Exploring Medallia Text Analytics for Business Insights
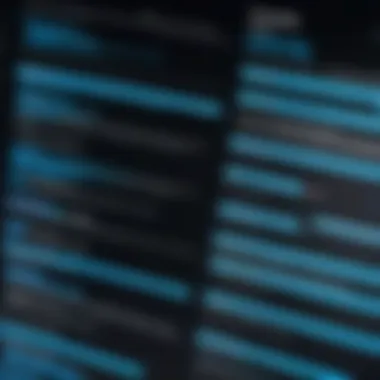
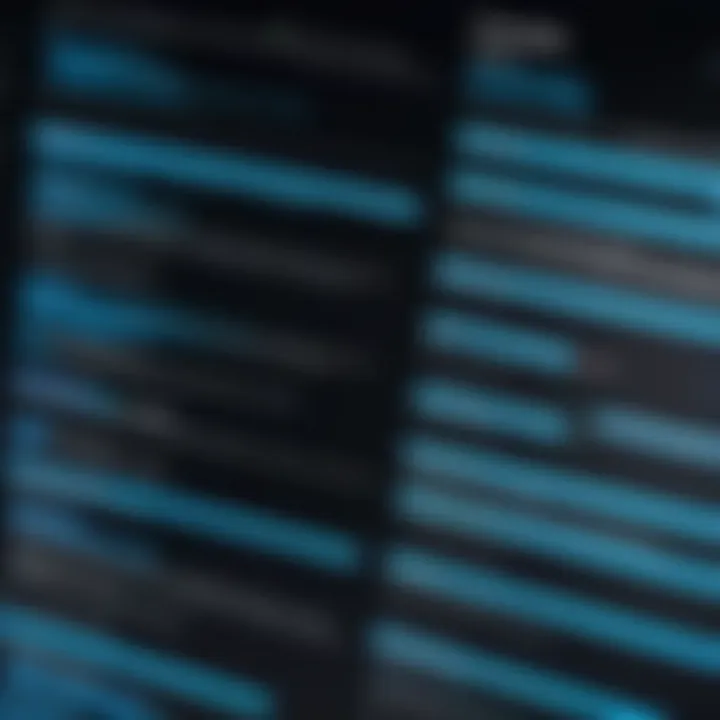
Intro
Understanding Medallia Text Analytics requires a thorough examination of its components and the framework it operates within. This text analytics tool is specifically designed to sift through unstructured data. Businesses increasingly turn to this technology to extract valuable insights from customer feedback, social media comments, and other textual content. The aim is clear: convert raw text into actionable intelligence.
Software Overview
Purpose and function of the software
Medallia Text Analytics stands out in its capability of transforming unstructured data into structured insight. This software aims to support organizations in comprehending vast amounts of text information efficiently. It analyzes sentiment and trends, allowing businesses to engage effectively with their customers’ opinions and emotions. The insights derived from this analysis can inform decision-making and enhance customer strategies.
Key features and benefits
Medallia Text Analytics boasts numerous features that enhance its functionality. Some notable aspects include:
- Natural Language Processing (NLP): This engine allows the software to recognize and interpret human language intuitively. NLP is essential for understanding customer sentiment and feedback nuances.
- Sentiment Analysis: Businesses gain valuable insights as the software evaluates the emotional tone behind customer texts. It categorizes feedback into positive, negative, or neutral sentiments.
- Trend Recognition: The system identifies patterns over time. Recognizing emerging trends can help businesses adapt their strategies and stay relevant in a competitive market.
These key features contribute significant benefits, like improved customer satisfaction, enhanced product development, and refined marketing campaigns.
Installation and Setup
System requirements
To successfully deploy Medallia Text Analytics, certain system requirements need to be met. Users typically need:
- A compatible operating system (usually recent versions of Windows, Linux, or macOS)
- Adequate RAM (8 GB minimum recommended)
- Sufficient storage space (preferably SSD for faster performance)
- A stable internet connection for cloud functionalities
Installation process
Installing Medallia Text Analytics involves several key steps:
- Download the software: Obtain the latest version from the official Medallia website.
- Run the installer: Open the installation file and follow on-screen instructions carefully.
- Configure settings: After installation, users must set their preferences, such as language, data sources, or integrations with other tools.
- Launch and test: Finally, run a few test analyses to ensure everything is functioning as expected.
Adhering to these steps can lead to a seamless setup experience.
"Understanding how to derive insights from unstructured data is crucial in today's information-rich environment."
Prelims to Medallia Text Analytics
Understanding Medallia Text Analytics is critical in grasping the complexities of extracting actionable insights from unstructured text data. This section serves as a foundation for the exploration of Medallia's capabilities and relevance in the modern business landscape. It highlights the vital elements that enable organizations to capitalize on customer feedback and sentiment.
Definition and Purpose
Medallia Text Analytics is a sophisticated tool designed for parsing and interpreting unstructured text data. Its primary purpose is to transform raw data from various sources, such as customer reviews, social media comments, and open-ended survey responses, into meaningful insights that can drive business decisions. This transformation allows companies to understand customer sentiment and preferences, which is essential for enhancing user experience and satisfaction.
By employing Natural Language Processing (NLP) algorithms, the tool not only identifies key themes in the text but also assesses the sentiment behind those themes. This functionality equips businesses with the ability to monitor customer opinions in real-time and adjust strategies accordingly.
Importance in the Digital Age
In today’s digital age, where interaction often occurs through various online platforms, understanding customer sentiment becomes paramount. The vast amounts of unstructured data generated by customer interactions present both a challenge and an opportunity.
With Medallia Text Analytics, businesses can leverage this data for competitive advantage. The ability to analyze sentiment quickly and effectively allows companies to respond to trends and issues as they arise. Moreover, by integrating insights gathered from text analytics into decision-making processes, organizations can tailor their offerings and customer engagement strategies to meet evolving consumer needs.
"Companies that leverage advanced text analytics can enhance their customer interactions and build enduring relationships."
In summary, the significance of Medallia Text Analytics lies in its capacity to bridge the gap between raw data and strategic business insights. It not only highlights customer sentiment but also illuminates areas for improvement, giving businesses a clearer path forward in a competitive landscape.
Key Features of Medallia Text Analytics
Medallia Text Analytics distinguishes itself through several key features that empower businesses to extract actionable insights from vast streams of unstructured text data. Understanding these features is vital as they lay down the groundwork for how organizations can effectively leverage this tool for enhanced customer understanding and informed decision-making.
The cornerstone of Medallia’s framework is its robust ability to interpret human language at scale. This interpretation is rooted in advanced algorithms and technologies that enable the platform to handle a multitude of queries and interpretations in real-time. The features also include versatility in data sources, allowing for an extensive range of data inputs from various customer interactions, be it social media, surveys, or support tickets. The efficiency in processing and analyzing these disparate data sets is critical for obtaining coherent insights.
Natural Language Processing Capabilities
Natural Language Processing (NLP) is a pivotal element of Medallia Text Analytics. It involves the use of sophisticated algorithms to understand, interpret, and generate human language in a valuable way. The importance of NLP lies in its capacity to convert qualitative text data into quantitative insights. It helps in identifying sentiment and emotions expressed by customers, providing a clearer picture of their experiences and expectations.
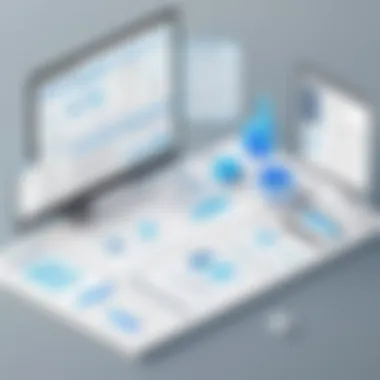
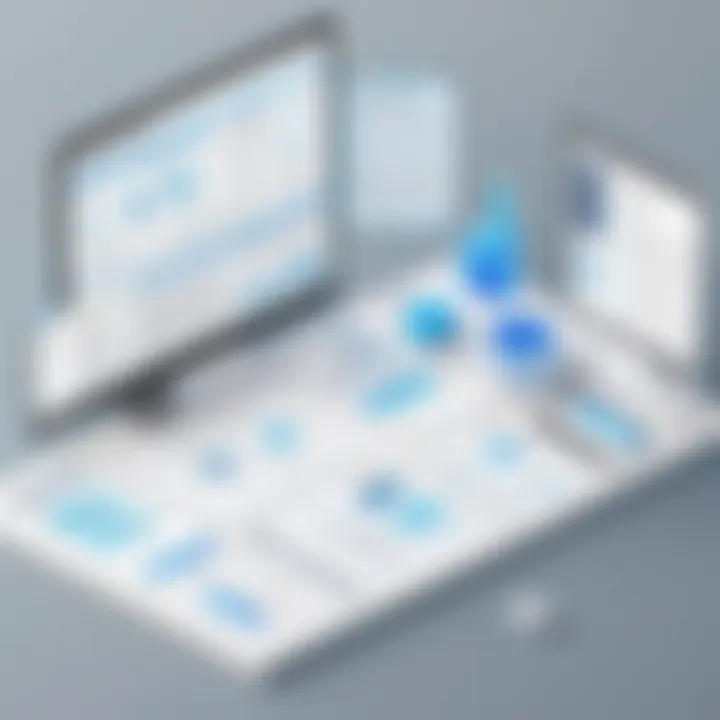
In practice, Medallia employs NLP to flag trends, categorize responses, and even summarize customer feedback. This is essential for companies looking to grasp nuances in consumer behavior and sentiment effectively. The multilayered analysis can also enhance automated response systems, allowing organizations to respond rapidly to customer concerns and feedback. By facilitating a deeper understanding of customer interactions, organizations can tailor their strategies for engagement and improvement.
Machine Learning Integration
Machine Learning (ML) is another critical feature that enhances the capabilities of Medallia Text Analytics. The integration of ML allows for continuous learning from new data, thus refining analyses over time. As the system processes incoming text data, it learns from previous interactions, adapting its algorithms to improve the accuracy of sentiment detection and insight extraction.
This integration not only augments the analytics process but also supports predictive capabilities. Businesses can forecast trends based on historical data, adapting their strategies proactively rather than reactively. For instance, if a retail organization observes rising negative sentiment about a certain product line, it can address potential issues before they escalate into broader problems.
"By leveraging Machine Learning, Medallia Text Analytics transforms a reactive approach into a proactive strategy, positioning organizations ahead of potential issues in customer experience."
Furthermore, the use of Machine Learning encompasses clustering techniques that group similar topics or sentiments, providing additional layers of understanding. This results in actionable insights that are not just a reflection of the customer’s current status but also an indication of future behaviors.
In summary, the Key Features of Medallia Text Analytics not only demonstrate the platform’s sophistication but also highlight its potential as a game-changer for businesses aiming to harness the power of unstructured text data. With robust Natural Language Processing Capabilities and effective Machine Learning Integration, organizations can achieve a comprehensive and accurate understanding of customer sentiment, making informed decisions that align with consumer needs.
Data Input Mechanisms
Data input mechanisms are crucial for determining the quality and effectiveness of any analytics solution. For Medallia Text Analytics, these mechanisms allow for the acquisition of data, which is fundamental for delivering insights. Choosing the right input method influences both the accuracy of analyses and the insights derived from unstructured text. Organizations can gain a clearer understanding of customer sentiments and feedback, which is pivotal in today’s data-driven landscape.
Structured vs. Unstructured Data
When discussing data input mechanisms, it is necessary to distinguish between structured and unstructured data. Structured data refers to information that is organized in a defined manner, typically within relational databases. Examples include spreadsheets or SQL databases. This format makes it simple to analyze as the data resides in neat tables with clear labels and types.
Unstructured data, on the other hand, encompasses a much broader range of content. This includes text from social media posts, open-ended survey responses, emails, and conversations. Since much of the data in the digital age is unstructured, Medallia's ability to process and analyze this type of data is crucial. Analyzing unstructured data can unveil significant insights that structured data alone may not reveal.
"The ability to harness both structured and unstructured data is vital for comprehensive analytics."
Understanding the differences allows businesses to leverage their text analytics capabilities effectively. Structured data facilitates quick analysis, while unstructured data offers depth and nuance to sentiment analysis.
Common Data Sources
Medallia Text Analytics can utilize various data sources to feed its analytical processes. Some common input sources include:
- Customer Feedback Forms: These forms often contain unstructured responses that provide insights into customer experiences.
- Social Media Platforms: Insights from platforms like Facebook or Twitter can reflect public sentiment about a brand or service.
- Email Communications: Analyzing emails can highlight customer concerns and questions, offering valuable feedback.
- Surveys and Reviews: Responses collected through surveys or ratings can serve as direct indicators of customer satisfaction.
- CRM Systems: These systems store customer interaction data, which can be analyzed to understand behaviors and preferences.
Each of these sources brings a unique set of information that, when processed correctly, can aid in drawing meaningful conclusions. By integrating diverse data sources, Medallia Text Analytics can provide a holistic view of the customer landscape, enabling businesses to make more informed decisions.
Analytical Frameworks Utilized
In the realm of Medallia Text Analytics, analytical frameworks form the backbone of the technology's capability to derive insights from textual data. These frameworks enable organizations to systematically analyze unstructured data, facilitating enhanced decision-making. An understanding of analytical frameworks utilized not only informs users about how the systems work but also sheds light on their applications across various industries.
Sentiment Analysis Models
Sentiment analysis models are essential components of Medallia Text Analytics. They interpret and classify emotions conveyed in text, distinguishing between positive, negative, and neutral sentiments. Businesses can leverage these insights to gauge customer perceptions and identify trends in consumer opinions.
There are various methods for performing sentiment analysis. Some use lexicons of words associated with specific sentiments while others employ machine learning techniques to develop predictive models. Machine learning approaches can be effective due to their ability to adapt to new data, continuously improving accuracy over time.
Moreover, understanding sentiment can lead to actionable strategies. For instance, a retail company may find that a product receives consistent negative feedback. By analyzing this data, the company can address customer complaints, ultimately enhancing its product offerings. This proactive engagement is crucial in today’s consumer-driven market landscape.
Topic Modeling Techniques
Topic modeling techniques serve another crucial function in Medallia Text Analytics. These techniques help organizations to automatically identify topics within a collection of documents or customer feedback. By discovering hidden themes, businesses can better understand their audience's interests or concerns.
One popular method for topic modeling is Latent Dirichlet Allocation (LDA). This technique works by assuming that each document contains multiple topics, and each topic comprises a distribution of words. By processing large datasets, LDA can uncover these underlying structures, revealing insights that may not be immediately apparent otherwise.
The application of topic modeling extends to several industry sectors. For example, in healthcare, organizations can analyze patient feedback to identify common issues or areas of satisfaction. This, in turn, can guide improvements in service delivery. Similarly, for financial services, understanding common themes in customer interactions can improve service levels and product offerings.
Topic modeling not only improves understanding of large text datasets but also enables smarter, data-driven strategies for business growth.
In summary, the use of sentiment analysis models and topic modeling techniques is fundamentally crucial to the efficacy of Medallia Text Analytics. They provide organizations with structured ways to interpret unstructured data, ultimately leading to better insights and enhanced decision-making processes.
Implementation Strategies for Businesses
When companies explore Medallia Text Analytics, it is essential to understand how to effectively implement this technology within their operations. The integration of such a system can significantly impact performance and yield valuable insights. This section discusses the importance of focusing on implementation strategies, highlighting key elements, benefits, and various considerations.
The deployment of Medallia Text Analytics does not merely mean installing software. It involves careful planning and the consideration of existing technologies, processes, and workforce abilities. This methodical approach can help organizations realize the full potential of text analytics.
Integration with Existing Systems
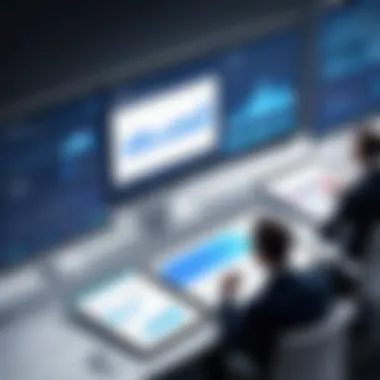
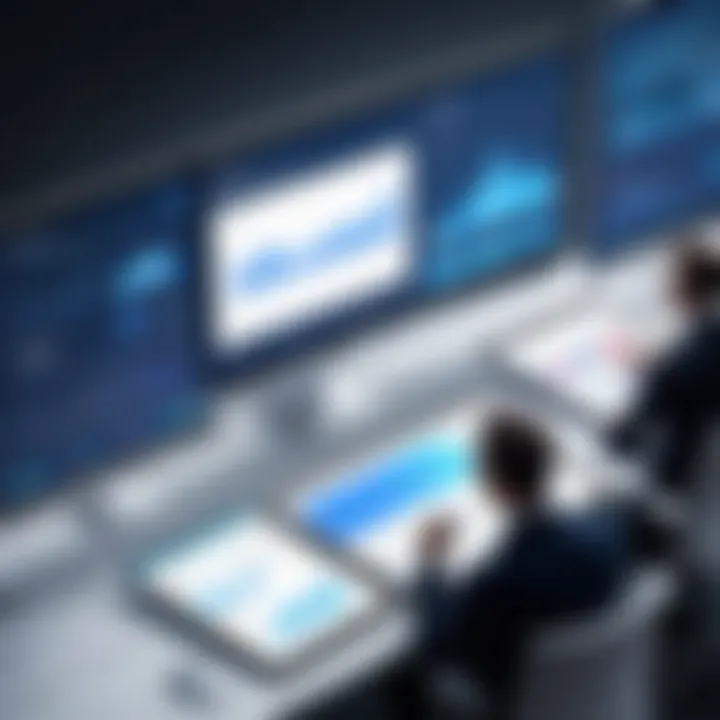
Integrating Medallia Text Analytics with existing systems is crucial for seamless functionality and maximum efficiency. A well-executed integration strategy allows valuable data from various sources to flow into the analytics platform.
- Compatibility: Organizations must assess the compatibility of Medallia with current systems. This includes CRM solutions like Salesforce or operational systems such as SAP.
- Data Migration: The process of migrating existing data is vital. Companies should map out how historical data can be imported without loss, enabling a comprehensive analysis of trends over time.
- APIs and Connectors: Using APIs (Application Programming Interfaces) simplifies integration. It allows disparate systems to communicate, thus streamlining processes. Well-documented APIs help developers create connectors that facilitate data exchange and enhance functionality.
Integrating with existing systems not only streamlines operations but also enhances the overall user experience. When systems communicate effectively, businesses can quickly respond to customer insights, thus improving service delivery.
Training and Onboarding
Ensuring that employees are trained effectively on how to use Medallia Text Analytics can be the difference between successful implementation and failed adoption. Targeted training and onboarding programs equip staff with the necessary skills to utilize analytics efficiently.
- Understanding the Tool: Employees should learn how to navigate the user interface and utilize essential features. A deep understanding of the tool helps maximize its value.
- Real-World Scenarios: Training sessions that involve real-world scenarios make learning practical. Employees should see the connection between theoretical knowledge and everyday tasks.
- Continuous Learning: The learning process should not stop after initial training. Continuous learning opportunities, such as workshops or online courses, keep employees updated on new features and best practices.
Effective onboarding fosters a culture where analytics is embraced and utilized in decision-making processes. When professionals understand how to leverage these insights, they can contribute more effectively to the company’s objectives.
"A successful implementation of text analytics in an organization hinges on the synergy between technology and people."
In summary, the implementation strategies for Medallia Text Analytics should focus on integration with existing systems and thorough training for employees. These steps ensure that businesses can fully utilize the insights provided by text analytics, thus enhancing decision-making and operational efficiency.
Use Cases in Different Sectors
Understanding the various applications of Medallia Text Analytics across sectors offers insights into its versatility and profound impact. Each sector faces unique challenges that can be navigated through effective data analysis. These use cases demonstrate how organizations can harness the power of text analytics to gain valuable insights, enhance customer experiences, and drive strategic decision-making.
Retail and E-commerce Applications
In the retail industry, understanding customer sentiment is critical. Medallia Text Analytics can analyze vast amounts of unstructured data, such as product reviews, social media comments, and customer surveys. By doing this, companies can identify trends and respond swiftly to changing customer preferences.
- Customer Feedback Analysis: Retailers use text analytics to monitor feedback on products and services. This helps identify popular items, areas for improvement, and new market opportunities.
- Personalized Marketing: Insights drawn from customer sentiment can inform targeted marketing strategies. Retailers can customize promotions and product recommendations based on customer opinions, maximizing engagement.
- Inventory Management: By analyzing text data related to product inquiries and reviews, companies can predict inventory needs, ensuring popular items are always in stock.
Healthcare Insights
The healthcare sector has seen a burgeoning interest in text analytics driven by the need for enhanced patient care. Medallia Text Analytics aids in analyzing patient feedback and clinical notes. This not only improves service delivery but also enhances patient safety.
- Patient Experience Improvement: Feedback from patient surveys can illuminate aspects of care that may require improvement, thereby directly enhancing patient experience.
- Clinical Insights: Healthcare providers can analyze notes and reports to identify common concerns and treatment outcomes, which can inform best practices and drive quality improvement initiatives.
- Regulatory Compliance: Text analytics can help healthcare organizations ensure they remain compliant with regulations by analyzing documentation and reports to spot potential issues before they escalate.
Financial Services Applications
In finance, companies must navigate a complex landscape marked by regulatory requirements and fluctuating market dynamics. Medallia Text Analytics supports financial institutions in analyzing customer interactions and risk assessments.
- Customer Sentiment in Banking: By harnessing text analytics, banks can gauge customer feelings regarding services, aiding in customer retention strategies and product development.
- Fraud Detection: Textual data from various sources, including transaction descriptions and customer communications, can be analyzed to spot anomalies indicative of fraud, protecting both banks and customers alike.
- Regulatory Reporting: Financial services firms can leverage text analytics for regulatory compliance, ensuring that all communications align with legal standards. This helps mitigate against compliance breaches that could lead to severe penalties.
Key Takeaway: Across sectors, Medallia Text Analytics empowers organizations to convert unstructured data into actionable insights, supporting enhanced performance and customer engagement.
Benefits of Medallia Text Analytics
Text analytics has become an essential aspect of data-driven decision-making. Medallia Text Analytics stands out for its ability to transform unstructured data into actionable insights. This section explores the benefits this tool offers, focusing specifically on how it enhances customer understanding and improves decision-making processes.
Enhanced Customer Understanding
In today's competitive landscape, understanding customer sentiment is key for business growth. Medallia Text Analytics enables companies to monitor and analyze feedback from various sources like surveys, social media, and customer reviews. By being able to dissect complex narratives, organizations can pinpoint customer pain points, preferences, and emotions. This understanding allows businesses to tailor their offerings and services more closely to the needs of their clientele.
The tool employs advanced natural language processing and machine learning algorithms to sift through volumes of text data. For instance, when analyzing customer reviews, Medallia Text Analytics can identify common themes and trends in sentiment. This feature can be particularly useful for marketing teams looking to develop targeted campaigns or for product managers aiming to refine existing products.
- Real-world Application: A retail company using Medallia Text Analytics might discover that a significant number of customers are dissatisfied with a product's usability. This insight allows the company to make informed adjustments to enhance customer satisfaction.
- Key Takeaway: Every interaction with customers through various platforms contributes to a wealth of data. Medallia ensures this data is not just archived but utilized effectively.
Improved Decision-Making Processes
Decision-making in any organization relies heavily on accurate and relevant data. With Medallia Text Analytics, businesses can access comprehensive insights derived from customer feedback, allowing for informed strategic decisions. For example, if multiple feedback entries suggest a demand for a new feature, product teams can prioritize their development accordingly.
Moreover, the tool supports data-driven approaches to risk management. When executives have a clear understanding of customer perceptions and sentiments, they can foresee potential issues before they escalate. Medallia helps in aligning business strategies with real-time customer data, making adjustments as needed.
- Benefits Overview:
- Enhanced visibility of customer needs.
- Informed decision-making based on rigorous analysis.
- Ability to respond proactively to market changes.
In summary, Medallia Text Analytics not only enhances customer understanding but also streamlines the decision-making process. These benefits make it a valuable asset for modern businesses looking to maintain a competitive edge.
Challenges and Limitations
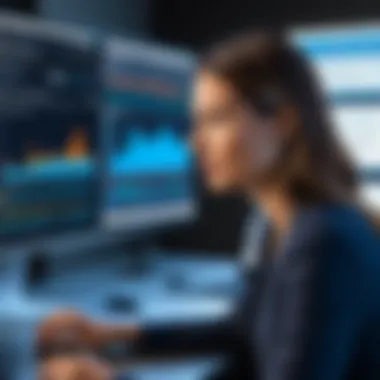
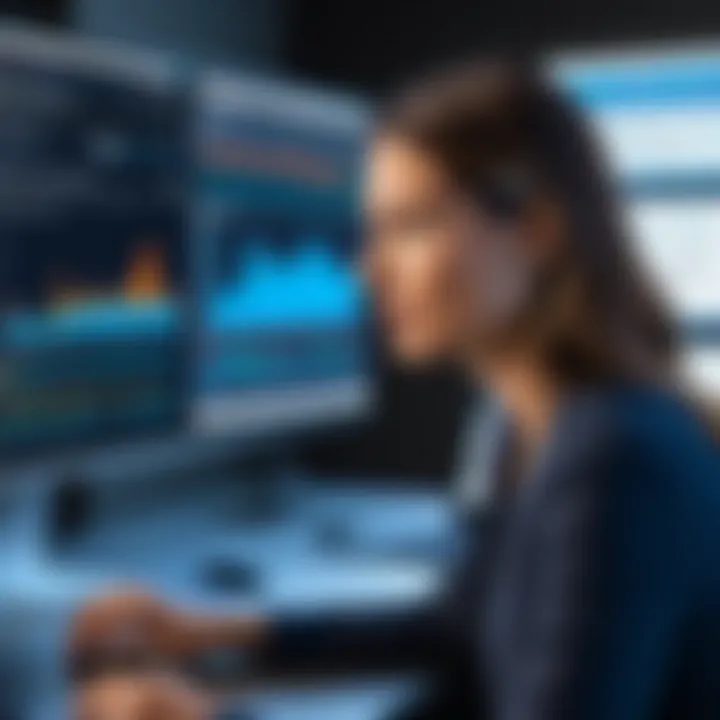
Understanding the challenges and limitations of Medallia Text Analytics is crucial for businesses looking to harness its power. While the tool offers robust functionalities, relying solely on its insights without considering potential pitfalls can lead to misinformed decisions. This section highlights data quality issues and the interpretability of results, both of which are fundamental for effective implementation.
Data Quality Issues
Data quality is a foundational element in text analytics. If the input data is flawed or inconsistent, it directly impacts the output generated by Medallia Text Analytics. Poor quality data can arise from several sources, including but not limited to incomplete datasets, noisy data, or biases embedded within the text.
- Incompleteness: Having missing data points can skew the results significantly. For instance, if customer feedback is not fully captured, the insights derived may not represent the entire customer sentiment.
- Noise: Unstructured texts often contain irrelevant or extraneous information. Filtering out this noise is necessary for achieving clarity in analysis.
- Biases: Data collected from a single source might inherently contain biases that could mislead interpretations. This occurs when certain customer sentiments are over or underrepresented.
All these factors underline the need for rigorous preprocessing of data. Implementing effective data cleaning methods before analysis can mitigate these risks, ensuring that the generated insights are valid and actionable. Involving data scientists or specialists in this phase maximizes the quality of the input data.
Interpretability of Results
The interpretability of results refers to how comprehensible the findings are when generated by the Medallia Text Analytics platform. High-quality insights are of little use if the stakeholders do not understand their implications.
- Complexity of Insights: Advanced algorithms and models used in Medallia can produce intricate patterns, which sometimes pose interpretive challenges. Stakeholders need clarity on what these results mean in practical, real-world scenarios.
- Trust in Automated Analytics: Users must have confidence that the findings are legitimate. If the results come across as too technical or opaque, it can lead to skepticism about the analytics process itself.
- Actionability: It is essential for businesses to translate analytical results into actionable steps. If the insights are difficult to comprehend, the potential for making informed decisions diminishes.
Proving the results through detailed documentation and providing contextual information can enhance interpretability. This effort allows stakeholders to align analytical outcomes with business strategies, ultimately ensuring better decision-making and operational success.
These aspects are vital when engaging with this technology to derive meaningful insights from customer feedback.
Future Trends in Text Analytics
The landscape of text analytics is continually evolving. As businesses seek to gain insights from unstructured data, understanding the future trends becomes imperative. The advancements in technology and shifts in consumer behavior play key roles in reshaping how text analytics is approached. This section will highlight significant emerging trends, their relevance, and the potential benefits they bring to organizations leveraging these analytics.
Advancements in AI and Machine Learning
AI and machine learning are vital components of modern text analytics. These technologies facilitate sophisticated methods for data processing and analysis. Companies are increasingly utilizing AI algorithms to improve accuracy in understanding customer sentiment, which stems from textual content.
Recent developments include the creation of better natural language processing (NLP) models. These models can interpret context more effectively, providing deeper insights than traditional methods. Models such as OpenAI's GPT-3 show the capability of generating human-like text, posing opportunities for automation in customer service responses or feedback analysis.
Additionally, advancements in unsupervised learning allow for more flexible data manipulation. This enables businesses to analyze vast amounts of data without needing extensive labeled datasets. Enhanced algorithms can recognize patterns and themes across diverse datasets, allowing for more targeted strategies.
"AI will not replace humans, but humans using AI will replace those who do not."
Furthermore, as AI become more accessible, even smaller companies can harness these powerful tools. The integration of AI and machine learning leads to improved predictive capabilities. Firm can anticipate customer needs more effectively, enhancing user experiences.
Increasing Importance of Real-Time Analytics
Another critical trend is the growing demand for real-time analytics. Businesses are moving toward immediate insights from their text data. Customers expect prompt responses, and companies need to act swiftly to maintain competitive advantage.
Real-time analytics facilitate timely decision-making. Platforms that analyze textual data instantly can highlight emerging trends, allowing companies to adjust marketing strategies quickly. For instance, social media monitoring tools utilize real-time analytics to capture conversations around brands, enabling firms to respond proactively.
The importance of real-time capabilities cannot be overstated. Organizations that can interpret customer feedback as it happens can pivot their strategies based on real-time sentiment, thus enhancing their engagement techniques.
Furthermore, technological advancements such as cloud computing make it easier for firms to adopt real-time data analytics. With scalable solutions, organizations can securely access and analyze data on the fly without significant infrastructural investment.
In summary, future trends in text analytics signal a shift towards greater efficiency and responsiveness in extracting insights from unstructured data. Advanced AI and machine learning algorithms are set to propel organizations forward, while real-time analytics emphasize the need for immediacy in decision-making processes. These trends not only enhance operational effectiveness but also enable firms to align closer with customer expectations.
Culmination
The conclusion is a vital section in this article as it synthesizes the information presented and underscores the significance of Medallia Text Analytics in contemporary business environments. By reflecting on the key points discussed, it not only reinforces the value of the analytics tool but also enhances understanding among diverse audiences.
Summary of Key Insights
In the earlier sections, we explored essential features like Natural Language Processing and Machine Learning integration that are foundational to Medallia Text Analytics. Accompanying these features, we analyzed various data input mechanisms, distinguishing between structured and unstructured data, while highlighting the importance of source quality.
We also examined how analytical frameworks like sentiment analysis and topic modeling emerge as powerful tools for gleaning insights from vast amounts of textual data. The practical implementation strategies discussed provide businesses with a roadmap for integrating this technology effectively into their workflows.
Key insights summarized include:
- Natural Language Processing allows for nuanced understanding of customer feedback.
- Machine Learning enhances predictive analytics capabilities, aiding decision-making.
- Different sectors, like retail and healthcare, are already leveraging these tools for better outcomes.
- Implementing such analytics can uncover valuable customer insights that are often hidden in unstructured data.
The article highlights that while significant advantages exist, one must also consider challenges such as data quality and interpretability of results.
Implications for Future Research
Looking ahead, several implications arise from the findings discussed in this article. Future research on Medallia Text Analytics should focus on overcoming inherent data quality issues. As organizations yield more unstructured data daily, improving accuracy and relevance will be paramount.
Moreover, exploring advanced methods in artificial intelligence and natural language processing could significantly enhance the interpretability of analytical outcomes. Understanding how different industries adapt and evolve in using this technology will also present new opportunities for research. Behavioral patterns and sentiment shifts over time offer ripe areas for deeper exploration as they inform how brands can better connect with their customers.
Research efforts could also focus on the ethical implications of text analytics, especially concerning privacy and data security, ensuring that these insights do not come at the expense of consumer trust.
Overall, Medallia Text Analytics stands as an intersection of technology and business intelligence. It truly signifies how future advancements in the field can continue to shape data-driven decision-making processes.