Exploring IBM Data and Analytics for Insightful Decisions
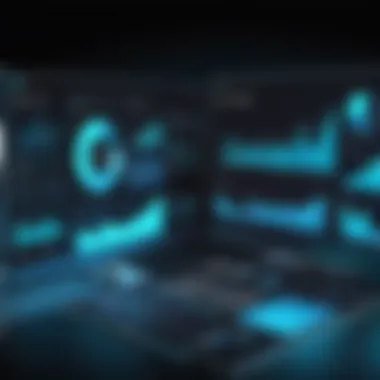
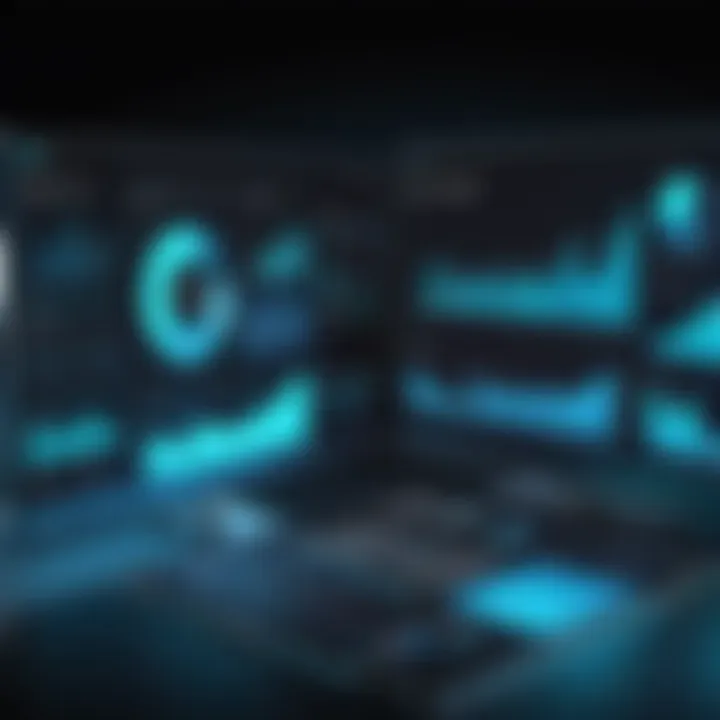
Intro
In today's data-driven world, organizations increasingly rely on advanced analytics tools to extract meaningful insights from large volumes of data. Within this landscape, IBM offers a robust suite of data and analytics solutions that empower businesses to turn raw information into strategic advantages. This exploration delves into the essential features and applications of IBM's offerings, while also addressing the pressing issues of data integrity and security in analytics.
Software Overview
IBM’s data and analytics software serves a crucial role in guiding organizations through their data journey. Its key purpose is to facilitate transformative data analysis, enabling businesses to make informed decisions more effectively. IBM provides various tools and platforms, each tailored to specific analytical needs and challenges.
Purpose and Function of the Software
The primary function of IBM's data and analytics solutions is to simplify and accelerate the extraction of insights from data. By employing machine learning algorithms, natural language processing, and advanced data visualization, the software transforms complex data sets into user-friendly dashboards and reports. This function is vital for organizations aiming to harness data to drive efficiency and innovation.
Key Features and Benefits
IBM's offerings come packed with a range of features designed for precision and usability. Some of the key features include:
- Data Integration: Seamless connectivity with various data sources.
- Predictive Analytics: Forecasting capabilities that help businesses anticipate trends.
- Real-Time Analytics: Instantaneous data processing for timely decision-making.
- Data Governance: Tools to ensure compliance and data quality.
- Collaboration Features: Facilitate team communication and sharing of insights.
The benefits of utilizing IBM's solutions are numerous. Enhanced decision-making, improved operational efficiency, and increased competitive advantage are among the most significant gains.
Installation and Setup
Getting started with IBM’s data and analytics tools requires an understanding of the system’s prerequisites and the installation procedure. Here, we outline the essential considerations to effectively set up the software to maximize its potential.
System Requirements
Before installation, it's critical to assess whether the system meets the required specifications. Key requirements often include:
- Operating System: Windows Server 2016 or newer, Linux distributions, or IBM Cloud environments.
- Processor: Dual-core or higher recommended.
- Memory: Minimum of 8 GB RAM, with more for extensive data processing.
- Storage: Sufficient disk space, ideally SSD drives, for optimal performance.
Installation Process
Installing IBM’s software typically follows a straightforward series of steps:
- Download the latest version from the official IBM site.
- Follow the installation wizard, which guides through configuration options.
- Configure database connections as required.
- Verify installation by launching sample reports to ensure everything is functioning properly.
By completing these steps, users can ensure a smooth transition into leveraging IBM's data and analytics capabilities.
"Data is the new oil, but like oil, it must be refined to be useful." - Clive Humby
In summary, IBM's data and analytics solutions represent a culmination of advanced technology and practical application. With proper setup, organizations can unlock the full potential of their data to support informed decision-making.
Preamble to Data Analytics
Data analytics has become crucial in today's data-driven landscape. Companies and organizations generate vast amounts of data daily. Understanding this data helps them in making informed decisions. In this section, we will explore the fundamental aspects of data analytics and its role in driving business strategies.
Definition and Importance of Data Analytics
Data analytics refers to the process of examining and interpreting raw data to extract valuable insights. It encompasses various techniques and tools used to analyze data sets. The importance of data analytics lies in its ability to reveal patterns and trends that inform business decisions. For instance, business intelligence tools allow for efficient reporting and data visualization, helping organizations understand their performance metrics clearly.
Organizations that embrace data analytics are often more competitive. They can identify market trends, optimize operations, and tailor services to meet customer needs. Moreover, by leveraging predictive analytics, companies can forecast future outcomes based on historical data. This can significantly enhance strategic planning and operational efficiency, ensuring better resource allocation.
In addition, data analytics promotes a culture of evidence-based decision-making. Leaders equipped with relevant data make choices that are less reliant on intuition and more on factual analysis. This shift not only enhances accountability but also fosters a more agile organizational structure capable of adapting to changing market conditions.
Historical Context: Evolution of Data Analytics
The journey of data analytics goes back several decades. Initially, organizations relied on simple statistical methods and manual calculations. As computational technology advanced, so did the ability to process and analyse larger data sets. By the 1970s, businesses began utilizing database management systems, laying the groundwork for the analytical practices we see today.
The introduction of business intelligence in the 1990s marked a significant evolution. Companies began to use software to analyze complex data more comprehensively. This era saw the creation of myriad tools dedicated to data warehousing and reporting. Fast forward to the late 2000s, the rise of big data turned data analytics into a mainstream practice. Novel technologies emerged, allowing organizations to process unstructured data, thus expanding analytical capabilities even further.
Now, with the advent of AI and machine learning, the field is continually evolving. Modern analytics involves integrating these technologies to provide deeper insights and more sophisticated forecasting. The potential for data analytics at this stage is vast and primarily focused on empowering organizations to transform information into actionable strategies.
"Data analytics is not just about numbers; it is about leveraging insights to enhance decision-making."
In summary, understanding data analytics from historical and practical perspectives emphasizes its growing significance in today’s industries. The evolution reflects an ongoing journey toward enhanced decision-making supported by data.
Overview of IBM's Data and Analytics Offerings
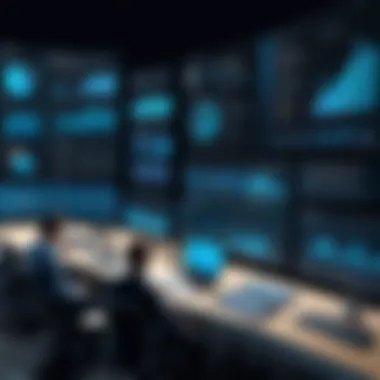
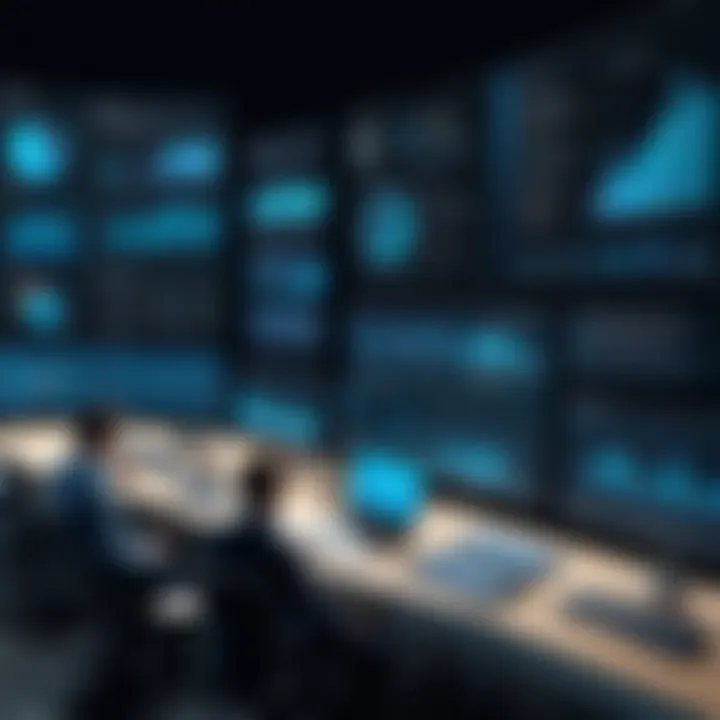
Understanding IBM's data and analytics offerings is crucial for organizations aiming to leverage their data effectively. IBM provides a range of solutions that not only facilitate data analysis but also enhance how organizations make informed decisions. The offerings include tools that integrate various data sources, apply sophisticated analytics, and ensure data security and integrity. This holistic approach allows businesses to convert raw data into valuable insights, positioning them to stay competitive in their respective markets.
IBM Business Intelligence Tools
IBM’s business intelligence tools are designed to help organizations transform data into actionable insights. These tools provide visualization, reporting, and analysis features. They allow users to create interactive dashboards that showcase key metrics and trends. IBM Cognos Analytics is a notable tool in this category. It empowers users to visualize data through a self-service interface, which promotes independence in data exploration.
Key benefits include:
- User-Friendly Interfaces: Simplifies the process of data visualization for non-technical users.
- Comprehensive Reporting: Facilitates detailed reports that can be customized according to the needs of the business.
- Data Integration: Seamlessly combines data from various sources, enabling a more holistic view of operations.
IBM Watson Analytics: A Study in AI
IBM Watson Analytics harnesses the power of artificial intelligence to further enhance data analysis capabilities. This tool uses natural language processing to interpret user queries, making data analysis more intuitive. Users can ask questions in plain English and receive insights with minimal effort. This democratizes access to analytics, allowing even those without a technical background to derive insights from complex datasets.
Some highlights of Watson Analytics include:
- Predictive Analytics: Identifies potential future trends by analyzing historical data.
- Automated Insights: Generates insights automatically, saving users time and effort.
- Visual Storytelling: Presents data in visually engaging formats, fostering easier comprehension of findings.
IBM Cloud Pak for Data
IBM Cloud Pak for Data provides an integrated data and AI platform that simplifies data collection, organization, analysis, and modeling. This offering is versatile and supports hybrid multi-cloud environments. Organizations can run their analytics anywhere, whether on local servers or cloud infrastructures. Cloud Pak for Data aims to facilitate data ops and AI lifecycle governance, ensuring organizations can manage their data efficiently.
Benefits include:
- Unified Data Governance: Centralizes data management and ensures compliance with regulations.
- Scalability: Can grow with the organization’s data needs.
- Collaboration Features: Encourages different teams to work together on data-driven projects, enhancing overall productivity.
"With IBM’s offerings, organizations can not only harness insights but also secure a strategic advantage through informed decision-making across various sectors, from healthcare to finance."
The advantages of IBM's data and analytics tools are significant. By utilizing these solutions, businesses can optimize operations, enhance decision-making capabilities, and achieve better outcomes in a rapidly changing environment. This foundational understanding sets the stage for exploring specific applications and trends, which will be addressed in subsequent sections.
Key Features of IBM Analytics Solutions
IBM's Data and Analytics solutions offer a comprehensive suite of features that refine the process of transforming data into valuable insights. These features are essential for organizations aiming to improve their decision-making capabilities. Accurate data governance, advanced analytical functions, and seamless integration with existing systems stand out as pivotal elements that enhance the effectiveness of IBM's offerings. The ability to effectively manage data quality, utilize sophisticated analytics, and integrate with pre-existing infrastructures can lead to more informed strategic choices and operational efficiencies.
Data Governance and Integrity
Data governance refers to the management of data availability, usability, integrity, and security across an organization. IBM places strong emphasis on data governance as a key feature of its analytics solutions. The importance of keeping data accurate and secure cannot be overstated. Effective governance ensures that data is stored properly, used appropriately, and is consistent across all platforms.
With IBM's tools, organizations can establish clear policies and procedures regarding data usage. This lowers the risk of data breaches and enhances compliance with regulatory standards. Moreover, data integrity is crucial for trustworthiness in analytics outcomes. When data is accurate and complete, the insights derived from it can significantly enhance decision-making.
Advanced Analytics Capabilities
IBM's analytics solutions boast advanced capabilities that enable users to perform sophisticated analyses of vast volumes of data. These tools incorporate techniques such as predictive analytics, machine learning, and artificial intelligence to uncover patterns and trends that can be pivotal for business strategy.
- Predictive Analytics: By analyzing past data, predictive analytics provides organizations insights into future trends. This can significantly aid in inventory management, customer engagement, and other core functions.
- Machine Learning: Through machine learning algorithms, the software can learn from past data and adapt its analysis techniques, which leads to increasingly accurate predictions over time.
- AI Integration: The incorporation of AI tools allows for natural language processing and more intuitive data exploration, enriching the data analysis experience.
IBM’s advanced analytics capabilities empower businesses to leverage their data more effectively, leading to enhanced operational efficiencies and more strategic planning.
Seamless Integration with Existing Systems
In today’s technological landscape, companies utilize a variety of systems and platforms. For data analytics tools to be effective, seamless integration with existing systems is vital. IBM ensures that their analytics solutions can easily integrate with other software and infrastructure that organizations already use. This reduces friction during implementation and promotes a unified approach to data management.
Compatibility with systems such as SAP, Salesforce, or Microsoft Azure facilitates data flow between various platforms. Moreover, this kind of integration allows for a holistic view of organizational data, ensuring that insights are derived from all available information. Firms can then establish a more coherent strategy that reflects insights from different data sources.
By focusing on these key features, IBM positions its analytics solutions as valuable assets for organizations aiming to harness the transformative power of data. Through robust governance, advanced analytical capabilities, and straightforward integration with existing systems, businesses can navigate the complexities of data management to drive successful outcomes.
Applications of IBM Data and Analytics Solutions
The application of IBM's data and analytics solutions plays a crucial role in shaping organizational strategies and improving efficiencies. By transforming data into insights, businesses can make more informed decisions that align with their strategic objectives. The solutions offered by IBM are tailored to address distinctive industry needs. Understanding these applications can lead to uncovering opportunities for growth and innovation.
Industry-Specific Use Cases
Healthcare Applications
In healthcare, IBM's analytics tools are essential for improving patient outcomes and operational efficiency. These applications engage with large volumes of patient data, enabling healthcare professionals to identify trends and predict patient needs. One key characteristic of these applications is their ability to provide real-time analytics. This capability is beneficial because it allows for timely interventions which can save lives. Furthermore, IBM Watson Health exemplifies how AI can analyze unstructured data, such as clinical notes and medical images, to provide actionable insights. The unique feature of this area lies in its integration of various data sources to present a holistic view of patient health, though challenges remain concerning data privacy and interoperability.
Financial Services Insights
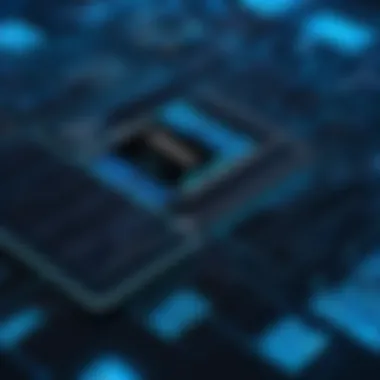
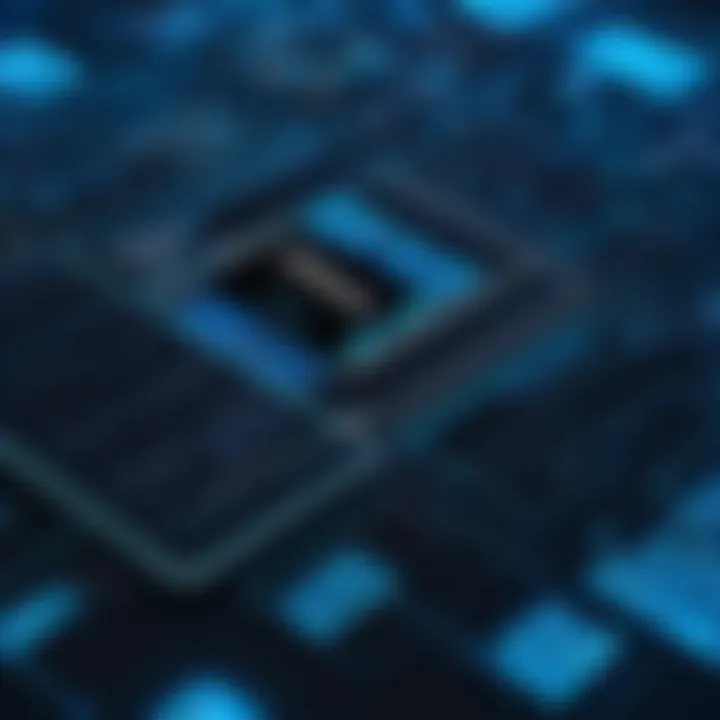
In the financial sector, IBM analytics provides insights that help businesses navigate risks andOptimize their portfolios. The ability to analyze market trends and customer behaviors makes it a vital tool for decision-making. A prominent characteristic of these solutions is their predictive analytics capabilities. This feature allows financial institutions to identify potential issues before they escalate, thus safeguarding assets. One unique aspect of IBM's financial services analytics is its capability to simulate various economic conditions, enabling strategic planning. While these tools provide significant advantages, concerns around data security and regulatory compliance persist, requiring careful management and governance.
Retail Analytics
Retail analytics powered by IBM focuses on enhancing customer experiences and optimizing supply chains. These applications analyze purchasing patterns and inventory levels to enable retailers to make data-driven decisions. A major characteristic of retail analytics is its customer segmentation feature, which helps businesses tailor offerings to diverse consumer groups. This specialty makes it a popular choice in the retail industry. The unique feature within this application is its use of machine learning algorithms to forecast demand accurately, thus minimizing excess inventory and potential losses. Despite these advantages, companies must remain vigilant about data accuracy and the complexity of integrating these analytics into their existing systems.
Augmenting Decision-Making Processes
The integration of IBM's data and analytics solutions significantly enhances decision-making processes across industries. By eliminating guesswork and providing insights grounded in data, organizations can confidently navigate the complexities of modern business environments. The importance of these solutions extends beyond mere analytics; they represent a strategic asset in achieving organizational objectives. They foster a culture of data-driven decision-making that can directly contribute to competitive advantage.
"Data is the new oil, but like oil, it must be refined to be useful."
This highlights the necessity of having sophisticated analytics tools that can refine raw data into valuable insights.
Current Trends in Data Analytics
The landscape of data analytics is constantly evolving. Keeping up with the latest trends is vital for organizations that aim to stay competitive. Today, businesses are not just looking at historical data; they want predictive insights that guide future decisions. Understanding these current trends helps professionals in the IT field, software developers, and students grasp how data can shape decisions and outcomes.
The Rise of Predictive Analytics
Predictive analytics is becoming increasingly important in various industries. This method uses statistical algorithms and machine learning techniques to identify the likelihood of future outcomes based on historical data. For instance, many retail companies utilize predictive analytics to forecast sales and manage inventory more effectively.
These insights assist not only in operational efficiency but also in customer engagement. By analyzing past behavior, companies can tailor marketing efforts to individual needs. This targeted approach can significantly increase conversion rates.
The adoption of predictive analytics is not without challenges. Data quality plays a crucial role in the reliability of predictions. Besides, understanding the balance between human intuition and algorithmic decision-making is essential. Organizations must ensure that their predictive models are transparent and understandable to foster trust in their analytics.
Data Visualization Techniques
Data visualization has transformed the way information is presented. Complex datasets can overwhelm decision-makers. Thus, effective visualization techniques simplify data interpretation. Tools like Tableau or IBM Watson Studio help turn raw numbers into intuitive visual formats. These visual representations can take forms like charts, graphs, or dashboards.
With the rise of big data, the need for effective visualization becomes more significant. Visual tools allow stakeholders to identify trends and anomalies that might otherwise go unnoticed. Properly designed visuals can communicate complex ideas quickly, aiding in faster, more informed decision-making.
However, it is crucial to maintain clarity. Overly complicated graphics can lead to misinterpretations. Hence, creators should focus on simplicity while ensuring that the visual communicates the required information effectively.
Machine Learning and Automation
Machine learning continues to influence the data analytics space. This field enables systems to learn from data and improve from experience without explicit programming. Automation in data analytics streamlines repetitive tasks, allowing professionals to focus on analysis rather than data handling.
As organizations collect vast amounts of data, leveraging machine learning becomes indispensable. Algorithms can uncover hidden patterns and provide insights that humans might miss. For example, fraud detection in financial services often relies on machine learning to analyze transactions in real time.
Automation also brings efficiency. Tasks such as data cleaning and preprocessing, which are time-consuming, can be automated. This automation not only speeds up the process but also reduces the likelihood of errors. By integrating machine learning with traditional analytics processes, companies can enhance their analytical capabilities and drive better decision-making.
"Embracing current trends in analytics is not just about adopting the latest technology; it’s about transforming how organizations understand and leverage their data for strategic advantage."
In summary, the current trends in data analytics dictate how organizations approach decision-making. Professionals and students in IT must recognize the impact of predictive analytics, data visualization, and automation. Staying informed of these trends is essential for driving innovation and maintaining relevance in an ever-changing market.
Challenges in Data Management
Data management is a cornerstone of successful data analytics. It encompasses strategies that govern how data is collected, stored, and utilized. As organizations increasingly rely on data to drive decision-making, addressing the challenges in data management becomes crucial. Issues can arise from various fronts, including data quality, regulatory compliance, and scalability. Each of these challenges necessitates careful consideration to ensure an effective analytical process.
Data Quality and Consistency Issues
Data quality is fundamental. Poor quality data can lead to misinformed decisions that impact organizational effectiveness. Issues like inaccuracy, incompleteness, and inconsistency can skew analytics results. When multiple sources of data exist, ensuring their harmonization is vital.
To combat these issues, organizations should employ data validation techniques. Regular audits can identify discrepancies, while data cleansing tools can rectify inaccuracies. It is also essential to create a culture that values data quality across departments. Encouraging consistent data entry practices helps maintain a high standard of quality. Without addressing data quality and consistency, firms may find themselves making decisions based on flawed information.
Regulatory Compliance and Data Privacy
As data privacy regulations become stringent, compliance is a challenge that no organization can overlook. Laws like GDPR and HIPAA impose strict guidelines for handling sensitive data. Non-compliance can result in significant penalties and reputational damage.
Organizations must develop robust policies that ensure adherence to regulations. This includes implementing data encryption, user access controls, and rigorous data handling procedures. Regular training for employees on compliance issues is also necessary. A proactive approach not only safeguards against fines but also fosters trust with customers. Protecting data privacy is non-negotiable for sustainable business practices.
Scalability Concerns for Big Data
With the explosion of big data, scalability emerges as a pressing concern. Many organizations struggle to adapt their data management systems to handle increasing data volumes efficiently. If systems are not scalable, performance may deteriorate. This could lead to delays in data processing and analysis, hampering timely decision-making.
Investing in scalable data solutions is critical. Cloud-based platforms, for instance, offer flexibility that on-premises systems may lack. They can dynamically allocate resources as demand fluctuates. Additionally, organizations should regularly assess their infrastructure to ensure it meets evolving data volumes. Scalability is ultimately about preparing for the future, enabling businesses to leverage data effectively as their needs grow.
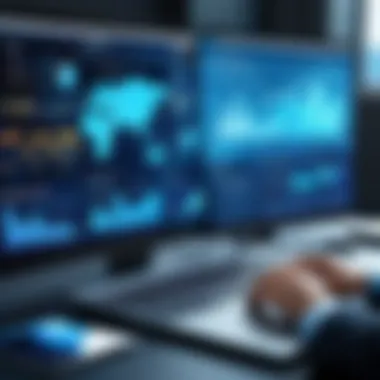
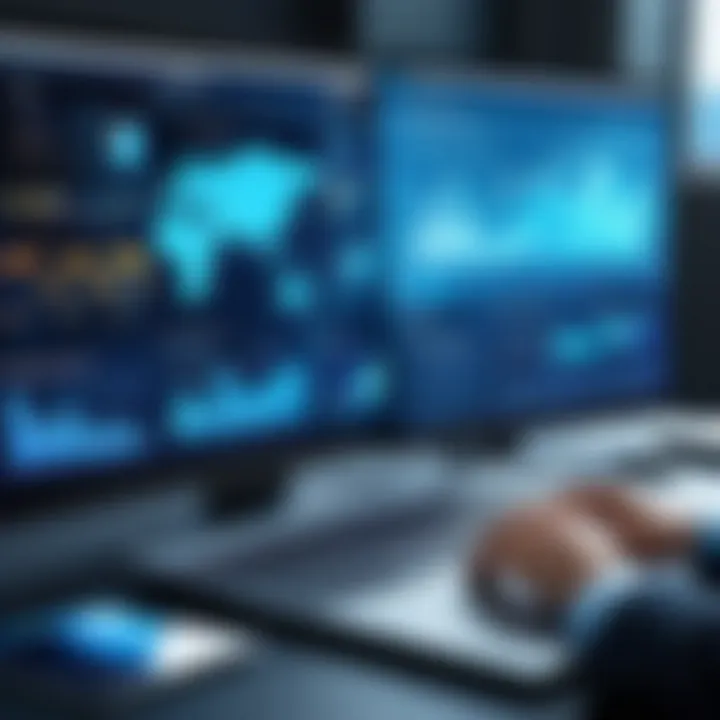
"Understanding and addressing data management challenges is essential for harnessing the true power of data analytics."
Ethics in Data Analytics
In the age of big data and advanced analytics, ethics in data analytics is a crucial topic. Organizations must understand the implications of their data practices to maintain the trust of stakeholders and the public. Ethical considerations help guide how data is collected, stored, analyzed, and utilized for decision-making. Missteps in these areas can lead to severe consequences, including privacy violations, discrimination, and damage to reputation.
Understanding ethics in data analytics helps ensure that data is handled responsibly. Organizations can create frameworks that address ethics in their analytical processes. This can lead to improved data quality and compliance with regulations, thereby enhancing overall integrity. Moreover, a strong ethical stance can foster innovation and creativity within organizations by encouraging responsible exploration of data-driven solutions.
Navigating Ethical Dilemmas
Navigating ethical dilemmas in data analytics requires a systematic approach. Organizations face multiple challenges, such as balancing user privacy with the need for data in developing insights. A common dilemma is whether to anonymize data or to make it identifiable to extract richer insights. This choice can lead to ethical challenges, especially if identifiable data is misused or mishandled.
Furthermore, bias in algorithms poses significant ethical concerns. Data used for training models can reflect societal biases, leading to unjust or discriminatory outputs. As a result, developers and data scientists must be diligent in ensuring their models are fair and equitable. Some strategies to navigate these dilemmas include:
- Establishing clear guidelines: Organizations should create robust data governance policies that outline ethical standards.
- Conducting regular audits: Frequent assessments of data usage and analytics processes can identify potential ethical issues before they escalate.
- Encouraging collaboration: Engaging diverse teams in the data analysis process can provide different perspectives and reduce biases.
Fostering Transparency and Trust
Fostering transparency and trust is essential for the ethical practice of data analytics. When organizations are open about their data practices, stakeholders can understand how data is collected and used. This transparency fosters a sense of security and confidence among the public and clients.
Several approaches can promote transparency:
- Clear communication: Detailing data usage policies helps build trust. Stakeholders should be informed about how their data will be utilized.
- User control: Implementing features that allow users to manage their data can empower them and enhance trust.
- Ethical training: Providing education on data ethics to all employees ensures that everyone understands the importance of ethical considerations.
Understanding the importance of ethics in data analytics is a vital aspect of responsible data usage. It equips organizations to navigate challenges and build trust, which ultimately leads to better decision-making processes.
The Future of Data Analytics at IBM
Understanding the future of data analytics at IBM is of paramount importance. As organizations become increasingly reliant on data, IBM is at the forefront, innovating solutions that leverage analytics for better decision-making. This future revolves around enhancing capabilities, addressing emerging needs, and fostering a data-driven culture within enterprises. By embracing advanced technologies and methodologies, IBM positions itself to guide businesses in navigating the complexities of big data and analytics.
Innovations on the Horizon
IBM is continually pushing the boundaries with new innovations. One significant development is the expansion of artificial intelligence (AI) and machine learning in analytics. By integrating AI, organizations can realize predictive capabilities that were not previously possible. This facilitates deeper insights through natural language processing and automated data analysis.
Additionally, IBM is exploring quantum computing as a potential game-changer in data analytics. Such advancements can lead to unparalleled processing power. Organizations can handle vast datasets more effectively, uncovering insights at an unprecedented scale.
IBM also invests in data integration tools, which streamline the processes of merging and analyzing data from disparate sources. This innovation ensures that businesses can gain a holistic view of their data landscape.
"The integration of advanced technologies in analytics will redefine how businesses can operate, driving efficiency and smarter decision-making."
These innovations not only enhance the analytical capabilities but also provide a competitive edge to organizations willing to invest in these tools.
IBM's Vision for Next-Gen Analytics
IBM's vision for next-gen analytics is centered on adaptability, accessibility, and actionable insights. The company envisions a future where analytics are embedded into various organizational processes rather than existing as standalone systems. This seamless integration will facilitate real-time decision-making based on current data insights.
Furthermore, IBM aims to democratize analytics. By offering user-friendly interfaces and reduced complexity, employees across all levels can utilize these tools without requiring extensive technical expertise. This democratization fosters a data-centric culture, empowering all stakeholders to engage in decision-making based on solid data.
To address future challenges, IBM is committed to enhancing data governance. This involves ensuring data quality, compliance, and ethical considerations remain priorities as companies proceed with data use. By proactively approaching these concerns, IBM prepares organizations for an increasingly complex regulatory environment.
In summary, IBM's future in data analytics hinges on technological innovation and a commitment to accessibility and governance. This vision aligns with the growing demand for robust data strategies, equipping organizations to thrive in an era defined by data-driven insights.
The End
The conclusion of this article encapsulates the essential findings from the exploration of IBM's data and analytics solutions. In a world where data is an invaluable asset, understanding how to harness it can significantly influence organizational decision-making. IBM's tools and technologies provide insights that are critical for effective operations across various sectors.
Summarizing Key Insights
Throughout this discussion, several key points emerge clearly. First, IBM’s data analytics offerings stand out for their advanced capabilities. They enable organizations to engage deeply with their data, revealing patterns and trends that may not be immediately visible. These insights facilitate informed decisions, driving more effective outcomes and strategic planning.
- IBM Watson Analytics exemplifies this capability, utilizing AI to enhance data interpretation.
- The importance of data governance is also pivotal; organizations must ensure accuracy and integrity in their data collection methods.
- Additionally, industry-specific applications demonstrate the flexibility of IBM's solutions. From healthcare to financial services, tailored use cases highlight the practical benefits of analytics.
By leveraging these insights, businesses can not only react to market changes but also anticipate future trends, thereby gaining a competitive edge.
The Continuing Evolution of Data and Analytics
Looking forward, the field of data analytics continues to evolve. IBM is at the forefront of this evolution, constantly innovating and integrating new technologies. As organizations face increasing volumes of data, scalability will become even more crucial.
Key areas to watch include:
- Integration of machine learning: This will enhance predictive analytics further and enable more sophisticated data handling.
- Improvements in data privacy: Addressing regulatory compliance will be vital as public concern about data security grows.
- Enhanced user experience design: This ensures that advanced systems remain accessible to users, allowing velocity in decision-making.
In summary, as IBM enhances its capabilities, the role data analytics play in strategic decision-making will only increase. Organizations that adapt to these changes will likely thrive in a data-driven future.