Mastering NoSQL Databases: Effective Query Strategies
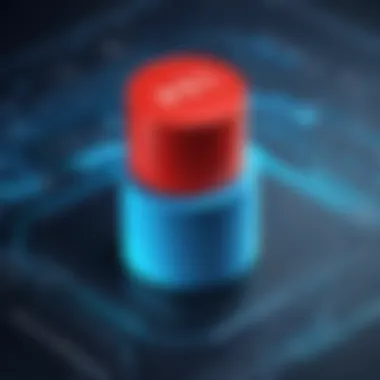
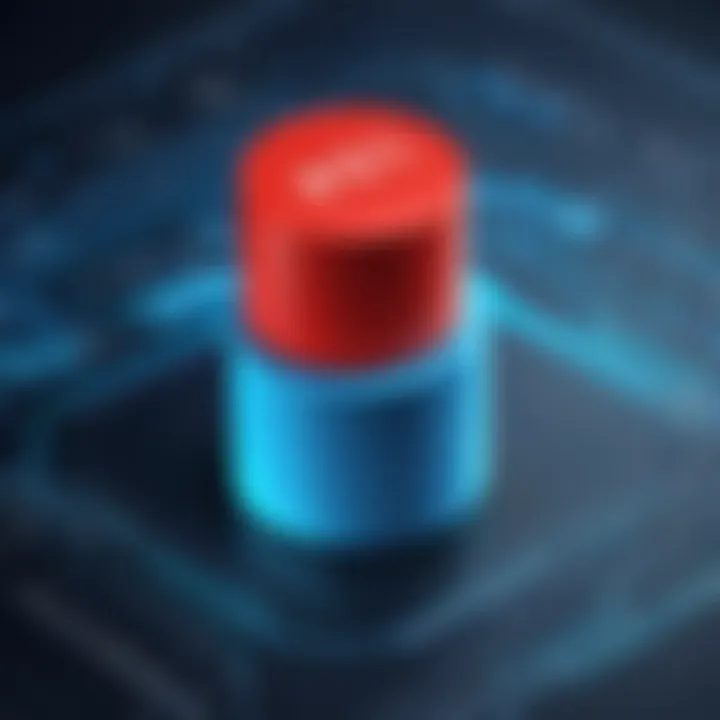
Intro
In the realm of data management, NoSQL databases have carved a niche that traditional relational databases often struggle to fulfill. As businesses generate massive amounts of unstructured and semi-structured data, the necessity for adaptive, scalable, and efficient data storage solutions rises. This article delves into the intricacies of querying NoSQL databases, providing insights aimed at improving proficiency for both seasoned tech professionals and curious learners.
Understanding how to navigate NoSQL databases is akin to learning a new language. The fundamentals might seem straightforward, but the nuances reveal themselves only through practical engagement. While relational databases are like a rigid grid where everything fits into neat boxes, NoSQL databases offer flexibility and schema-less architecture. This adaptability opens the door to various types of databases—key-value stores, document stores, wide-column stores, and graph databases—each designed for different data needs and querying methods.
As we peel back the layers of NoSQL querying, expect to explore specific techniques tailored to unique environments, practical examples, and the challenges that arise in real-world applications. We will also touch on best practices that can empower developers and data analysts alike.
"In the world of NoSQL, flexibility rules supreme. But with great flexibility comes the challenge of effective querying."
This introductory segment sets the stage for a comprehensive exploration of NoSQL querying strategies. From examining the software’s purpose to understanding installation, we will cover a variety of topics to facilitate efficient data retrieval. So let’s buckle up and traverse the landscape of NoSQL databases.
Intro to NoSQL Databases
In the digital age, where data generation is a ceaseless river flowing at unprecedented rates, understanding the nature of data storage and retrieval becomes crucial. This segment serves as a crucial touchstone, setting the stage for diving into the complexities and intricacies of NoSQL databases. Not only is it vital for professionals and students in IT-related fields to comprehend the fundamentals of NoSQL, but it also helps demystify the landscape for those who may have veered into this uncharted territory.
Definition and Characteristics
NoSQL databases, often called "non-relational databases," are designed to handle large volumes of data that do not always fit comfortably into the rows and columns dictated by traditional relational database models. Typically, NoSQL databases are more flexible, allowing for unstructured or semi-structured data formats. They march to the beat of a different drum, often described through characteristics that distinguish them distinctly:
- Scalability: Unlike traditional databases that tend to struggle under large data loads, NoSQL databases are built to expand horizontally. This means you can simply add more servers rather than overhauling the system.
- Schema Flexibility: Data can be stored without a predefined schema, making it easier to adapt to changes or new data types—an asset in agile development environments.
- High Performance: With no complex joins or extensive cases for data retrieval, NoSQL databases often offer faster performance for certain types of queries compared to their relational counterparts.
- Variety of Models: Whether through document stores, key-value pairs, or graph structures, there’s a NoSQL database model to address almost every data requirement.
These characteristics not only usher in a new paradigm for handling data but also highlight the need for specialists who can navigate this complex terrain.
The Evolution of Data Storage
Tracing back through the likes of punch cards, magnetic tapes, and the classic relational databases, data storage has undergone transformative changes. The innovation of NoSQL databases wasn’t born out of a vacuum; instead, it arose from the burgeoning web and the need to manage its voluminous and diverse data effectively.
With the explosion of social media and the rise of the Internet of Things, traditional databases began to buckle under the weight of sheer data. Realizing the limitations of relational models led to innovative thinkers establishing alternative approaches. Companies like Amazon, Google, and Facebook pioneered different NoSQL technologies that catered to their specific needs, from fast-read/write times to the sheer volume of diverse data generated every millisecond.
NoSQL vs Relational Databases
At the crux of data management lies a fundamental question: NoSQL or SQL? The key differences between these two categories highlight each one’s strengths and challenges:
- Data Model: Relational databases depend on structured data, whereas NoSQL can accommodate unstructured data, which is more representative of real-world information.
- Scalability: While relational databases often require significant resources to scale, NoSQL databases can easily adjust to increased loads.
- Transaction Consistency: SQL databases emphasize ACID compliance—ensuring reliable transaction management. NoSQL databases can sometimes sacrifice this consistency for availability and partition tolerance.
Understanding these differences not only aids in selecting the right database for a project but also illuminates the path forward for future-proofing applications in an era where data is king.
Types of NoSQL Databases
In the expansive realm of data storage solutions, NoSQL databases have carved their distinct niche. They cater to varied requirements that arise from different applications, be it web applications, real-time analytics, or even large-scale machine learning projects. Recognizing the types of NoSQL databases is pivotal because it influences how data is structured, queried, and effectively utilized.
Each type of NoSQL database adheres to unique principles, which provide various advantages such as flexibility in handling unstructured data and scaling horizontally. This section aims to shed light on the core varieties of NoSQL databases, emphasizing their roles, characteristics, and practical applications in modern-day data management.
Document Stores
Document stores take the concept of storing data one step further by organizing it in a way that resembles a document. Think of them as digital filing cabinets where each document contains data in formats like JSON or XML. MongoDB stands out as a primary example in this realm.
This model provides a flexible schema, allowing for dynamic modifications without the need to overhaul the entire structure. As applications evolve, so do the data requirements. In scenarios where the data schema needs to adjust frequently, document stores shine, ensuring that businesses can pivot with minimal disruption.
Key features of document stores include:
- Schema Flexibility: No rigid structure allows for easy alterations as application requirements change.
- Complex Data Types: Documents can store arrays and nested objects quite effortlessly, which is advantageous for applications needing rich data representation.
- Powerful Queries: With ad-hoc querying capabilities, you can quickly retrieve data leveraging indexes that cater to various query patterns.
Key-Value Stores
Key-value stores are akin to a giant dictionary; each unique key maps to a specific value, which can be anything from a simple string to a more complex object. Amazon DynamoDB falls into this category, famous for its reliability and speed. At their core, key-value stores prioritize speed and flexibility, making them perfect for caching and session management applications.
They offer several benefits:
- Simplicity: The data model is straightforward, facilitating easy retrieval and storage of data using a unique key.
- Scalability: They can seamlessly distribute data across multiple servers, which is crucial for high-traffic applications.
- Performance: Optimized for read and write operations, these databases can handle large quantities of data with minimal latency.
Column-Family Stores
Column-family stores, like Apache Cassandra, opt for a different approach where data is stored in columns instead of rows. This model allows for aggregation and compression, often resulting in better performance for certain queries. Their structure is best suited for analytical applications, as they can efficiently process queries that involve large datasets.
Some of their notable characteristics include:
- Wide Rows: Storing data in rows isn’t always necessary; wide rows allow for storing massive amounts of data in a single row, drastically improving performance in specific queries.
- Fault Tolerance: Designed to handle node failures effortlessly, making them ideal for environments that require high availability.
- Flexible Data Model: Adaptability in terms of schema allows developers to evolve application needs without significant overhead.
Graph Databases
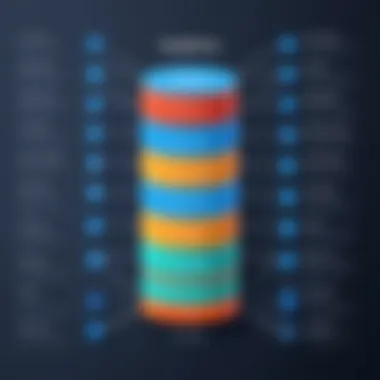
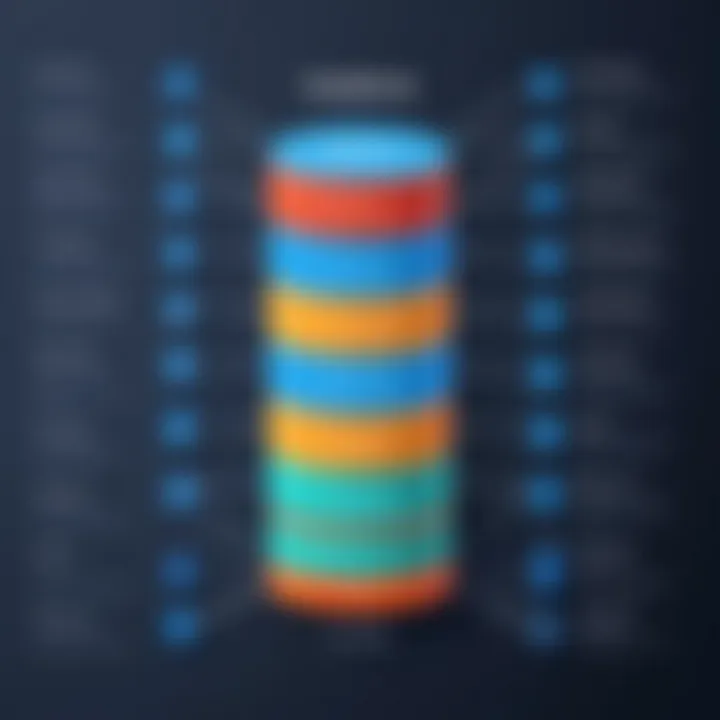
Graph databases specialize in understanding and querying complex relationships within data. They represent data in nodes and edges, akin to a web. Neo4j illicits attention in this area, making it easier to manage interconnected data effectively. This model proves especially effective for applications requiring extensive relationship analysis, such as social networks or recommendation systems.
Highlights of graph databases include:
- Complex Querying: They facilitate sophisticated queries about relationships, enabling businesses to glean insights from intricate interconnections.
- Performance at Scale: With optimizations for relationship-heavy queries, they can outperform traditional databases significantly in specific contexts.
- Intuitive Representation: The graph data model aligns closely with human reasoning, providing a more straightforward approach to visualizing relationships and connections.
Querying Basics
Understanding the fundamentals of querying in NoSQL databases is crucial for developers and data professionals alike. With the rise of non-relational database systems, traditional querying approaches have evolved to meet the needs of diverse data structures. Knowing the basics allows one to navigate through the complexities effectively, ensuring optimized data retrieval and manipulation. Querying, at its core, is about asking questions to the database and receiving answers that aid in decision-making.
Understanding Query Language
NoSQL databases employ various query languages designed to cater to specific data models. For instance, MongoDB uses its own syntax to interact with document stores, whereas Cassandra has CQL tailored for its column-family architecture. Understanding these languages is essential, as each comes with unique functions and capabilities.
The beauty of query languages often lies in their flexibility, accommodating a variety of data formats. This flexibility means you can retrieve information based on different criteria—whether through precise conditions or more relaxed, heuristic ones.
For example, in MongoDB, a simple query could look like this:
This query fetches all users who are 18 years or older, highlighting the powerful querying potential. Similarly, understanding how to write and optimize queries means that even large datasets can be navigated swiftly, thus improving application performance.
Specialized Query Techniques
Once a grasp on the basic query language is achieved, one can delve into specialized techniques that can enhance querying efficiency. Techniques vary from using indexes to structuring queries in ways that prevent bottlenecks. Here are some notable techniques worth considering:
- Indexing: An index can speed up data retrieval, making queries faster and easing the load on database systems. Without indexes, the database may scan through every record, which is not practical, especially for large datasets.
- Pagination: When dealing with a substantial amount of data, implementing pagination can improve user experience and system performance. Breaking down large datasets into smaller, manageable chunks ensures responsiveness.
- Aggregation Frameworks: NoSQL databases often come equipped with aggregation capabilities. For instance, MongoDB's aggregation pipeline allows for complex data transformations and analysis—helpful in tasks requiring summary statistics or data restructuring.
Employing these specialized techniques is vital for anyone looking to harness the true power of NoSQL databases. The right methods can lead to significant performance improvements, making applications robust and efficient.
The key to effective querying in NoSQL is not just understanding how to pull data, but also how to request it efficiently for your specific use case.
Such refinements in querying practices not only yield quick results but also enhance the overall usability and accessibility of data. This level of understanding sets the stage for more complex queries and better database management.
Querying Document Stores
Querying document stores is a pivotal aspect of leveraging the capabilities of NoSQL databases. With their flexible data models, document stores like MongoDB enable developers to store data in JSON-like structures, allowing for easy representation of complex data types. This flexibility can be a game-changer for applications that require quick iterations and constant updates, especially in environments where the data schema is likely to evolve.
One of the key benefits of querying document stores is the ability to manage unstructured and semi-structured data without the constraints imposed by traditional relational databases. This means that developers can easily adapt to changing business requirements without having to frequently adjust the database schema. For instance, imagine a content management system where each article has a different set of attributes; document stores allow these variations without the need to refactor a common schema.
Moreover, the capability to perform complex queries directly on nested documents adds another layer of efficiency. Users can filter or aggregate data based on various attributes, often resulting in faster execution times compared to relational databases that might struggle with similar queries. However, querying document stores isn't without its challenges; accessing deeply nested data can lead to performance bottlenecks if not handled properly.
As you navigate this landscape, understanding the strengths and weaknesses of querying document stores is essential for optimizing your applications. By mastering the specific queries and techniques tailored for document stores, you can harness the full potential of NoSQL.
Using MongoDB Query Language
Delving into MongoDB query language reveals a powerful tool that allows for efficient data manipulation and retrieval. Basic operations leverage a syntax that closely mirrors the structure of the stored data. This means that users can write intuitive queries that are easy to grasp, even for those who might not have extensive database training.
For instance, to find documents within a collection where the field for "status" has a value of "active," a simple query can be constructed like this:
This straightforward approach highlights the benefit of MongoDB’s expressive syntax. Additionally, MongoDB supports advanced querying features such as field projection and data aggregation, helping to refine the results further when needed. The built-in indexing options allow queries to execute with much greater speed, although choosing the right index requires careful consideration of read and write patterns.
Aggregation and Indexing Strategies
When it comes to maximizing performance in document stores, knowledge of aggregation and indexing strategies is crucial. Aggregation pipelines in MongoDB provide a powerful way to process data by transforming the collections into required output formats. Consider a scenario where an online bookstore wants to analyze sales data to gauge the performance of each genre. By setting up an aggregation pipeline, the bookstore could easily compute sums, averages, or counts without sacrificing performance.
This allows for detailed data analysis while keeping the operations within the NoSQL environment’s sweet spot—dealing with vast datasets efficiently.
Indexing, on the other hand, can significantly speed up query response times and should never be overlooked. Choosing the right type of index—whether single-field, compound, or geospatial—can make all the difference. It also requires an understanding of query patterns, as overly broad indexing can lead to unnecessary overhead and complexity.
In summary, while querying document stores like MongoDB offers significant flexibility, combining effective querying techniques with strong indexing strategies is vital to achieving optimal performance in real-world applications.
By mastering these aspects, professionals can better position themselves to take advantage of NoSQL capabilities, facilitating swift adaptations to changing data landscapes.
Querying Key-Value Stores
Key-value stores, as the name suggests, are databases that store data as a collection of key-value pairs. This simplicity is both their strength and their limitation. The ease of storing and retrieving data using keys makes these databases particularly suited for use cases where rapid retrieval of information is essential. From session management in web applications to caching frequently accessed data, key-value stores deliver a performance punch when speed is a priority.
Simple Retrieval Techniques
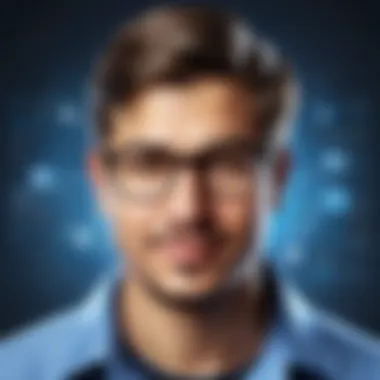
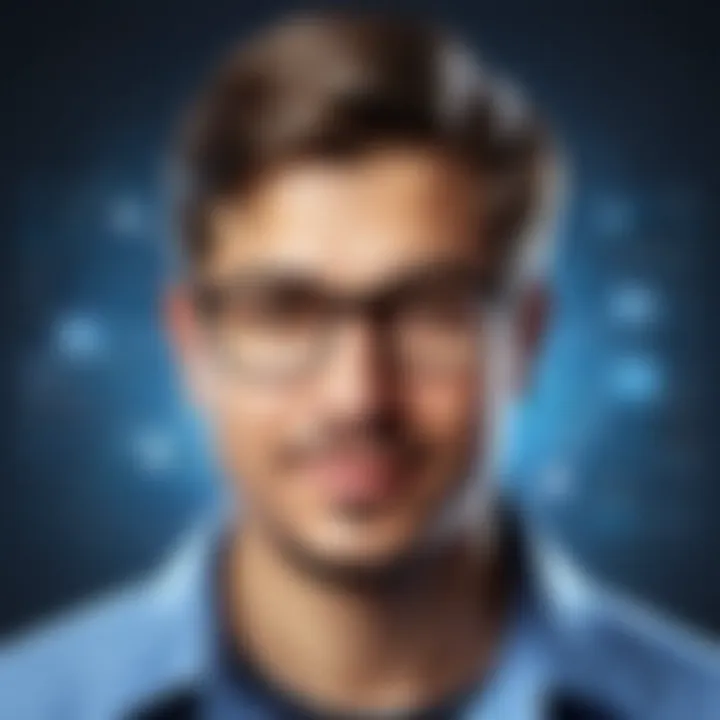
Retrieving data from key-value stores is straightforward, but there are nuances worth noting.
- Direct Key Access: The most common retrieval method is accessing data directly using the associated key. This technique exemplifies the efficiency of key-value stores. It's akin to having the perfect index in a book that gets you straight to the information you need, no leafing through pages required.
- Batch Retrieval: Many systems allow batch retrievals, where multiple keys can be queried in a single call. This approach minimizes round-trips to the server, optimizing performance further. However, the design must account for potential limits on the number of keys retrieved in one query.
- Using Secondary Indexes: Some key-value stores support secondary indexes. Though they introduce a bit of complexity, they enable users to query data based not solely on the primary key. For instance, if a store contains user profiles, you would typically retrieve a user's profile via their unique user ID. Still, if you want to query by age or location, secondary indexes can help in that direction.
Overall, these retrieval techniques show that while the operational model is simple, extracting data effectively relies on understanding its underlying structure.
Data Modeling Considerations
When working with key-value stores, how you mold your data can dramatically influence performance and usability.
- Choose Key Names Wisely: The key is your direct gateway to the data, so think carefully about how you structure them. Keys should be unique and descriptive enough to convey the purpose of the data behind them. A poor key can lead to confusion later, especially as the dataset grows.
- Effective Value Design: The value can be anything from a simple string to more complex objects like JSON. How you structure your value impacts retrieval efficiency. Denormalization techniques, where related data is stored together, can speed up reads significantly.
- Scaling Considerations: As your application grows, how will your key-value approach adapt? Your initial design might work for a small dataset but could falter under larger loads. Plan for scalability right from the start; this means anticipating future access patterns, potential bottlenecks, and how much data will be kept in memory.
Querying Column-Family Stores
Column-family stores have carved a significant niche in the vast landscape of NoSQL databases, primarily due to their ability to handle large volumes of data and their flexible schema design. Understanding how to effectively query these databases is critical for any data-driven application, especially those requiring rapid access to a massive amount of structured data.
Column-family stores, such as Apache Cassandra and Google Bigtable, allow users to store data in columns instead of rows, which can enhance performance for certain types of queries. This method can be a game changer when scaling applications. By organizing data in this way, queries that involve retrieving only specific columns can execute much faster, as the database engine doesn't need to sift through unused data.
Cassandra Query Language (CQL)
Cassandra Query Language, or CQL, serves as the main access language for databases like Apache Cassandra. It resembles SQL in its syntax, facilitating its adoption for those familiar with relational databases. However, the operational behavior is quite distinct. CQL focuses on terminology like "tables" and "rows" but extends into a non-relational structure, where data integrity must be maintained with a different mindset.
Here's a simple example of how to execute a basic query using CQL:
Such commands allow developers to perform CRUD (create, read, update, delete) operations efficiently while managing considerable datasets. CQL provides an abstraction that makes it easier to handle data arrangements, including using primary keys and clustering keys to organize data logically as well as performance-efficient.
"CQL acts as a bridge, merging the familiar concepts of SQL with the unique capabilities of NoSQL databases, making it approachable for developers from diverse backgrounds."
Managing Large Datasets
Managing large datasets in a column-family store requires careful consideration of how data is distributed across the cluster. Unlike traditional databases, where data is stored in fixed rows and columns, column-family stores inherently support a variable schema, allowing for more efficient storage of sparse data.
To optimize query performance, one must focus on the following:
- Data Modeling: Understand the access patterns of your application. Designing your tables with future queries in mind is key. For instance, if analytics or report generation will require multiple columns, design your table accordingly from the outset.
- Partitioning: Data distribution across nodes comes into play heavily. In Cassandra, a well-defined partitioning strategy helps to ensure even load among nodes. This safeguards against hotspots and keeps writes and reads efficient.
- Compaction: Regular maintenance processes, such as compaction, help manage large datasets by merging SSTables (Sorted String Tables) and reclaiming unused space, further enhancing performance.
The art of querying column-family stores lies not just in writing the correct syntax, but also in fully grasping the underlying architecture—ensuring each query runs as smoothly as possible while handling large-scale data efficiently.
Querying Graph Databases
When we look at the world of NoSQL databases, graph databases stand out as unique players. They offer distinct advantages over traditional databases, mainly due to their ability to efficiently manage data modeled in terms of nodes and relationships. Understanding how to query graph databases can provide powerful insights, making it an essential skill for developers and database administrators alike.
Graph databases are designed to handle structured data that is primarily concerned with relationships. The key here is that in many applications – think social networks, recommendation engines, or fraud detection systems – it's not just the data itself that matters, but how that data interacts. Efficient querying of these relationships allows one to traverse from one data point to another, shining light on patterns and connections that would otherwise remain obscured.
Cypher Queries in Neo4j
A primary player in the graph database arena is Neo4j, which employs Cypher query language. Cypher is designed specifically for expressing how to retrieve and manipulate graph data. One of its notable features is how it allows users to write queries that read like expressive, declarative sentences. For example:
This simple query finds all pairs of friends in a graph where nodes are connected by relationships.
Some critical elements when working with Cypher include the following:
- Pattern Matching: This is the linchpin of graph queries, where you outline what you want to find in the graph's structure.
- Filtering: You can add clauses to filter for specific values.
- Aggregation: Functions help to summarize data, akin to SQL but tailored for graph structures.
Using Cypher effectively requires an understanding of these principles, which can then lead to crafting queries that are optimized for performance and clarity.
Exploring Relationships and Patterns
Diving deeper, the beauty of querying graph databases lies in their ability to unveil relationships. Unlike traditional databases where relationships are often captured through foreign keys, graph databases bring these connections to the forefront.
When you query a graph database, think in terms of relationships. For example, in a social network graph, if you're looking to understand how many degrees of separation exist between two individuals, a simple query can yield that information quickly. Consider these insights:
- Traversing Relationships: By following the edges (relationships), one can find not just direct connections, but also indirect ones, which are crucial in understanding the data's context.
- Pattern Recognition: Querying not only for direct relationships but for more complex patterns can uncover insights like cliques or clusters within your data. This unlocks the potential for predictive analytics.
Graph databases also excel at dynamic schema changes, meaning as your data model evolves, your querying capabilities can adapt without needing complete redesigns.
"In an interconnected world, graph databases are the key to unlocking the narratives hidden within complex data relationships."
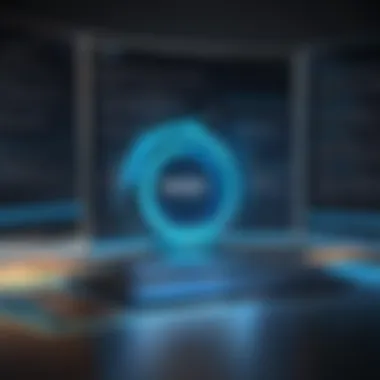
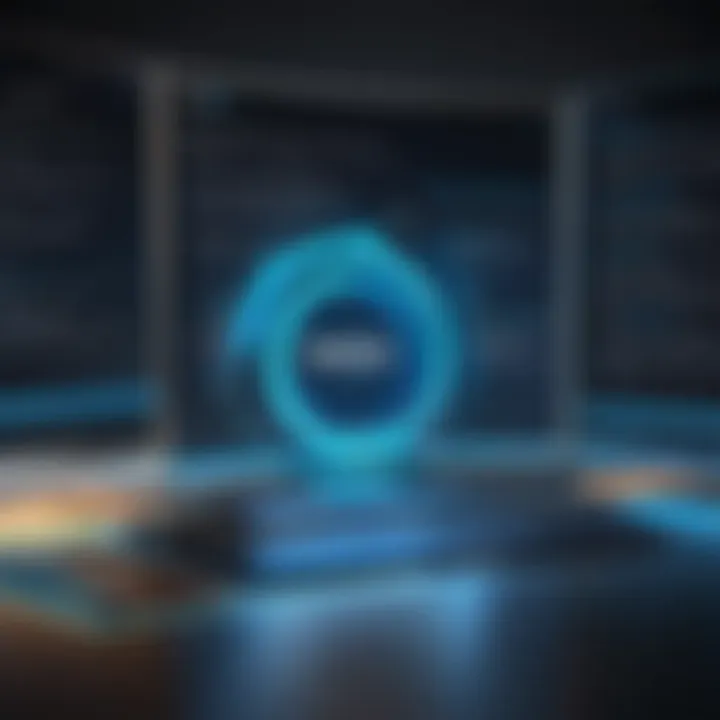
Challenges in NoSQL Querying
Navigating through NoSQL databases is akin to traversing a labyrinth; it’s full of twists, turns, and some unexpected barriers. Understanding the challenges in NoSQL querying is crucial, especially for professionals and students in IT-driven fields. These challenges impact the efficiency of data retrieval and can greatly affect overall system performance. In maintaining a delicate balance between consistency and availability, one must also comprehend the limitations of complex queries that arise in these non-relational systems. Identifying these obstacles is the first step in creating effective strategies to mitigate them, ensuring successful data management.
Consistency vs Availability
The trade-off between consistency and availability is a fundamental aspect of NoSQL databases. This tension is often framed by the CAP theorem, which contends that it’s impossible for a distributed data store to simultaneously guarantee consistency, availability, and partition tolerance. In simpler terms, when you have a choice between a reliable data update and continued access to the data, you often have to make a decision. This can certainly complicate querying strategies.
For instance, consider a social media application's database handling user posts. If one server is updated with a new post but another has not yet synchronized, users querying posts may see inconsistent data. The application has to determine whether to provide the most recent updates (favoring consistency) or to allow users continued access to existing data (favoring availability). This makes clearly defining the requirements of your application essential.
"Choosing between consistency and availability is like picking between two equally tempting desserts—sometimes you just can’t have both!"
Understanding this tradeoff is vital for developers aiming to design robust systems. If an application needs absolute consistency, employing strategies like using a leader election process or a quorum system can sync databases at the cost of availability. Conversely, allowing eventual consistency may provide a better user experience under heavy load, yet it also means that users might see outdated information temporarily.
Complex Query Limitations
Complex queries in NoSQL databases often present their own set of challenges. Unlike relational databases, where structured query language (SQL) provides a familiar syntax for complex joins and aggregates, NoSQL databases lack a universal querying framework. This leads to greater variability in how data can be accessed across different NoSQL systems.
Take MongoDB, for example. While it offers powerful aggregation tools, creating intricate queries involving multiple collections can become cumbersome. Instead of straightforward joins, developers must often reshape their data model to fit the queries they need, which may involve denormalization or embedding documents. This means that what could be achieved with a few SQL commands might require a more convoluted series of operations in NoSQL environments.
Also, performance can degrade with increasingly complex queries. For instance, slow query response times may occur if the database isn’t correctly indexed, or if the underlying architecture can’t support the requested operations efficiently.
Thus, understanding the limitations regarding query complexity is imperative for developers. Strategies including query profiling, careful index management, and data modeling reconsiderations help in optimizing query performance.
In summary, grasping the nuances surrounding consistency versus availability and recognizing the limitations of complex queries is essential when working with NoSQL systems. By addressing these challenges head-on, developers can enhance their skills and improve database interactions, ultimately leading to more effective data management for applications.
Best Practices for NoSQL Querying
Effective querying in NoSQL databases is not just about mastering the syntax of a specific query language; it is about developing an overall strategy that respects the data model, optimizes performance, and ensures the integrity of the data. Navigating NoSQL environments requires an understanding of their underlying principles and a thoughtful approach tailored to the specific requirements of your project. By implementing best practices, developers and data professionals can greatly enhance their querying capabilities, ultimately improving efficiency and output.
Optimization Techniques
Optimization techniques are the lifeblood of efficient querying in NoSQL systems. One needs to keep pace with growing datasets and complex requirements. For example, utilizing indexing properly can make a world of difference in the speed of queries. Indexing allows the database engine to locate and retrieve data without scanning entire datasets, which can be particularly beneficial in larger databases. Consider the following strategies:
- Use compound indexes when dealing with queries that filter on multiple fields. This can drastically reduce query time since the database can look up multiple criteria in one go.
- Regularly analyze query performance. If a query is consistently slow, it may be worth re-evaluating its structure.
- Batch operations whenever possible. Instead of performing numerous single operations that can result in overhead, grouping them can lead to more efficient data management.
Keeping an eye on the query execution plan provides insights into how the database processes requests. This can serve as a foundation for further optimization.
Remember, optimization is not a one-time task; it requires ongoing attention as data evolves and grows.
Maintaining Data Integrity
Data integrity is a cornerstone of any database, NoSQL systems included. It is essential to have mechanisms that ensure data remains accurate and reliable throughout its lifecycle. Without proper measures in place, issues like data duplication, inconsistent states, or even total data loss can occur. Here are key practices to maintain integrity within NoSQL databases:
- Use transactions when supported. Not all NoSQL databases offer full ACID properties, but using transactions where available can prevent partial updates that corrupt data states.
- Implement validation rules to ensure data conforms to expected formats and types. This could range from simple type checks to more complex schema validations.
- Regular data audits can help catch issues early. By regularly comparing the actual state of the data against expected conditions, you can maintain consistency and address potential issues before they snowball.
- Document your database schema thoroughly, even if the schema is flexible. Keeping track of what data types are stored and their relationships adds a layer of robustness to your data management practices.
By adhering to these practices, organizations are not just improving their querying processes, but they are fundamentally strengthening the architecture of their data handling strategies. In essence, balancing efficiency and integrity leads to a healthier NoSQL environment.
Future Trends in NoSQL Querying
As technology continues to evolve at breakneck speed, so too does the landscape of NoSQL databases and their querying capabilities. Embracing future trends in this domain isn’t just a nice-to-have; it’s an absolute necessity for organizations looking to harness the full potential of their data. With the shift to non-relational systems, understanding upcoming innovations is critical. The integration of advanced technologies and methodologies will not only enhance querying efficiency but will also pave the way for sophisticated data handling that meets the growing demands of businesses in the digital age.
Emerging Technologies
When talking about the future of NoSQL querying, it's hard to ignore the impact of emerging technologies. Several tools and platforms have been developed that directly address the shortcomings of traditional querying methods. For instance, the rise of multi-model databases allows users to query multiple types of data models through a single interface. This flexibility leads to greater efficiency in handling diverse datasets. Technologies like Apache Cassandra and MongoDB are continually updating their features, focusing on cross-platform compatibility and multi-cloud capabilities, making data querying a breeze across various environments.
- Serverless Architectures: Organizations are starting to turn to serverless options for NoSQL databases, such as AWS DynamoDB. Serverless architectures help remove the burden of infrastructure management, allowing developers to focus solely on querying data.
- Real-Time Processing: The need for real-time data insights is pushing NoSQL technologies to evolve rapidly. Systems are being designed to facilitate real-time querying, integrating seamlessly with event-driven architectures to deliver instant results. This is particularly relevant in sectors like finance, where decision-making is time-sensitive.
- Declarative Queries: Another developing trend is the shift towards declarative querying instead of imperative styles. This means that users will define what they want from the dataset without needing to specify how to achieve it. This approach makes it easier for less technical users to engage with databases, democratizing data access.
"The future of NoSQL querying is about simplicity and power, enabling users from various backgrounds to tap into the data they need effortlessly."
Integration with Machine Learning
The integration of machine learning with NoSQL querying forms a potent combination for extracting insights that were once buried deep in large, unstructured datasets. By merging these two fields, organizations can not only analyze data but can predict trends based on complex patterns within the data. This synergy between NoSQL and machine learning significantly enhances the querying process and the value derived from the data.
- Predictive Analytics: As machine learning models learn from data, they improve in accuracy over time, leading to more refined predictions. By incorporating these models into NoSQL querying, organizations can analyze not just what data exists, but what may happen next, making them more proactive rather than reactive in their decision-making.
- Natural Language Processing (NLP): NLP is becoming increasingly significant. With the combination of NoSQL databases and NLP, querying becomes as simple as asking a question in everyday language. This can open doors for non-technical stakeholders to interact with complex datasets without needing to understand the underpinnings of the technology.
- Automated Data Management: Machine learning algorithms can help automate the categorization and indexing of vast datasets. This means that the querying process can become faster and more efficient, as the system learns which data is relevant to the queries being executed.
In summary, looking ahead at NoSQL databases means staying attuned to emerging technologies and the intersection with machine learning. As these areas converge, the way we query databases will inevitably shift toward more accessible, efficient, and intelligent systems, thereby providing businesses valuable insights and maintaining their competitive edge in the marketplace.
Culmination
In wrapping up this exploration of NoSQL databases and their querying strategies, it’s essential to emphasize several crirical elements that hold significant relevance. Understanding how to query NoSQL systems effectively is no small feat—however, it offers substantial benefits for those willing to learn.
Firstly, one must recognize the extraordinary versatility that NoSQL databases present. Unlike traditional relational databases, which often fit into rigid schemas, NoSQL databases allow for dynamic structures, accommodating varying data formats with ease. This flexibility can substantially enhance efficiency, particularly in environments where data evolves rapidly. For example, in a social media application where user-generated content is continually changing, a NoSQL database simplifies the storage and querying tasks.
Moreover, understanding the nuances of different NoSQL types can empower professionals to choose the right database for their specific needs. For instance, when handling complex relationships and extensive datasets, graph databases such as Neo4j can shine, whereas document stores like MongoDB excel in managing hierarchical data efficiently. Each type offers unique querying techniques tailored to various use cases, thereby streamlining processes.
Additionally, practitioners must consider the challenges inherent in NoSQL querying, like consistency and availability trade-offs. Lighting a path through these complexities not only reduces frustration but enhances performance, leading to more responsive applications. It’s about striking the right balance of data integrity without sacrificing agility.
Lastly, as technology continues to evolve, staying adaptable is imperative. New tools and integrations, especially with the burgeoning field of machine learning, will enable a fresh wave of capabilities for NoSQL querying. Keeping abreast of these trends ensures one remains on the cutting edge, ready to leverage advances in data handling.