Exploring DataRobot Machine Learning: A Comprehensive Analysis
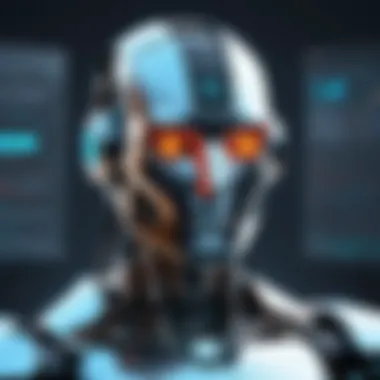
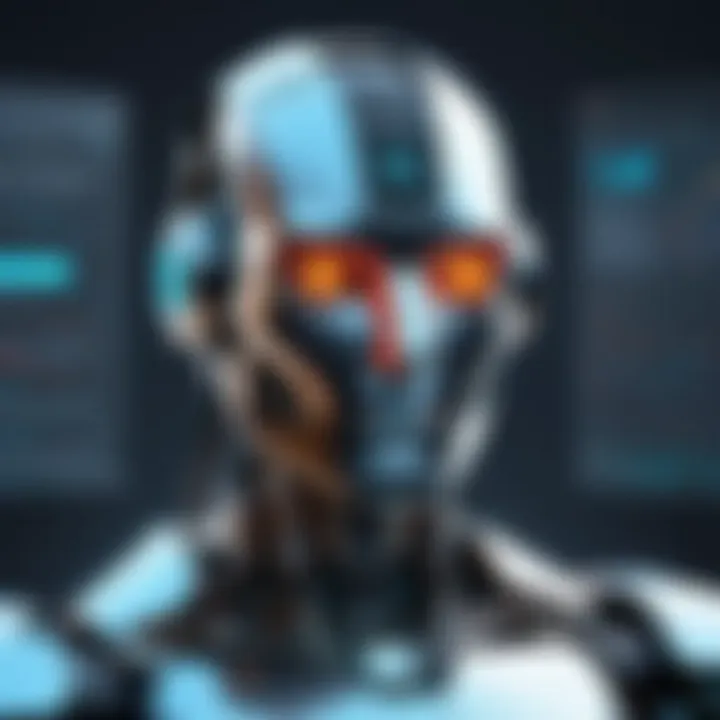
Intro
In the evolving landscape of machine learning, platforms like DataRobot play a crucial role in enhancing productivity and accuracy. This analysis aims to dissect the functionalities and advantages of DataRobot, providing insightful commentary for software developers, IT professionals, and students.
Software Overview
DataRobot presents an innovative solution for automating machine learning tasks. It caters to various sectors, facilitating data-driven decision making with ease.
Purpose and function of the software
The core purpose of DataRobot is clear: it simplifies machine learning processes for users through automation. Organizations benefit from fast model development and deployment. Data scientists can concentrate on higher-level tasks, while the platform handles intricate workflows and modeling steps, thus removing much of the manual effort traditionally associated with machine learning.
Key features and benefits
DataRobot is equipped with an array of powerful features designed to maximize efficiency and effectiveness:
- Automated Machine Learning: Automatically identifies the best models and algorithms for the dataset.
- User-Friendly Interface: A well-designed interface that aids users at different skill levels.
- Deployment Capabilities: Empowers users to deploy their models seamlessly into production.
- Integration Options: Works smoothly with various data sources and existing workflows, enhancing usability.
- Comprehensive Documentation: Offers extensive resources for users, facilitating a smoother learning curve.
The benefits of utilizing DataRobot are substantial. Speed and accuracy in model development are improved, leading to better insights and decision making.
"DataRobot transforms the way organizations think about and implement machine learning, reducing time spent on development while increasing the quality of results."
Installation and Setup
Setting up DataRobot is a straightforward procedure, but it does have specific requirements and steps necessary for optimal performance.
System requirements
To ensure proper functionality, users must fulfill certain system requirements:
- Minimum RAM: 16GB recommended
- CPU: 4 cores minimum
- Supported Operating Systems: Windows, Linux
- Internet connection for accessing cloud functionality and updates
Installation process
The installation process is user-friendly. Following these steps will facilitate a successful setup:
- Download DataRobot: Visit the official DataRobot website to acquire the installation file.
- Install the Software: Run the installer and follow the on-screen instructions.
- Initialize the Software: Open DataRobot and complete the initial setup, including user account creation and configuration settings.
- Verify Functionality: Before diving into projects, run a test to ensure everything is functioning as intended.
Understanding the installation and setup process is key to utilizing DataRobot effectively. With proper preparation, users can harness the software’s capabilities over a wide range of applications.
Prologue to DataRobot
Understanding DataRobot is crucial in the landscape of modern machine learning. As organizations increasingly rely on data-driven decision making, having a powerful platform like DataRobot facilitates and accelerates machine learning processes. This section will define what DataRobot is and its primary purpose, followed by a discussion on the evolution of machine learning platforms, highlighting how DataRobot fits into this changing environment.
Definition and Purpose
DataRobot is an automated machine learning platform that empowers users to build and deploy predictive models with minimal manual intervention. The platform aims to democratize access to machine learning by simplifying complex algorithms and making them available to a wider audience, including those who may not have a strong background in coding or data science. By providing tools for data preparation, model selection, and performance evaluation, DataRobot allows organizations to quickly analyze their datasets and generate insights that drive strategy and operational efficiency.
A salient feature of DataRobot is its commitment to improving accessibility to machine learning. It achieves this through a user-friendly interface and automated workflows. The intent is to enable stakeholders from various sectors—ranging from healthcare to finance—to utilize machine learning capabilities without needing extensive expertise in data science. This is vital for harnessing the full potential of data within organizations and aids in making informed decisions based on predictive analytics.
The Evolution of Machine Learning Platforms
Historically, the journey of machine learning has been marked by significant advancements in technology and methodology. Initially, machine learning required specialized knowledge and manual tuning of algorithms, often making it inaccessible for average users. As the demand for data-driven solutions surged, the need for more user-friendly platforms became apparent.
In response, platforms like DataRobot emerged, addressing a gap in the market. Early systems were labor-intensive and relied heavily on manual processes that limited efficiency. The development of automated machine learning—often referred to as AutoML—represents a paradigm shift, allowing users to streamline the model-building process significantly.
The evolution from foundational machine learning practices to sophisticated platforms like DataRobot highlights the ongoing transformation in technology. Not only do these platforms boast improved performance and speed, but they also incorporate diverse algorithms, making them adaptable for various business needs. Such developments underscore the importance of DataRobot in helping users navigate the complexities of machine learning.
DataRobot symbolizes a turning point in machine learning, merging automation with user empowerment, ultimately turning data into valuable insights.
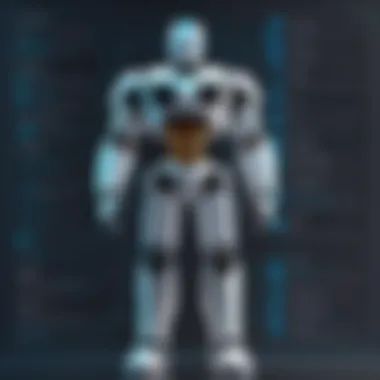
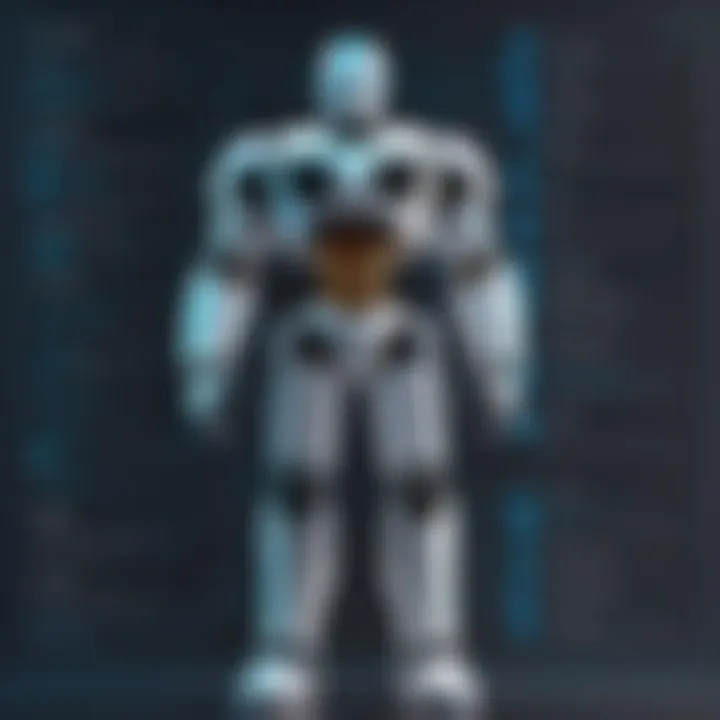
Key Features of DataRobot
DataRobot stands out as a leading machine learning platform due to several key features that enhance its utility across various sectors. Understanding these features provides insight into how organizations can leverage DataRobot to improve their data-driven decision-making processes. The importance of these features cannot be understated, as they cater to both novice users and experienced data scientists, making machine learning accessible to a wider audience.
AutoML Capabilities
One of the hallmark features of DataRobot is its AutoML capabilities. This functionality automates the machine learning workflow, which simplifies the model-building process. Users can easily input their datasets, and DataRobot will take over from there. It automatically selects appropriate algorithms, tunes hyperparameters, and conducts model evaluations. This is not only efficient but also reduces the time spent on mundane tasks. For professionals in the IT field, this feature is especially advantageous as it allows for the rapid deployment of models, enabling teams to focus on more strategic initiatives.
"DataRobot’s AutoML capabilities enable teams to construct complex models without deep expertise in data science."
Support for Multiple Algorithms
DataRobot supports a diverse array of algorithms, catering to various use cases and problems. This variety includes regression, classification, and time-series forecasting models. The platform allows users to compare different algorithms to determine which yields the best performance for their specific dataset. It also implements ensemble methods to leverage the strengths of multiple models, increasing predictive power. For developers and analysts alike, this flexibility means they do not have to limit themselves to just one approach. They can explore numerous options and utilize the best tools for their unique challenges.
User-Friendly Interface
The user interface of DataRobot is designed with intuitiveness in mind. Its clean layout and easy navigation contribute to an efficient user experience. Users, even with minimal technical background, can access critical functionalities without feeling overwhelmed. The visualizations provided help in understanding model performance and predictions, which is crucial for explanations to stakeholders who may not have deep technical knowledge. Ultimately, the user-friendly interface can facilitate greater adoption within organizations, streamlining the transition to data-driven methodologies across different levels of expertise.
Applications of DataRobot
The application of DataRobot is pivotal in harnessing the capabilities of machine learning across various industries. By automating the modeling process, organizations can achieve faster insights and data-driven solutions. The relevance of understanding DataRobot's applications lies in its ability to transform how businesses and sectors utilize their data. This section explores three major domains where DataRobot provides significant impact: business intelligence, healthcare solutions, and innovations within the financial sector.
Business Intelligence
In the realm of business intelligence, DataRobot enables companies to sift through vast amounts of data effectively. The platform supports the analysis of patterns and trends that inform strategic decisions. For instance, DataRobot can help organizations predict customer buying behavior, optimizing marketing strategies accordingly.
- Data-driven decision-making: By leveraging predictive analytics, businesses can anticipate future trends based on historical data.
- Enhanced reporting: Automated reporting tools streamline the generation of insights and dashboards, reducing manual effort and time.
- Real-time analytics: Integration with live data feeds ensures that businesses are making decisions based on the most current information.
In summary, DataRobot aids in crafting a more responsive organization prepared to embrace change based on analytical foresight.
Healthcare Solutions
Healthcare is a field where the implications of machine learning can be profound. DataRobot’s applications here are manifold and crucial. The use of predictive models can enhance patient care and operational efficiency.
- Patient outcome predictions: Using DataRobot, healthcare providers can model patient data to forecast outcomes, enabling timely interventions.
- Resource management: The platform can optimize resource allocation by predicting patient volumes, thus improving service delivery.
- Personalized medicine: By analyzing diverse data sets, including genetic information, healthcare professionals can tailor treatments to individual patient profiles.
Overall, DataRobot empowers healthcare organizations to make informed, data-driven decisions that can lead to better patient outcomes and efficient operations.
Financial Sector Innovations
Financial services benefit greatly from DataRobot’s machine learning capabilities. The sector requires robust risk assessment tools for fraud detection, credit scoring, and market analysis.
- Fraud detection: Models created in DataRobot can analyze transaction patterns to identify anomalies, potentially flagging fraudulent activity quickly.
- Risk assessment: Banks and financial institutions utilize predictive analytics to assess the creditworthiness of customers based on historical data.
- Investment forecasting: DataRobot allows financial analysts to leverage historical trends to make informed predictions about stock movements and market changes.
Thus, by integrating DataRobot into their frameworks, companies in the financial sector can enhance decision-making processes while minimizing risks associated with economic fluctuations.
DataRobot is a transformative force in various industries, driving forward the capabilities of machine learning to improve operational efficiency and decision-making.
Integrating DataRobot into Existing Workflows
Integrating DataRobot into existing workflows is essential for organizations looking to leverage machine learning without disrupting established processes. This integration can unlock significant efficiencies and improve decision-making across teams. By considering specific elements and benefits, companies can better understand how to optimize their use of DataRobot within their operational landscape.
Compatibility with Major Data Sources
One of the foremost strengths of DataRobot is its compatibility with various data sources. This flexibility allows users to connect seamlessly to platforms such as Amazon S3, Microsoft Azure, and Google Cloud Storage. A straightforward integration means less time spent on data migration and more time focusing on analysis. Handling structured and unstructured data efficiently helps in obtaining more comprehensive insights.
The ability to utilize databases like PostgreSQL and MySQL also promotes a more robust data processing environment. Using these compatibility features ensures that any data available to the organization can be tapped into for analysis and model building. This maximizes the potential of the existing data, transforming it into valuable insights.
APIs and Customization
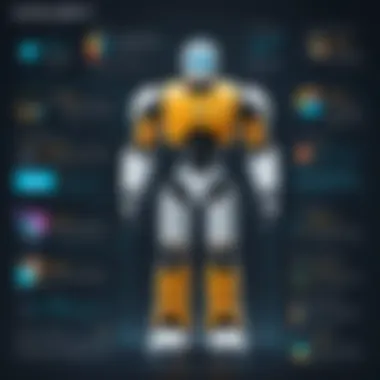
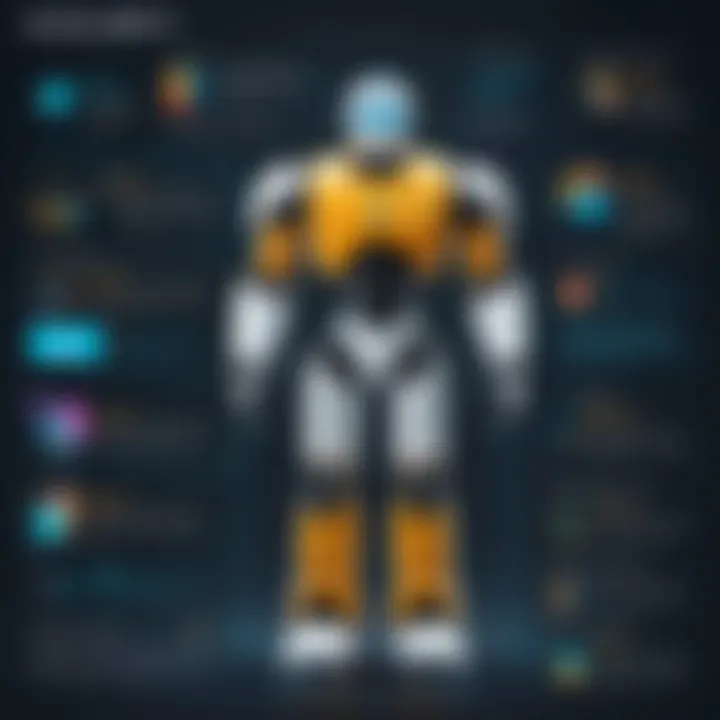
DataRobot provides a well-documented API that enhances its usability for developers. This API enables customization and automation of various tasks. Organizations can adapt DataRobot functionalities to meet distinct project needs, reducing repetitive actions and enhancing productivity.
Through the API, it is possible to automate model training, evaluation, and deployment workflows. By creating tailored solutions that reflect specific business requirements, companies can address unique challenges more effectively. Custom scripts can simplify complex processes and help integrate machine learning into broader IT ecosystems, fostering better communication between systems.
Collaboration Tools
Collaboration is key when employing technologies like DataRobot. Various built-in collaboration tools offered by DataRobot facilitate team interactions. Users can share models, documents, and results within the platform, allowing for a streamlined approach toward project collaboration.
Additionally, DataRobot supports features like commenting and tagging, which encourage real-time feedback and discussions on model iterations. These capabilities enhance collective decision-making and lead to higher-quality outputs. Teams can thus align their strategies, objectives, and insights more effectively, which is crucial in fast-paced environments.
Integrating DataRobot into existing workflows does not just enhance the current processes; it opens doors for innovation and promotes a culture of data-driven decision-making. By considering compatibility, customization through APIs, and effective collaboration tools, organizations can maximize the advantages that DataRobot brings to their operations.
Benefits of Using DataRobot
DataRobot provides significant advantages for businesses and individuals navigating the world of machine learning. It simplifies complex processes and drives operational efficiencies. Knowing the benefits allows organizations to make informed decisions about adopting this technology. Here are three key benefits that stand out.
Efficiency and Speed
In the realm of machine learning, time is of the essence. DataRobot excels in efficiency by automating numerous tasks traditionally performed manually. Data preparation, model selection, and hyperparameter tuning can consume substantial time, diverting resources from strategic objectives. By streamlining these processes, DataRobot reduces project timelines significantly. Users can focus on higher-order tasks rather than getting bogged down in minutiae. This efficiency is particularly crucial in fast-paced environments where decisions need to be made rapidly to stay competitive.
Benefits of DataRobot’s efficiency include:
- Reduced model training time
- Less human intervention required
- Scalability for handling large datasets
Enhanced Predictive Accuracy
Predictive analytics is a cornerstone of data-driven decision making. With DataRobot, users gain access to a suite of advanced algorithms and models. The platform automatically selects the most suitable model for the given dataset, optimizing for accuracy. This capability minimizes the subjective biases that often come into play when selecting machine learning models. Consequently, organizations achieve higher levels of predictive accuracy, leading to better informed business strategies and decisions.
The focus on accuracy can be summarized as follows:
- Automatic algorithm selection
- Continuous learning and model refinement
- In-depth analysis of model performance
Cost-Effectiveness
Investing in machine learning can be daunting, particularly for small to mid-sized companies. DataRobot offsets this concern by providing a cost-effective solution. The platform reduces the need for expensive infrastructure or extensive in-house data science teams. By enabling teams to generate insights more quickly and efficiently, organizations can realize a faster return on investment. Moreover, the predictive capabilities reduce costly errors that might arise from poor decision-making.
Some key considerations include:
- Lower operational costs
- Reduced dependency on external consultants
- Improved resource allocation
"By leveraging DataRobot, organizations not only enhance productivity but also unlock new possibilities in predictive analytics and decision-making efficiency."
In summary, the benefits of using DataRobot extend beyond mere technology adoption. They play a crucial role in strategic development and operational enhancement.
Challenges and Limitations of DataRobot
Understanding the challenges and limitations of DataRobot is crucial for users to maximize the platform's potential and make informed decisions. While DataRobot provides numerous advantages in automating machine learning tasks, awareness of these challenges can help in mitigating risks and ensuring more effective application of its tools.
Data Privacy Concerns
Data privacy is a significant issue for organizations utilizing DataRobot. As companies integrate various data sources into their workflows, the protection of sensitive information becomes paramount. When personal data is involved, particularly in sectors like healthcare and finance, regulatory compliance (such as GDPR or HIPAA) must be a top priority. Users must ensure that they handle data responsibly, employing robust encryption methods and access controls. Any breach of data privacy can lead to legal implications and reputational damage. Moreover, as DataRobot relies on cloud computing for many of its services, users need to be vigilant about potential vulnerabilities associated with third-party data storage and processing.
Model Interpretability Issues
Machine learning models produced by DataRobot often function as black boxes, making it challenging for users to understand how predictions are made. This lack of transparency can lead to skepticism regarding model outcomes, especially in high-stakes environments like healthcare or criminal justice. Furthermore, stakeholders may require explanations for predictions to trust and accept the results. Thus, the interpretability of models is critical for fostering user confidence and ensuring fairness in decision-making processes. DataRobot provides tools for model explanations, but they require users to be proactive in seeking out these insights and learning how to leverage them effectively.
Dependency on Quality of Input Data
The performance of any machine learning model, including those developed with DataRobot, is heavily dependent on the quality of the input data. Poor quality data can result in inaccurate predictions, undermining the entire model's utility. Issues such as missing values, biases, and inaccuracies in the dataset can skew results significantly. To combat this, users must commit to thorough data preprocessing and validation techniques before inputting data into the system. Regular audits of data sources are advised to ensure consistency and reliability.
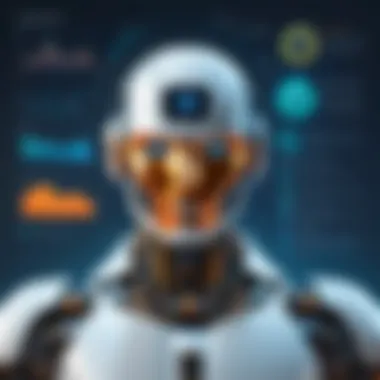
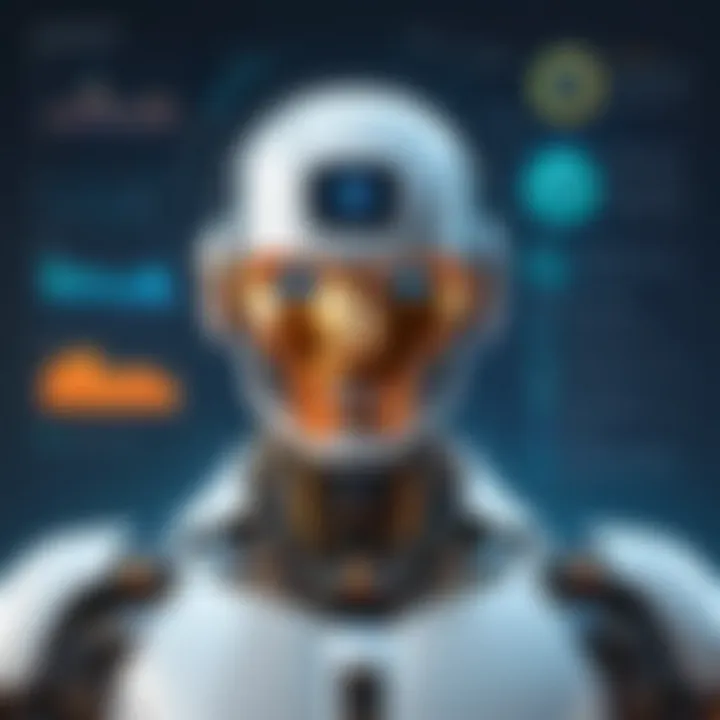
Best Practices for Utilizing DataRobot
The efficient use of DataRobot's machine learning capabilities hinges on adopting best practices that maximize its potential. Implementing these practices not only streamlines processes but also enhances the overall reliability of predictive models. Specialists and organizations that engage with DataRobot should recognize that steady adherence to best practices can significantly influence their outcomes, making it essential to explore effective strategies.
Data Preparation Strategies
Data preparation serves as the foundation of any successful machine learning project, especially when working with platforms like DataRobot. Proper data management encompasses several steps to ensure data quality, completeness, and relevance. Here are vital strategies for effective data preparation:
- Data Cleaning: It is crucial to identify and rectify errors in the dataset. This involves handling missing values, correcting discrepancies, and filtering out outliers that could skew results.
- Feature Selection: Selecting the right features can greatly impact model performance. Users should leverage domain knowledge to find the most relevant variables that contribute to the prediction task.
- Data Transformation: Transforming variables to fit a specific distribution can enhance model accuracy. Techniques such as normalization and encoding categorical data often assist in preparing data for analysis.
- Data Augmentation: In specific fields like image processing, augmenting the dataset can lead to improved model robustness. Techniques could include image rotation, scaling, or adding noise to training samples.
By investing time into these strategies, users can achieve a higher quality outcome and facilitate the learning process of their selected algorithms within DataRobot.
Iterative Testing and Validation
Iterative testing and validation play a vital role in ensuring that the machine learning models produced by DataRobot are both reliable and effective. Continuous evaluation improves adaptability and fine-tunes models based on real-time feedback. Some considerations include:
- Cross-Validation: Employ cross-validation techniques to assess model performance. This allows users to maximize their dataset by training on different segments and understanding variability within the data.
- Hyperparameter Tuning: Adjusting hyperparameters is crucial for optimizing model performance. Utilize DataRobot’s features to experiment with different configurations efficiently.
- Monitoring Metrics: Establish a clear set of metrics to evaluate success. Track metrics such as accuracy, precision, recall, and F1 score to understand model efficacy during different phases of testing.
- Feedback Loops: Create feedback loops to refine models based on new data and performance insights. This ensures models remain relevant and effective as conditions change over time.
Incorporating these best practices fosters a disciplined approach to using DataRobot, balancing thoroughness with adaptability. This continual improvement not only enhances predictions but also helps leverage machine learning in various applications.
Future Trends in Machine Learning and DataRobot
The landscape of machine learning is continuously evolving. Understanding future trends is essential for users of platforms like DataRobot. These trends shape how individuals and organizations can leverage machine learning to meet emerging demands. Exploring these trends provides insights into advancements that will drive success in data-driven environments. It also prepares users for challenges that innovation may bring. This section focuses on critical trends such as automation technologies and ethical AI. Both hold significant potential for influencing how DataRobot is utilized in years to come.
Emerging Technologies in Automation
Automation has advanced rapidly, impacting how machine learning is applied. New technologies enhance efficiency in various industries. Tools like robotic process automation (RPA) are making it easier to integrate machine learning in everyday operations. By combining RPA with DataRobot, organizations can minimize manual work while enhancing predictive capabilities. This efficiency is vital in businesses where time and accuracy are essential.
The following technologies will shape automation in the machine learning domain:
- Natural Language Processing (NLP): NLP allows systems to understand human language. This is critical for developing chatbots and customer service solutions.
- Automated Machine Learning (AutoML): As DataRobot excels in AutoML, it continues to provide an interface that reduces complexity. Those new to machine learning can utilize it without deep expertise.
- Edge Computing: Processing data at the edge of the network speeds up decision-making. This technology is increasingly paired with IoT devices and machine learning models to improve performance.
"Automation not only streamlines processes but also opens doors for innovation."
These emerging technologies indicate a shift towards a more automated and integrated approach in business operations.
Trends in Ethical AI
As machine learning applications proliferate, ethical considerations become crucial. With DataRobot's power comes responsibility in how predictive models are used. Ethical AI ensures that technology serves humanity positively. This includes addressing bias, ensuring transparency, and promoting accountability.
Key areas of focus in ethical AI include:
- Bias Mitigation: Machine learning models often reflect pre-existing biases in data. Users must be vigilant and adopt practices that address these biases.
- Transparency: Understanding how models make decisions is essential. DataRobot provides tools to explain decisions and the factors influencing them, leading to trust.
- Accountability: Organizations must establish who is responsible for the ethical use of AI models. This responsibility is paramount as AI begins to influence critical decisions in various sectors.
Ensuring ethical usage of machine learning platforms like DataRobot will build more robust systems. Ultimately, the focus on ethical AI leads to responsible innovation that benefits society as a whole.
Finale
The conclusion serves as an essential element in this article, providing a comprehensive wrap-up of the discussions on DataRobot's machine learning capabilities. It synthesizes the key points and insights shared, allowing readers to internalize the complex information more effectively. Understanding the role of DataRobot in automating machine learning tasks is vital for professionals seeking to enhance their data-driven decision-making process.
Summary of Key Insights
Throughout the article, several critical facets of DataRobot have been analyzed. The platform’s AutoML capabilities streamline the machine learning workflow, minimizing the manual effort typically involved. Users benefit from its support for multiple algorithms, which allows more significant flexibility in their analytical approaches. Furthermore, the user-friendly interface reduces the barriers to entry for non-specialists in data science.
Another point of emphasis is the platform's practical applications across diverse sectors such as business intelligence, healthcare, and finance. Specific case studies illustrate how DataRobot enables enhanced predictive accuracy and operational efficiency.
Additionally, while there are challenges like data privacy concerns and model interpretability issues, the overall benefits of utilizing DataRobot cannot be overstated.
The Path Forward for DataRobot Users
As DataRobot continues to evolve, users must stay informed of the latest features and trends in machine learning. The prsent environment of rapid technological advancement necessitates a proactive approach. Users can enhance their proficiency with DataRobot by embracing best practices in data preparation and iterative testing.
Adapting to the changing landscape involves approaching machine learning not just as a tool, but as a journey of discovery. Being aware of ethical considerations will be crucial as well, particularly in ensuring the responsible use of AI technologies. This moral compass will guide users in making decisions that not only benefit their organizations but also align with societal expectations.
Ultimately, the insights gained from this article equip DataRobot users with the foundational knowledge needed to leverage the platform. By applying these insights future-oriented strategies, users can effectively integrate machine learning into their operations for continued success.