer interactions in real time, enhancing customer service and engagement while collecting valuable feedback for further analysis.
However, integrating AI also requires careful consideration. Small businesses need to assess their existing data infrastructure. It is essential to ensure that systems can support AI capabilities. Additionally, training staff to work with AI tools becomes vital. This ensures that the insights derived are actionable and aligned with business goals.
In summary, the future of data analysis in small businesses is bright, driven by technological innovations. By adopting emerging technologies and integrating AI, small enterprises can unlock valuable insights that enhance decision-making and foster growth. The key lies in understanding these advancements and implementing them effectively.
Case Studies of Successful Implementation
Case studies serve as real-world examples that illustrate how data analysis can transform small businesses. By examining specific instances, readers can grasp practical applications and outcomes in different sectors. This section offers insights into successful implementations of data analysis techniques. Understanding these case studies is crucial for small business owners who seek to harness data effectively.
Case Study: Retails
The retail sector provides a plethora of opportunities to utilize data analysis. One example is a small clothing retailer facing declining sales. To combat this, the retailer used data analysis to track customer purchase patterns and inventory levels. Through customer surveys and sales data, the business identified a trend in size preferences and seasonal demands.
The retailer adopted a predictive analysis approach, using statistical models to forecast which styles would sell best in upcoming seasons. They employed demographic data to target specific customer segments with tailored marketing campaigns. Furthermore, by analyzing customer feedback and online reviews, the retailer adjusted its product offerings and improved service quality.
As a result, the business not only increased sales but also improved customer satisfaction. The retailer learned that consistent data monitoring leads to agile decision-making, fostering a more customer-centric approach.
Case Study: Service Providers
Service providers, such as local salons or fitness studios, also can benefit from data analysis. A small gym implemented a data-driven strategy to improve member retention. They gathered data on attendance patterns and member feedback through surveys. By analyzing this data, the gym identified peak usage times and popular classes.
They noticed that many members were dropping out after a few months, which prompted further analysis. The gym found that new members often needed guidance on how to use equipment, leading to frustration and eventual dropout. In response, they established a mentorship program, pairing new members with experienced trainers for initial months.
To measure success, the gym monitored retention rates before and after the program's introduction. Interestingly, they observed a 30% increase in member retention within six months. This case illustrates that data analysis can unveil critical insights driving service enhancements, leading to increased customer loyalty and business stability.
By applying specific data analysis techniques, small businesses can derive valuable insights, enhancing decision-making and operational strategies.
Epilogue
In today’s data-driven world, small businesses cannot afford to overlook the significance of data analysis. The conclusion of this guide emphasizes the countless benefits small enterprises stand to gain by effectively harnessing data. Throughout this article, various aspects were outlined, highlighting how data analysis informs decision-making processes, optimizes operations, and enhances customer insights. By leveraging both quantitative and qualitative data, businesses can make more strategic decisions that drive growth and improve efficiency.
Summary of Key Points
The journey through the landscape of data analysis for small businesses culminates in several key realizations:
- Importance of Data: Data is not just numbers; it is a foundation for informed decision-making.
- Methodologies and Tools: Various techniques and tools, such as statistical software and business intelligence, have their unique advantages and can cater to different business needs.
- Challenges and Solutions: Understanding common challenges like resource constraints or data overload is integral to formulating effective strategies to overcome them.
- Future Trends: Emerging technologies and AI integration will continue to shape data analysis in the future, presenting both opportunities and implications for small businesses.
A summary of these points showcases the multi-dimensional role of data analysis in shaping the future success of small businesses, reaffirming its role as a vital business asset.
Final Thoughts
As small businesses navigate the complexities of the modern market, the insights derived from data analysis will be indispensable. Embracing a data-driven culture will not only enhance decision-making but also foster innovation and resilience. The potential for data analysis to transform operations is immense, yet it requires commitment and a strategic approach.
In concluding, small business owners and stakeholders should prioritize investment in data-related competencies and technologies. By doing so, they position themselves to harness the full power of their data, paving the way for sustainable growth and competitiveness in the years to come.
"Data is the new oil. It's valuable, but if unrefined it cannot really be used."
In short, effective data analysis is not merely an option but a necessity for small businesses aiming to thrive in today's competitive landscape.
Resources for Further Learning
Learning about data analysis is not a one-time event. It requires continuous effort and engagement. This section emphasizes the importance of cultivating a network of resources that can guide small businesses in their data analysis journey. Accessing various learning materials aids not just in fundamental understanding but also in keeping abreast with emerging trends in the field.
Having diverse resources can help professionals in IT-related fields, software developers, and students to deepen their knowledge. They can explore specific areas such as data visualization techniques, statistical methods, or the application of artificial intelligence in data handling. In this manner, one can enhance analytical skills, therefore making more informed decisions in their respective domains.
Here are some specific types of resources that can significantly benefit anyone embarking on this journey:
Books
Books provide a structured way to learn about data analysis. They often contain comprehensive information on various methodologies. Some key titles include:
- "Data Science for Business" by Foster Provost and Tom Fawcett. It explains how data analysis can help companies make better decisions.
- "The Data Warehouse Toolkit" by Ralph Kimball offers insights into data warehousing.
- "Naked Statistics" by Charles Wheelan introduces statistical concepts in an accessible manner.
Reading these resources can empower small business owners to grasp foundational concepts, enhancing their analytical toolkit.
Online Courses
Online courses serve as an excellent medium for practical application of data analysis skills. They often provide hands-on experience. Popular platforms like Coursera, edX, and Udacity offer numerous courses suited for varied skill levels. Some noteworthy choices include:
- Coursera's "Data Analysis and Visualization with Python" helps learners utilize Python for data input and output.
- edX offers "Data Science MicroMasters" programs that delve deeper into statistical thinking and data mining.
- Udacity has its 'Data Analyst Nanodegree', where participants engage in real-world projects.
These courses help learners progress at their own pace while acquiring valuable skills relevant to their careers.
Webinars and Workshops
Webinars and workshops provide arrangement for direct interaction with experts. They often cover current trends, new tools, and real-world case studies. Participating in such events can be beneficial, as they allow for real-time queries and discussions. Many organizations frequently host webinars on data analysis:
- LinkedIn Learning provides workshops focusing on practical applications of data analysis tools.
- Google occasionally holds webinars on using their analytics tools effectively.
- Local universities might offer workshops that feature industry specialists.
Engagement in these activities fosters a deeper understanding and often sparks innovative ideas applicable in specific business contexts.
"Staying engaged with continuous learning opportunities ensures that you are never left behind in an evolving landscape."
Overall, investing time in resources for learning can equip small business owners, IT professionals, and students with the knowledge needed to leverage data analysis effectively. This, in turn, contributes to making informed decisions that can propel their business or projects forward.